Sharp Inequalities for -Divergences
Information Theory, IEEE Transactions (2014)
摘要
$f$-divergences are a general class of divergences between probability measures which include as special cases many commonly used divergences in probability, mathematical statistics, and information theory such as Kullback–Leibler divergence, chi-squared divergence, squared Hellinger distance, total variation distance, and so on. In this paper, we study the problem of maximizing or minimizing an $f$-divergence between two probability measures subject to a finite number of constraints on other $f$-divergences. We show that these infinite-dimensional optimization problems can all be reduced to optimization problems over small finite dimensional spaces which are tractable. Our results lead to a comprehensive and unified treatment of the problem of obtaining sharp inequalities between $f$-divergences. We demonstrate that many of the existing results on inequalities between $f$-divergences can be obtained as special cases of our results. We also improve on some existing non-sharp inequalities.
更多查看译文
关键词
algebra,probability,Kullback-Leibler divergence,chi-squared divergence,f-Divergences,infinite dimensional optimization problems,information theory,mathematical statistics,probability measurement,sharp inequalities,squared Hellinger distance,total variation distance,Choquet's theorem,Le Cam's inequality,Pinsker's inequality,convex optimization,hypothesis testing,joint range,probability divergences
AI 理解论文
溯源树
样例
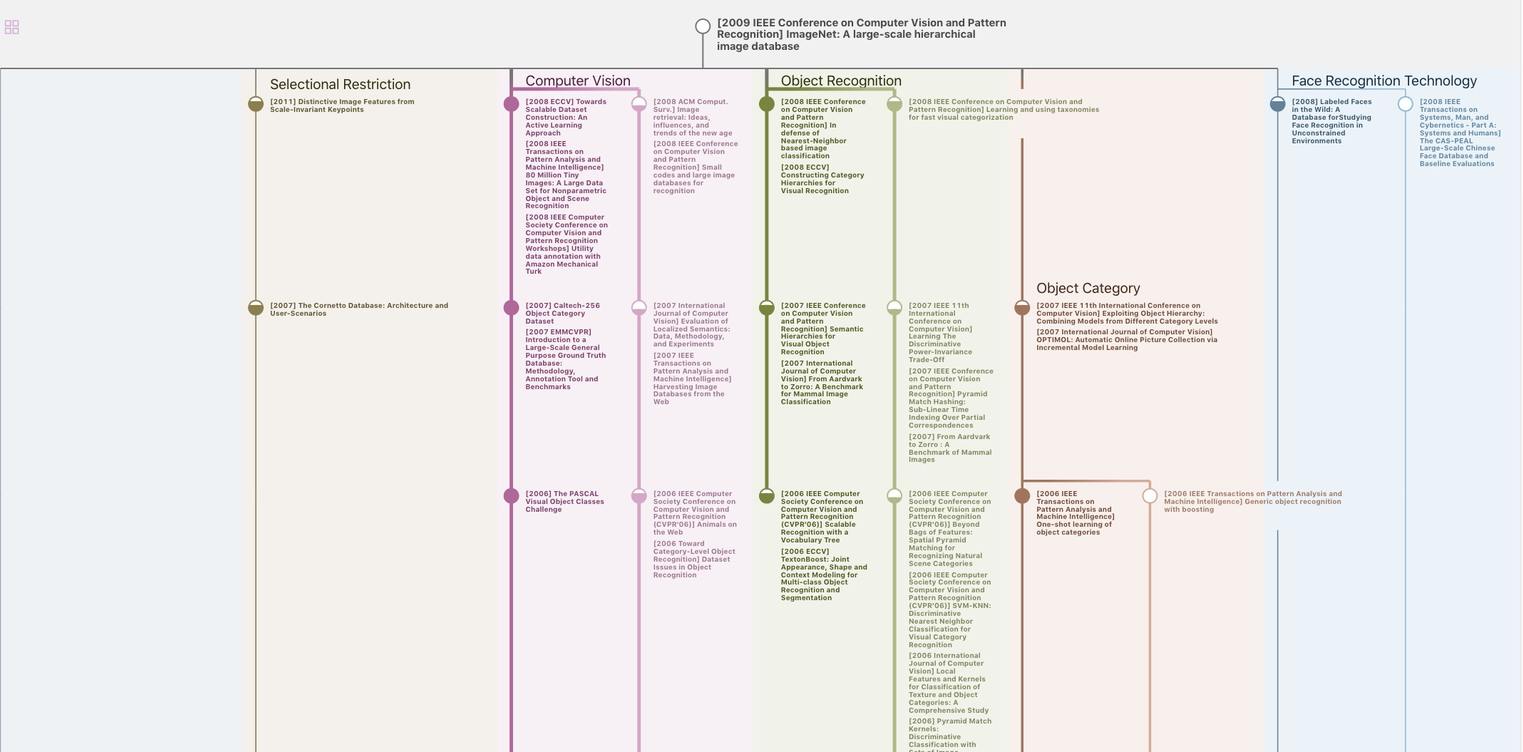
生成溯源树,研究论文发展脉络
Chat Paper
正在生成论文摘要