Swarm/evolutionary intelligence for agent-based social simulation
IEEE Congress on Evolutionary Computation(2014)
摘要
Several micro economic models allow to evaluate consumer's behavior using a utility function that is able to measure the success of an individual's decision. Such a decision may consist of a tuple of goods an individual would like to buy and hours of work necessary to pay for them. The utility of such a decision depends not only on purchase and consumption of goods, but also on fringe benefits such as leisure, which additionally increases the utility to the individual. Utility can be used then as a collective measure for the overall evaluation of societies. In this paper, we present and compare three different agent based social simulations in which the decision finding process of consumers is performed by three algorithms from swarm intelligence and evolutionary computation. Although all algorithms appear to be suitable for the underlying problem as they are based on historical information and also contain a stochastic part which allows for modeling the uncertainty and bounded rationality, they differ greatly in terms of incorporating historical information used for finding new alternative decisions. Newly created decisions that violate underlying budget constraints may either be mapped back to the feasible region, or may be allowed to leave the valid search space. However, in order to avoid biases that would disrupt the inner rationale of each meta heuristic, such invalid decisions are not remembered in the future. Experiments indicate that the choice of such bounding strategy varies according to the choice of the optimization algorithm. Moreover, it seems that each of the techniques could excel in identifying different types of individual behavior such as risk affine, cautious and balanced.
更多查看译文
关键词
consumer behaviour,decision theory,evolutionary computation,macroeconomics,search problems,social sciences,swarm intelligence,agent-based social simulation,bounded rationality,bounding strategy,budget constraints,consumer behavior evaluation,decision finding process,evolutionary computation,historical information,leisure,metaheuristic,microeconomic models,optimization algorithm,risk affine,search space,swarm-evolutionary intelligence,uncertainty modelling,utility function
AI 理解论文
溯源树
样例
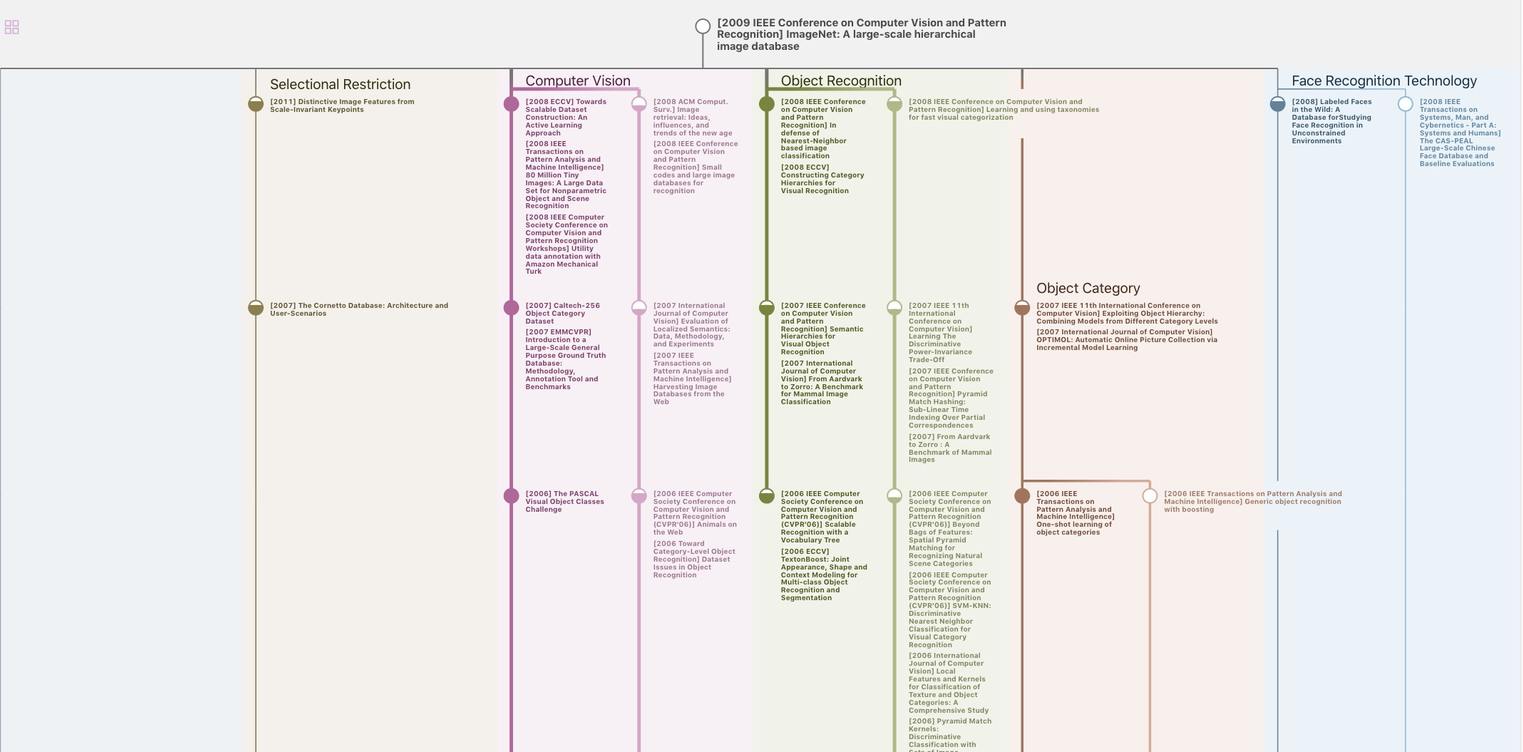
生成溯源树,研究论文发展脉络
Chat Paper
正在生成论文摘要