Optimizing Subspace SVM Ensemble for Hyperspectral Imagery Classification
Selected Topics in Applied Earth Observations and Remote Sensing, IEEE Journal of (2014)
摘要
In hyperspectral remote sensing image classification, ensemble systems with support vector machine (SVM), such as the Random Subspace SVM Ensemble (RSSE), have significantly outperformed single SVM on the robustness and overall accuracy. In this paper, we introduce a novel subspace mechanism, the Optimizing Subspace SVM Ensemble (OSSE), to improve RSSE by selecting discriminating subspaces for individual SVMs. The framework is based on Genetic Algorithm (GA), adopting the Jeffries-Matusita (JM) distance as a criterion, to optimize the selected subspaces. The combination of optimizing subspaces is more suitable for classification than the random one, at the same time having the ability to accommodate requisite diversity within the ensemble. The modifications have improved the accuracies of individual classifiers; as a result, better overall accuracies are present. Experiments on the classification of two hyperspectral datasets reveal that our proposed OSSE obtains sound performances compared with RSSE, single SVM, and other ensemble with GA to optimize SVM.
更多查看译文
关键词
genetic algorithms,geophysical image processing,hyperspectral imaging,image classification,support vector machines,ga,jm distance,jeffries-matusita distance,osse,svm rsse,genetic algorithm,hyperspectral dataset,hyperspectral remote sensing image classification,random subspace support vector machine ensemble optimization,support vector machine,ensemble,hyperspectral imagery classification,optimal subspace,random subspace,support vector machine (svm),optimization,accuracy
AI 理解论文
溯源树
样例
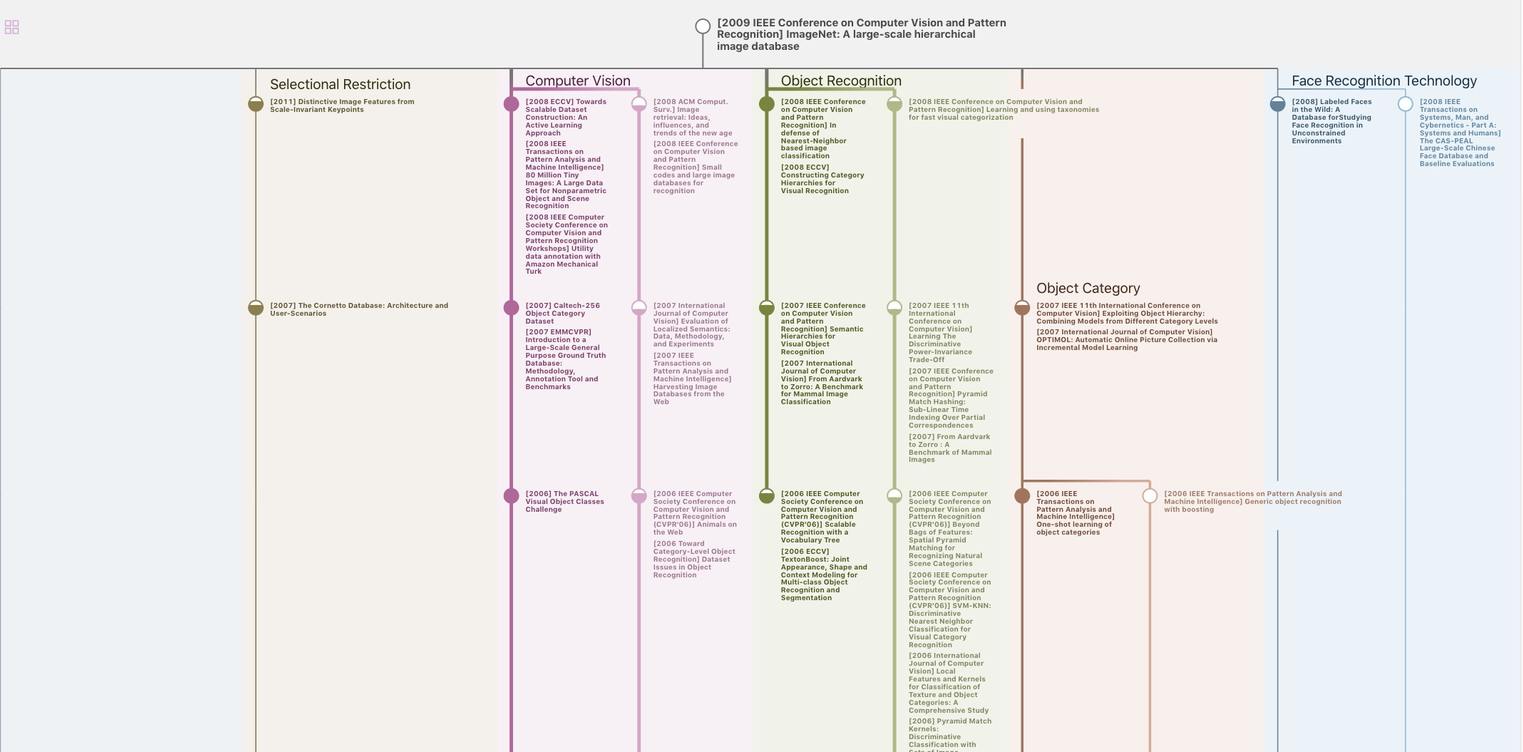
生成溯源树,研究论文发展脉络
Chat Paper
正在生成论文摘要