Adaptive learning of behavioral tasks for patients with Parkinson's disease using signals from deep brain stimulation
Pacific Grove, CA(2014)
摘要
We propose adaptive learning methods for identifying different behavioral tasks of patients with Parkinson's disease (PD). The methods use local field potential (LFP) signals that were collected during Deep Brain Stimulation (DBS) implantation surgeries. Using time-frequency signal processing methods, features are first extracted and then clustered in the feature space using two different methods. The first method requires training and uses a hybrid model that combines support vector machines and hidden Markov models. The second method does not require any a priori information and uses Dirichlet process Gaussian mixture models. Using the DBS acquired signals, we demonstrate the performance of both methods in clustering different behavioral tasks of PD patients and discuss the advantages of each method under different conditions.
更多查看译文
关键词
Gaussian processes,diseases,feature extraction,hidden Markov models,learning (artificial intelligence),medical signal processing,mixture models,prosthetics,support vector machines,time-frequency analysis,DBS implantation surgery,Dirichlet process Gaussian mixture models,LFP signals,PD patients,Parkinson disease,a priori information,behavioral task adaptive learning method,deep brain stimulation,feature extraction,feature space clustering,hidden Markov models,local field potential signals,patient behavioral task identification,support vector machines,time-frequency signal processing methods
AI 理解论文
溯源树
样例
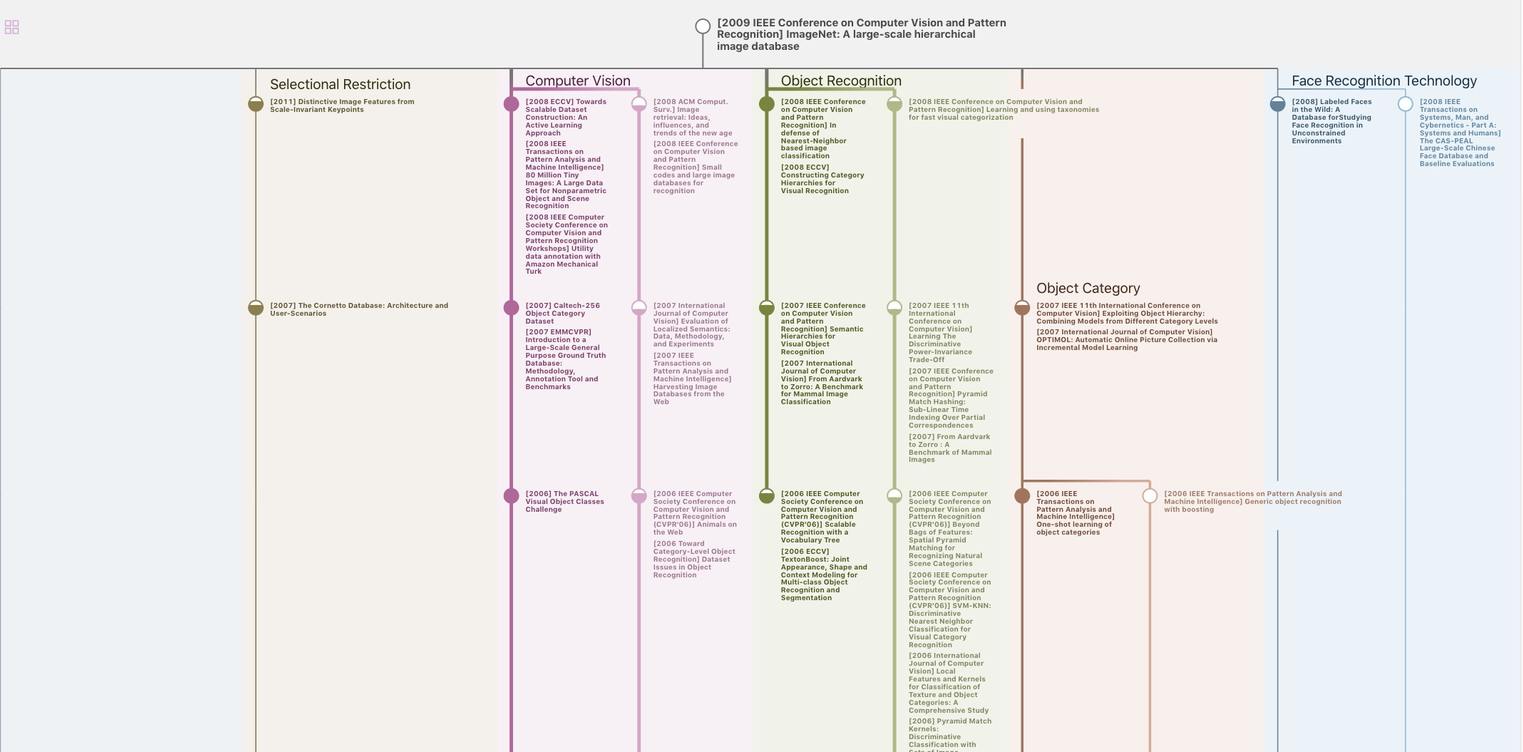
生成溯源树,研究论文发展脉络
Chat Paper
正在生成论文摘要