An instance selection and optimization method for multiple instance learning
SPAC(2014)
摘要
Multiple Instance Learning (MIL) has been an interesting topic in the machine learning community. Since proposed, it has been widely used in content-based image retrieval and classification. In the MIL setting, the samples are bags, which are made of instances. In positive bags, at least one instance is positive. Whereas negative bags have all negative instances. This makes it different from the supervised learning. In this paper, we propose an instance selection and optimization method by selecting the most/least positive/negative instances to form a new training set, and learning the optimal distance metric between instances. We evaluate the proposed method on two benchmark datasets, by comparing with representative MIL algorithms. The experimental results suggest the effectiveness of our algorithm.
更多查看译文
关键词
learning (artificial intelligence),optimisation,MIL,content-based image retrieval,image classification,instance selection,machine learning,multiple instance learning,negative bags,optimal distance metric,optimization method,positive bags,supervised learning
AI 理解论文
溯源树
样例
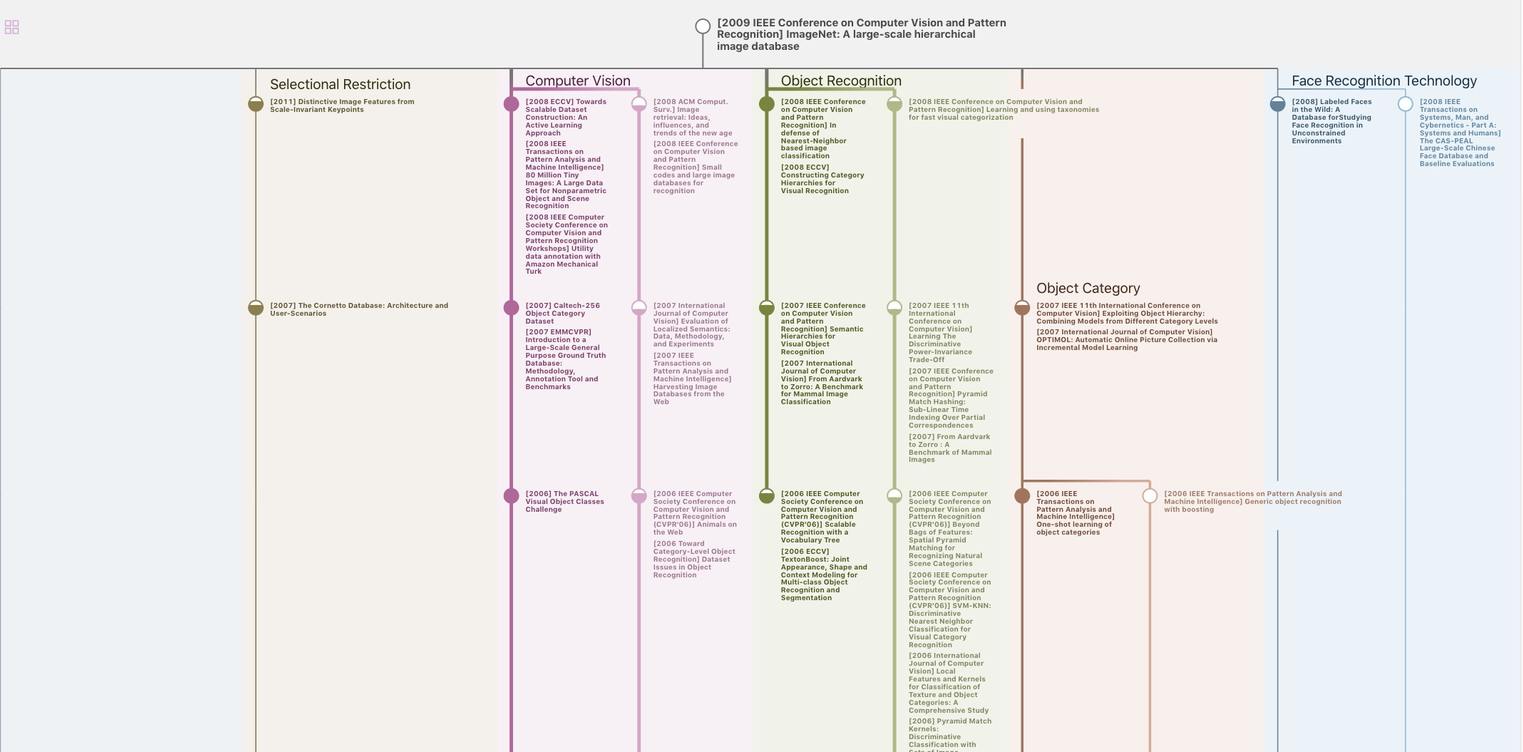
生成溯源树,研究论文发展脉络
Chat Paper
正在生成论文摘要