Learning similarity measures from data with fuzzy sets and particle swarms
CCE(2014)
摘要
Gauging the similarity among objects is a fairly common and important task that underpins many popular machine learning endeavours such as classification or clustering. Uncertainty representation mechanisms, such as rough set theory, or information processing paradigms like granular computing also lean upon well-defined similarity measures to better model the objects in the universe of discourse. In this informationladen world, the responsibility of designing these crucial granular constructs is shifting from domain experts to intelligent systems that automatically learn from data. An approach that hybridizes particle swarm optimization with elements from rough set theory has been recently proposed [1] to build these similarity measures from scratch. However, this scheme still remains fairly sensitive to the values of the similarity thresholds both in the input attribute space and the decision space. In this paper, we tackle this limitation by employing fuzzy sets to categorize the domain of both similarity thresholds. The efficacy of the proposed methodology is illustrated with the K-nearest neighbor classifier. Empirical results over several well-known repositories confirm that this approach preserves the classification accuracy while reducing the number of system parameters and enhancing its interpretability.
更多查看译文
关键词
fuzzy set theory,knowledge representation,learning (artificial intelligence),particle swarm optimisation,pattern classification,rough set theory,classification accuracy,fuzzy sets,granular computing,information processing paradigm,k-nearest neighbor classifier,machine learning,object similarity,particle swarm optimization,similarity measure,similarity measure learning,similarity threshold,uncertainty representation mechanism
AI 理解论文
溯源树
样例
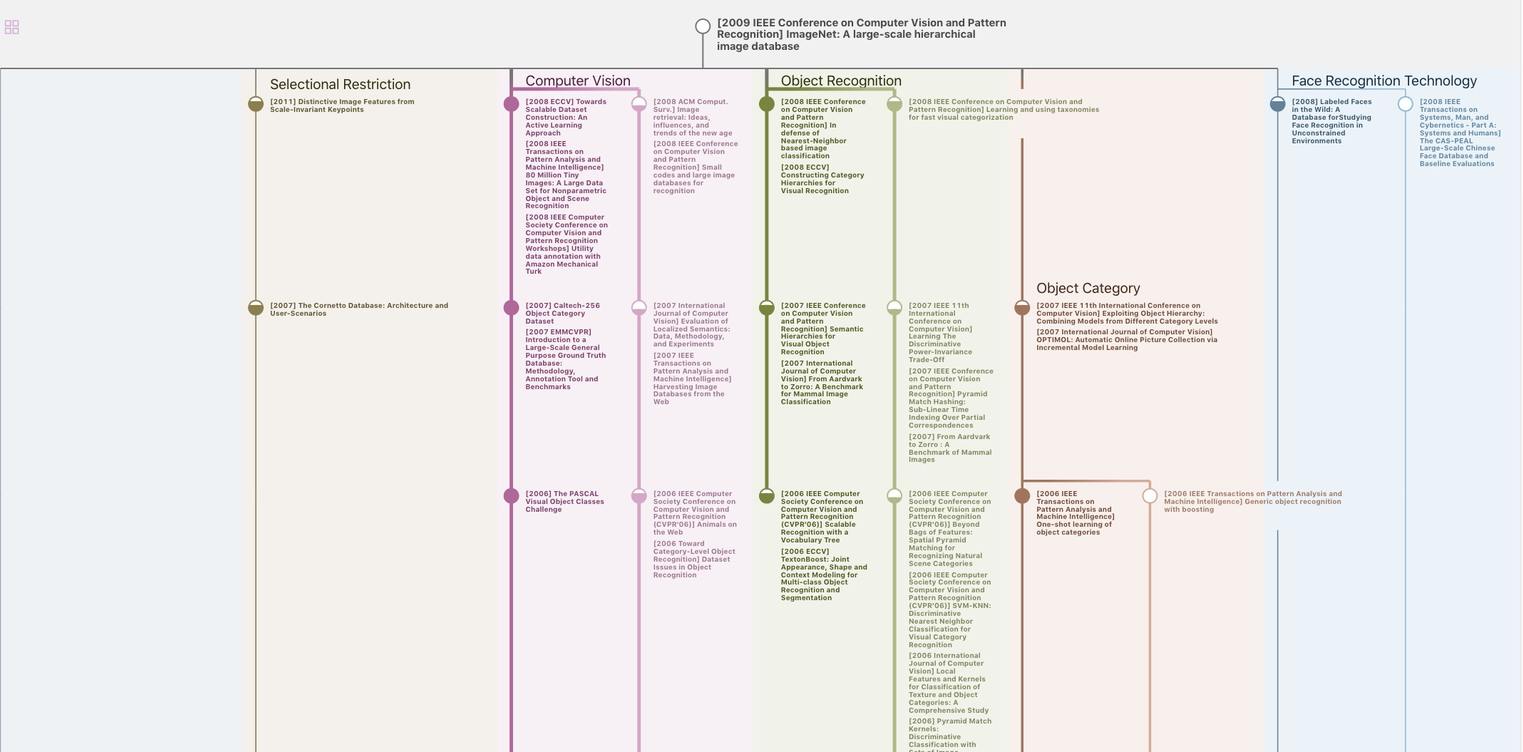
生成溯源树,研究论文发展脉络
Chat Paper
正在生成论文摘要