Online Discovery Of Auv Control Policies To Overcome Thruster Failures
Robotics and Automation(2014)
摘要
We investigate methods to improve fault-tolerance of Autonomous Underwater Vehicles (AUVs) to increase their reliability and persistent autonomy. We propose a learning-based approach that is able to discover new control policies to overcome thruster failures as they happen. The proposed approach is a model-based direct policy search that learns on an on-board simulated model of the AUV. The model is adapted to a new condition when a fault is detected and isolated. Since the approach generates an optimal trajectory, the learned fault-tolerant policy is able to navigate the AUV towards a specified target with minimum cost. Finally, the learned policy is executed on the real robot in a closed-loop using the state feedback of the AUV. Unlike most existing methods which rely on the redundancy of thrusters, our approach is also applicable when the AUV becomes under-actuated in the presence of a fault. To validate the feasibility and efficiency of the presented approach, we evaluate it with three learning algorithms and three policy representations with increasing complexity. The proposed method is tested on a real AUV, Girona500.
更多查看译文
关键词
autonomous underwater vehicles,fault diagnosis,fault tolerance,learning (artificial intelligence),state feedback,AUV control policies,Girona500,autonomous underwater vehicles,closed loop,fault detection,fault tolerance,fault tolerant policy,learning algorithms,model-based direct policy search,on-board simulated model,online discovery,optimal trajectory,persistent autonomy,policy representations,reliability,state feedback,thruster failures
AI 理解论文
溯源树
样例
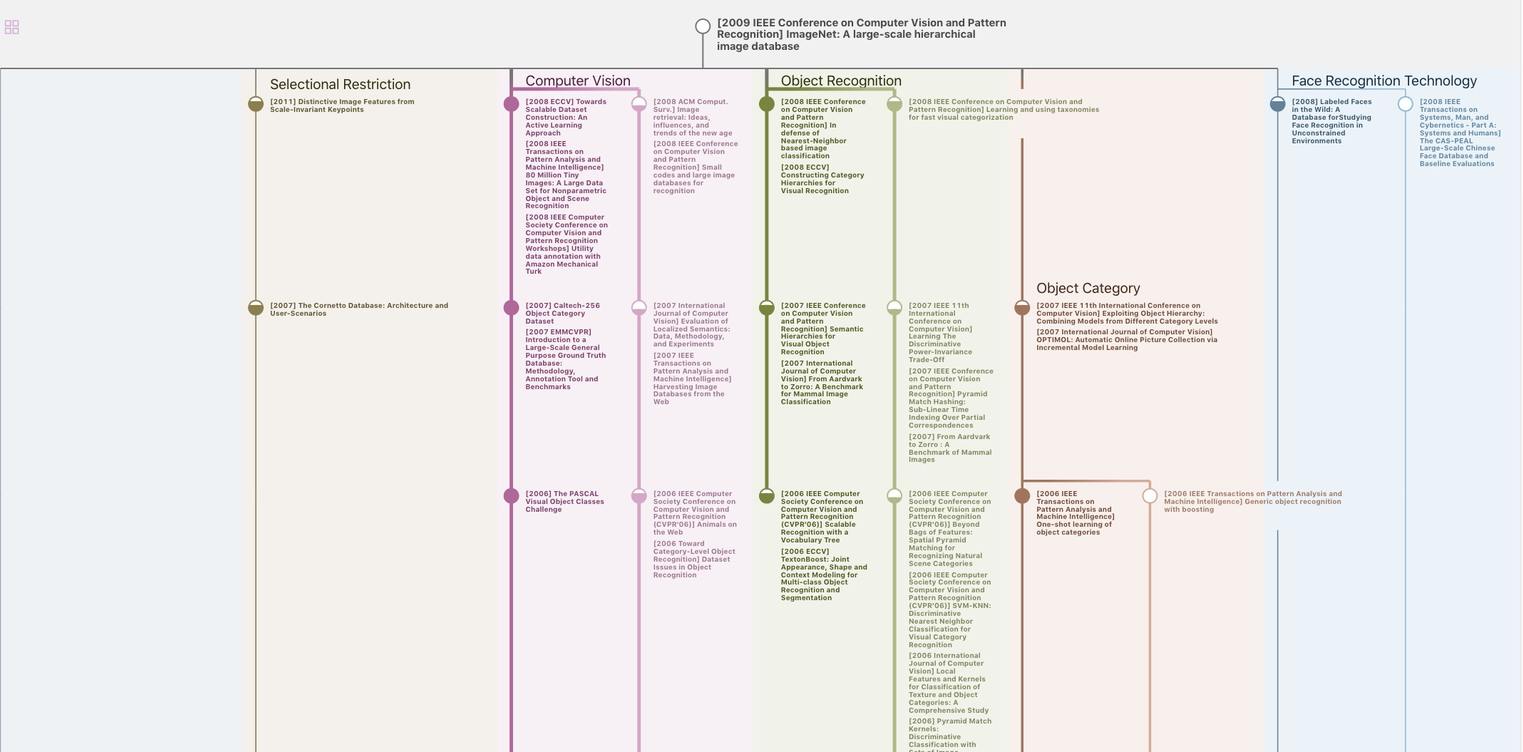
生成溯源树,研究论文发展脉络
Chat Paper
正在生成论文摘要