Analyzing convolutional neural networks for speech activity detection in mismatched acoustic conditions
Acoustics, Speech and Signal Processing(2014)
摘要
Convolutional neural networks (CNN) are extensions to deep neural networks (DNN) which are used as alternate acoustic models with state-of-the-art performances for speech recognition. In this paper, CNNs are used as acoustic models for speech activity detection (SAD) on data collected over noisy radio communication channels. When these SAD models are tested on audio recorded from radio channels not seen during training, there is severe performance degradation. We attribute this degradation to mismatches between the two dimensional filters learnt in the initial CNN layers and the novel channel data. Using a small amount of supervised data from the novel channels, the filters can be adapted to provide significant improvements in SAD performance. In mismatched acoustic conditions, the adapted models provide significant improvements (about 10-25%) relative to conventional DNN-based SAD systems. These results illustrate that CNNs have a considerable advantage in fast adaptation for acoustic modeling in these settings.
更多查看译文
关键词
audio recording,filtering theory,neural nets,speech recognition,telecommunication computing,wireless channels,CNN analysis,DNN,SAD model,audio recording,convolutional neural network analysis,data collection,deep neural network,mismatched acoustic condition,noisy radio communication channel,radio channel,speech activity detection,speech recognition,two dimensional filter,Convolutional neural networks,Neural network adaptation,Speech activity detection
AI 理解论文
溯源树
样例
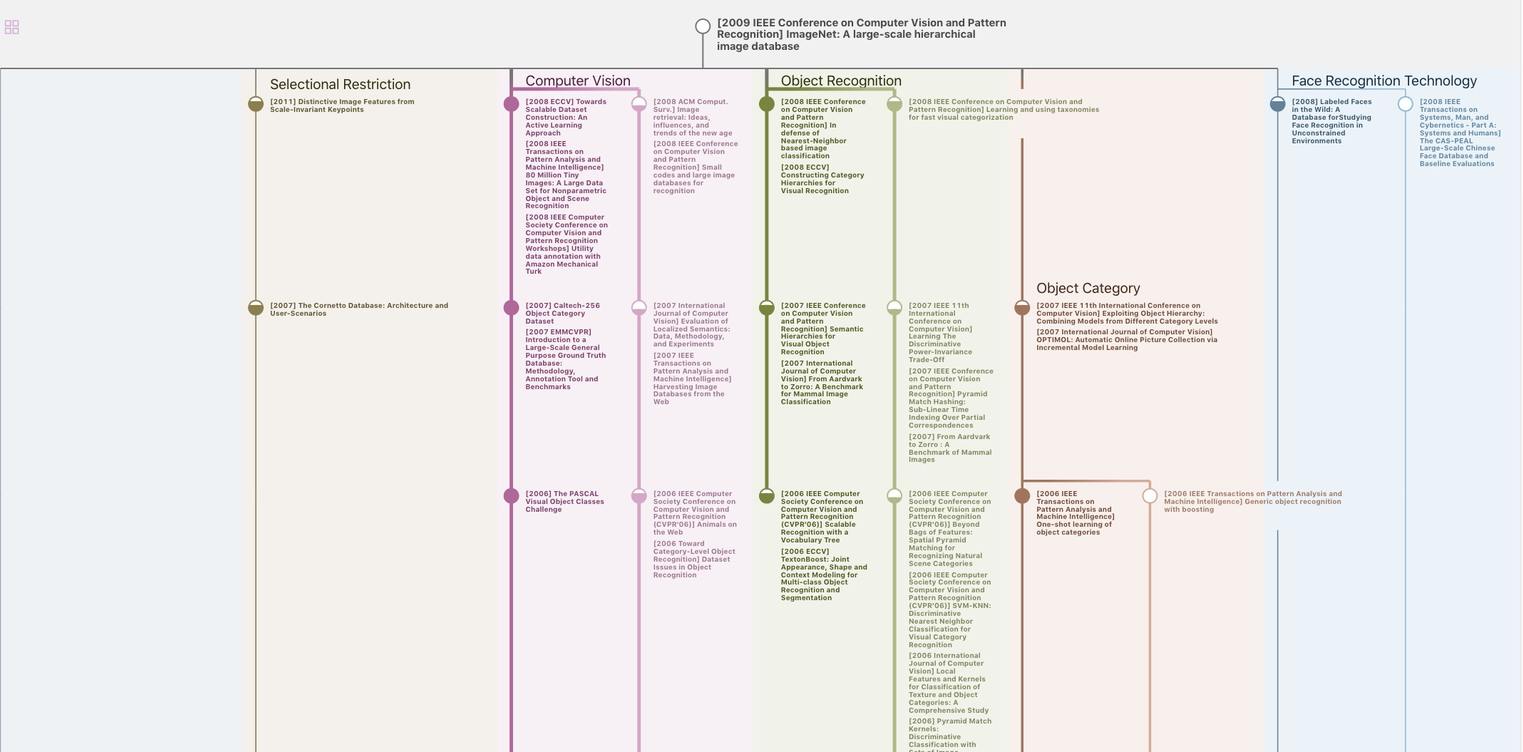
生成溯源树,研究论文发展脉络
Chat Paper
正在生成论文摘要