Classemes and Other Classifier-Based Features for Efficient Object Categorization
Pattern Analysis and Machine Intelligence, IEEE Transactions (2014)
摘要
This paper describes compact image descriptors enabling accurate object categorization with linear classification models, which offer the advantage of being efficient to both train and test. The shared property of our descriptors is the use of classifiers to produce the features of each image. Intuitively, these classifiers evaluate the presence of a set of basis classes inside the image. We first propose to train the basis classifiers as recognizers of a hand-selected set of object classes. We then demonstrate that better accuracy can be achieved by learning the basis classes as “abstract categories” collectively optimized as features for linear classification. Finally, we describe several strategies to aggregate the outputs of basis classifiers evaluated on multiple subwindows of the image in order to handle cases when the photo contains multiple objects and large amounts of clutter. We test our descriptors on challenging benchmarks of object categorization and detection, using a simple linear SVM as classifier. Our results are on par with those achieved by the best systems in these fields but are produced at orders of magnitude lower computational costs and using an image representation that is general and not specifically tuned for a predefined set of test classes.
更多查看译文
关键词
image classification,image representation,object recognition,support vector machines,abstract category,classemes,classifier-based feature,compact image descriptor,image representation,linear SVM,linear classification model,object categorization,Object categorization,attributes,image features
AI 理解论文
溯源树
样例
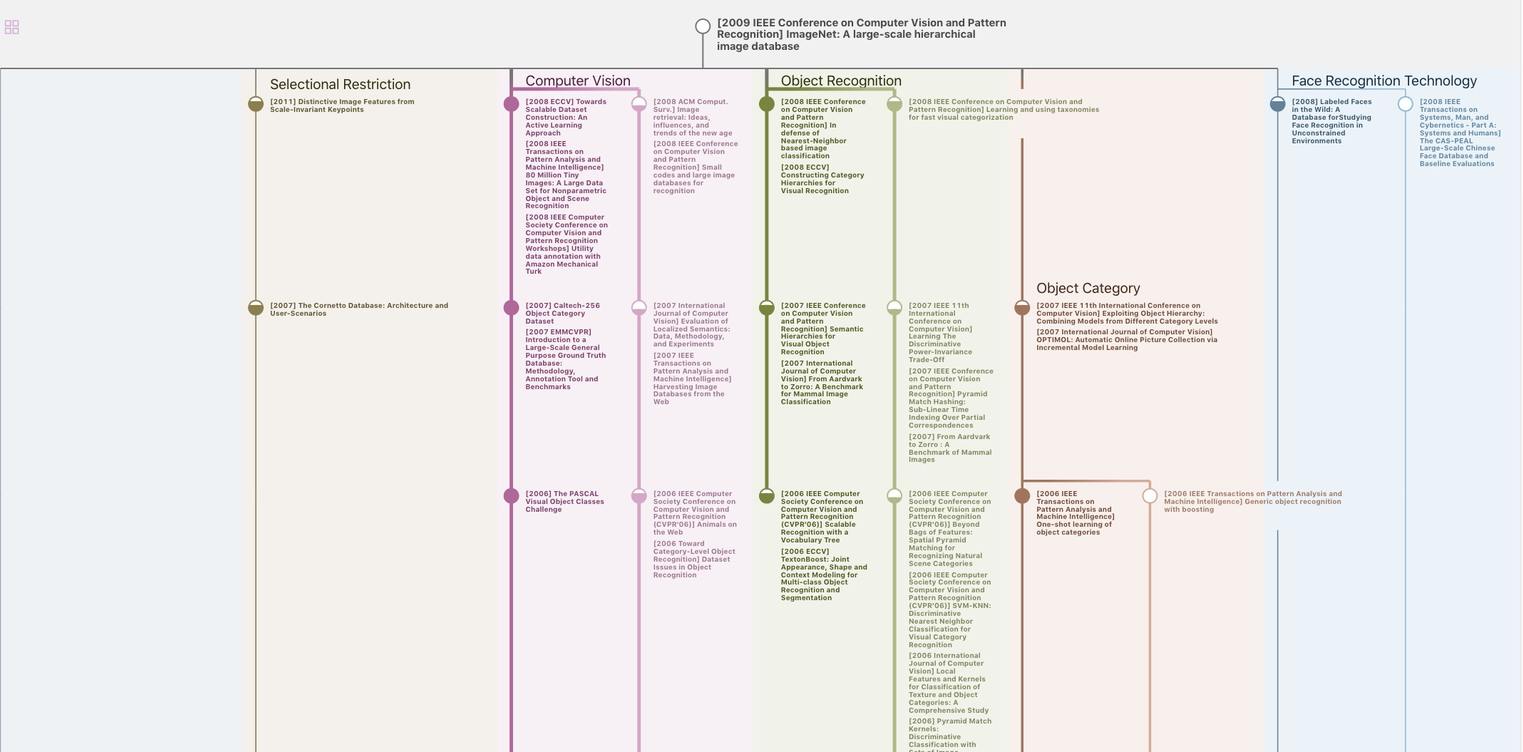
生成溯源树,研究论文发展脉络
Chat Paper
正在生成论文摘要