Calibration of an Integrated Land-Use and Transportation Model Using Maximum-Likelihood Estimation
Computers, IEEE Transactions (2014)
摘要
The focus of this work is calibration of the land-use module of an integrated land-use and transportation model (ILUTM). The calibration task involves estimating key parameters that dictate the output of the land-use module. Hence, an algorithm based on maximum-likelihood estimation (MLE) is developed for calibration. Furthermore, the observed values of the outputs from the land-use module are assumed to admit a Gaussian error. The ILUTM methodology used here is TRANUS which is used to model the city of Grenoble in France. The aforementioned algorithm is then applied to calibrate the land-use module of the Grenoble model. The covariance of the Gaussian error term is assumed to be unknown. It is represented as a function of the land-use module inputs and "hyperparameters.â' The resulting MLE optimization problem has 111 parameters to be estimated, 90 of which are land use parameters and 21 are hyperparameters of the Gaussian covariance kernel. The performance of the proposed calibration methodology is then compared to the traditional calibration techniques used for land use and transportation models, when applied to the Grenoble land-use model. It is observed that the proposed method outperforms the traditional technique when compared based upon a given quantity of interest.
更多查看译文
关键词
Gaussian processes,calibration,land use planning,maximum likelihood estimation,transportation,France,Gaussian covariance kernel,Gaussian error,Grenoble city,Grenoble land-use model,ILUTM methodology,MLE,TRANUS,calibration techniques,hyperparameters,integrated land-use and transportation model calibration,land-use module calibration,maximum-likelihood estimation,Gaussian process modeling,Integrated land use and transportation,calibration and validation under uncertainty,maximum-likelihood estimation,supervised learning
AI 理解论文
溯源树
样例
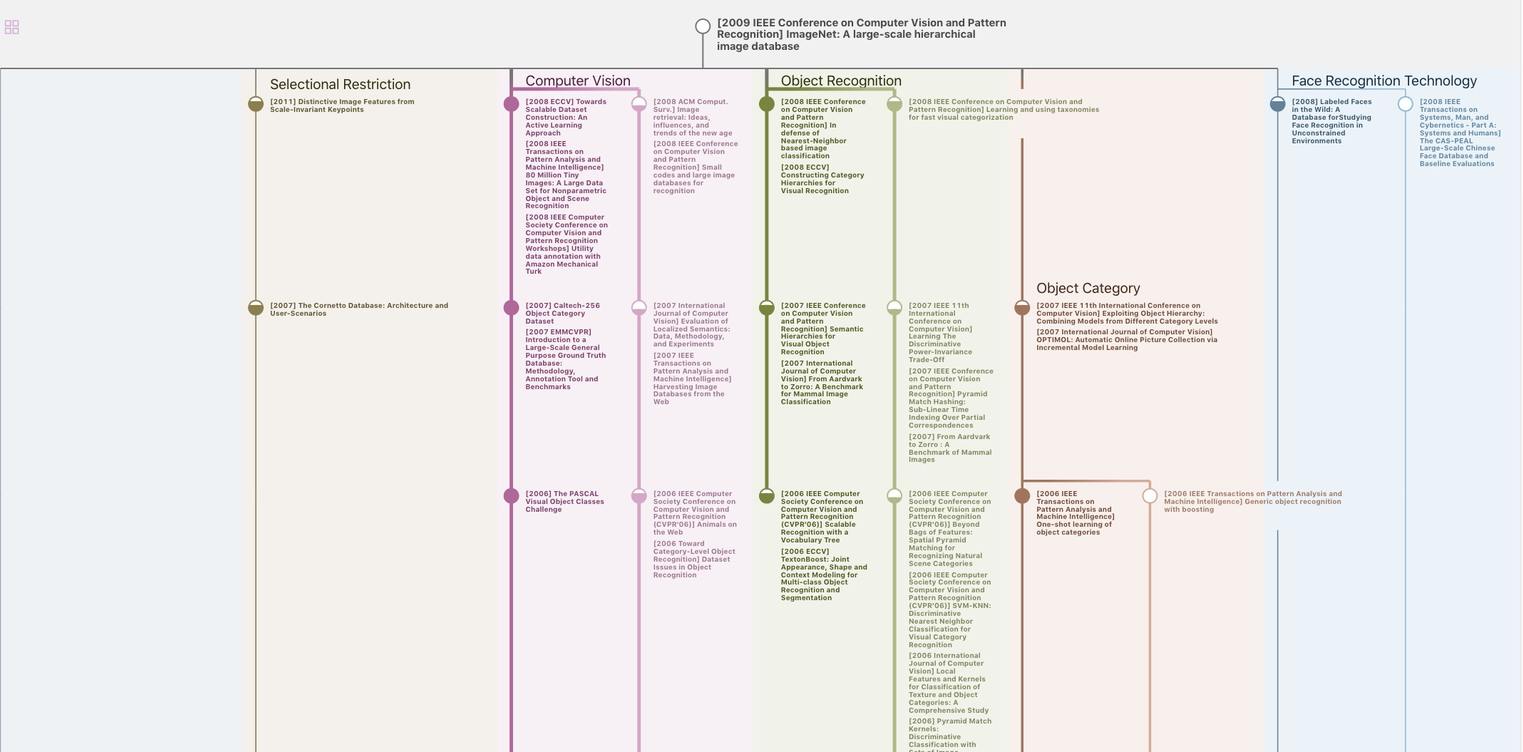
生成溯源树,研究论文发展脉络
Chat Paper
正在生成论文摘要