Large-Scale Multiclass Support Vector Machine Training via Euclidean Projection onto the Simplex
Pattern Recognition(2014)
摘要
Dual decomposition methods are the current state-of-the-art for training multiclass formulations of Support Vector Machines (SVMs). At every iteration, dual decomposition methods update a small subset of dual variables by solving a restricted optimization problem. In this paper, we propose an exact and efficient method for solving the restricted problem. In our method, the restricted problem is reduced to the well-known problem of Euclidean projection onto the positive simplex, which we can solve exactly in expected O(k) time, where k is the number of classes. We demonstrate that our method empirically achieves state-of-the-art convergence on several large-scale high-dimensional datasets.
更多查看译文
关键词
computational complexity,iterative methods,optimisation,support vector machines,Euclidean projection,SVM,dual decomposition methods,large-scale high-dimensional datasets,large-scale multiclass support vector machine training,multiclass formulation training,positive simplex,restricted optimization problem
AI 理解论文
溯源树
样例
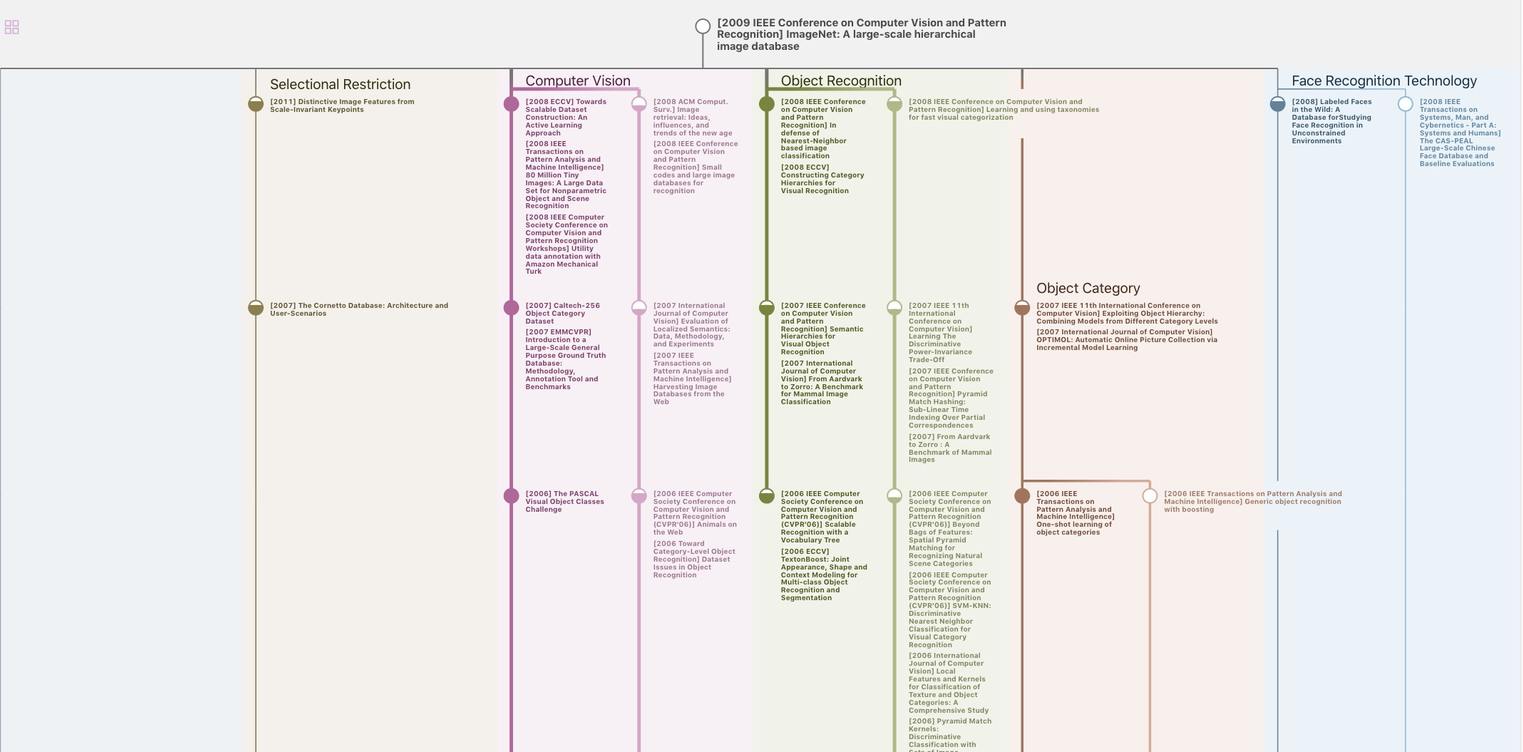
生成溯源树,研究论文发展脉络
Chat Paper
正在生成论文摘要