Decision support for stroke rehabilitation therapy via describable attribute-based decision trees.
EMBC(2014)
摘要
This paper proposes a computational framework for movement quality assessment using a decision tree model that can potentially assist a physical therapist in a telerehabilitation context. Using a dataset of key kinematic attributes collected from eight stroke survivors, we demonstrate that the framework can be reliably used for movement quality assessment of a reach-to-grasp cone task, an activity commonly used in upper extremity stroke rehabilitation therapy. The proposed framework is capable of providing movement quality scores that are highly correlated to the ratings provided by therapists, who used a custom rating rubric created by rehabilitation experts. Our hypothesis is that a decision tree model could be easily utilized by therapists as a potential assistive tool, especially in evaluating movement quality on a large-scale dataset collected during unsupervised rehabilitation (e.g., training at the home), thereby reducing the time and cost of rehabilitation treatment.
更多查看译文
关键词
assistive tool,biomechanics,telemedicine,decision support,computational framework,movement quality scores,unsupervised rehabilitation,reach-to-grasp cone task,physical therapist,rehabilitation treatment,movement quality assessment,patient rehabilitation,decision support systems,upper extremity stroke rehabilitation therapy,custom rating rubric,stroke survivors,kinematics,describable attribute-based decision trees,rehabilitation experts,telerehabilitation context,large-scale dataset,medical computing,decision trees,patient diagnosis,decision tree model,patient treatment
AI 理解论文
溯源树
样例
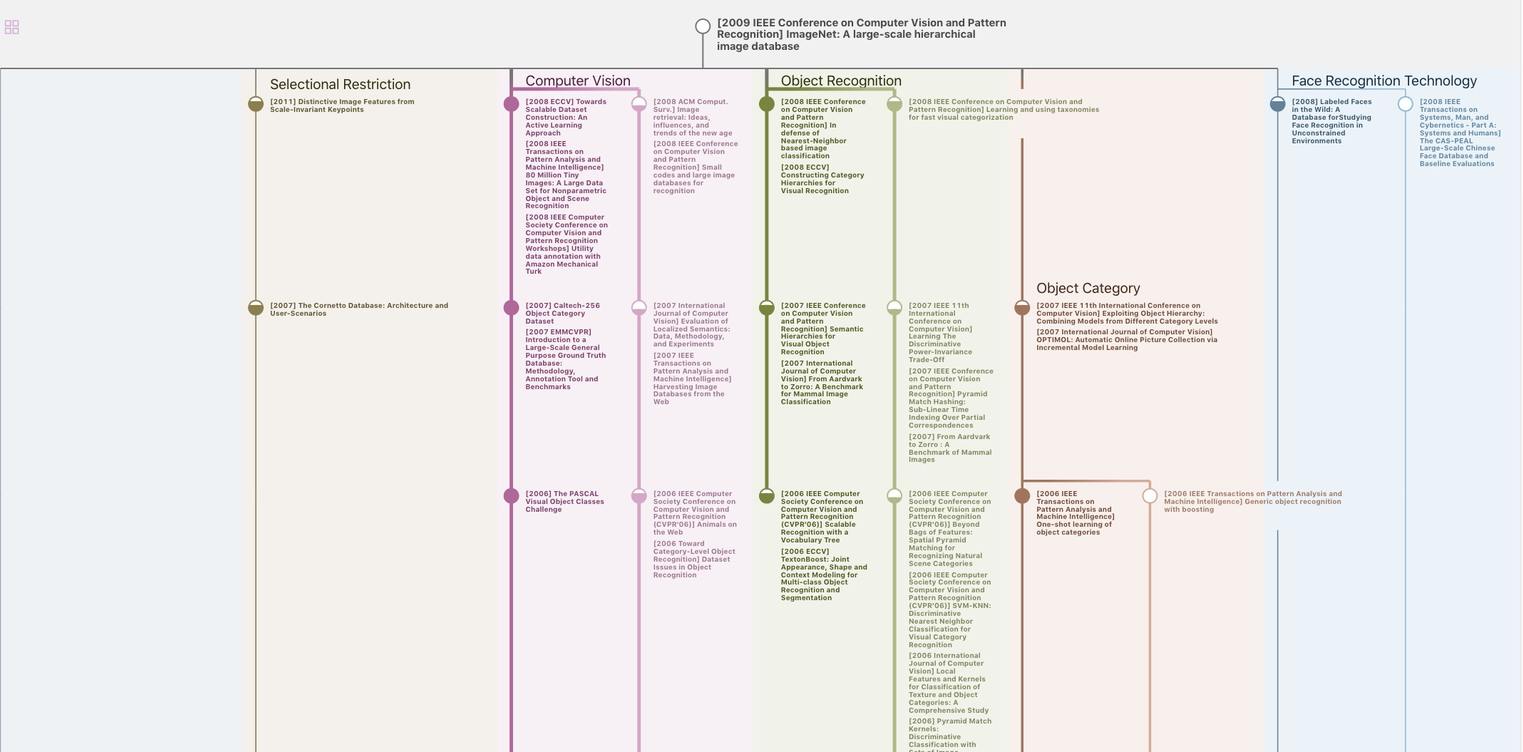
生成溯源树,研究论文发展脉络
Chat Paper
正在生成论文摘要