Dropout Improves Recurrent Neural Networks for Handwriting Recognition
Frontiers in Handwriting Recognition(2014)
摘要
Recurrent neural networks (RNNs) with Long Short-Term memory cells currently hold the best known results in unconstrained handwriting recognition. We show that their performance can be greatly improved using dropout - a recently proposed regularization method for deep architectures. While previous works showed that dropout gave superior performance in the context of convolutional networks, it had never been applied to RNNs. In our approach, dropout is carefully used in the network so that it does not affect the recurrent connections, hence the power of RNNs in modeling sequences is preserved. Extensive experiments on a broad range of handwritten databases confirm the effectiveness of dropout on deep architectures even when the network mainly consists of recurrent and shared connections.
更多查看译文
关键词
handwriting recognition,recurrent neural nets,RNN,convolutional network,dropout,handwritten databases,long short-term memory cells,recurrent connection,recurrent neural network,regularization method,unconstrained handwriting recognition,Dropout,Handwriting Recognition,Recurrent Neural Networks
AI 理解论文
溯源树
样例
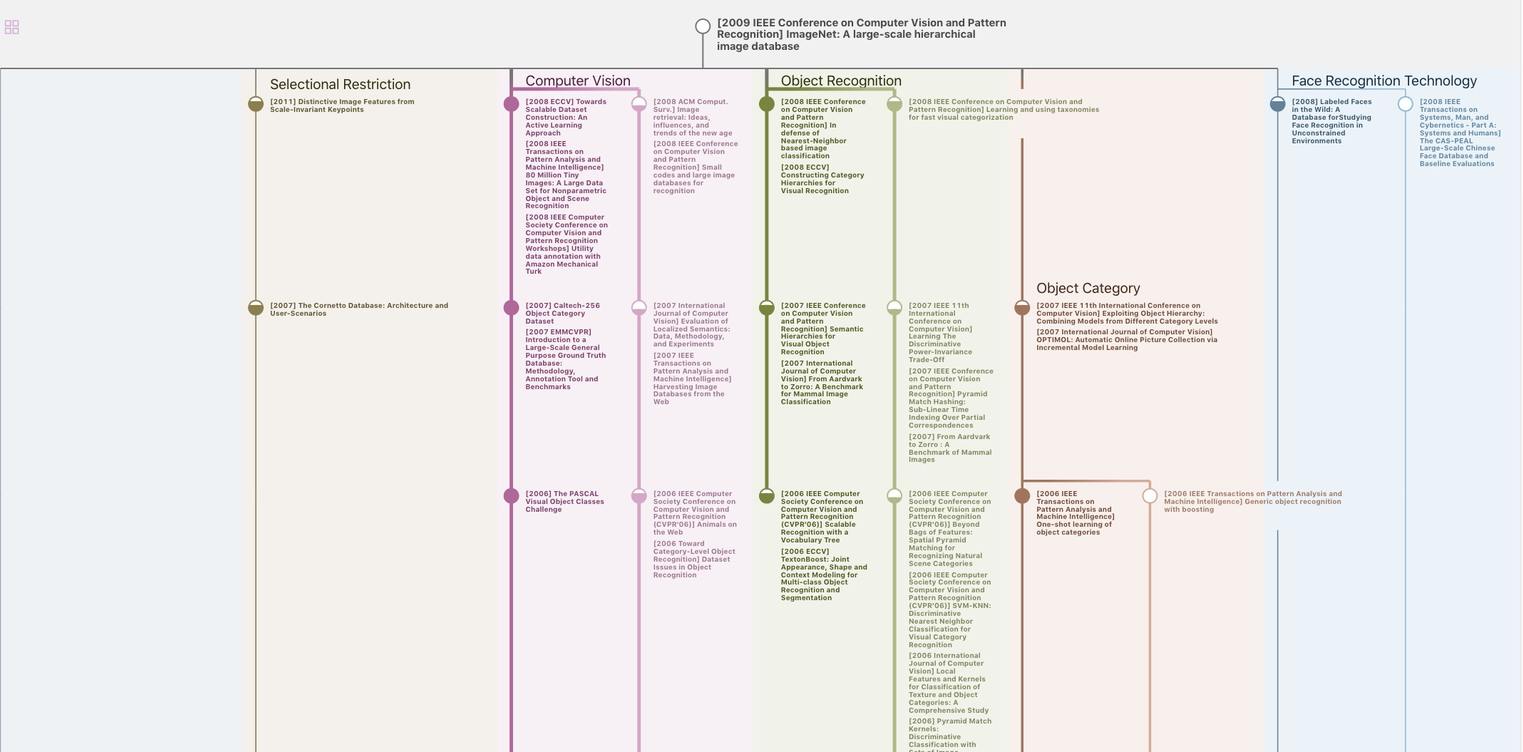
生成溯源树,研究论文发展脉络
Chat Paper
正在生成论文摘要