Multiple-Model Linear Kalman Filter Framework for Unpredictable Signals
Sensors Journal, IEEE (2014)
摘要
This paper presents sensor fusion techniques for systems where the process model is a function of the human input and, therefore, unpredictable. The system consists of free and user-driven motion regimes. The free regime can be modeled as a damped sinusoidal waveform, while the driven regime and the transitions between regimes do not respect any sort of probability, pattern, or sequence. The quantity of interest is the deflection of a clamped beam, measured using three sensor technologies: 1) strain gages; 2) infrared; and 3) Hall effect sensors. Experiments using infrared-based motion capture as reference measuring system show that: 1) none of the sensors present optimal performance for both motion regimes and 2) measurement errors of each sensor differ significantly according to the motion regime. These findings suggest the use of sensor fusion techniques with low processing cost, compatible with real-time embedded applications. Our solution is based on a multiple-model linear Kalman filter in combination with motion segmentation. The motion segmentation discriminates gestures according to the knowledge of their process model. This allows a more predictive estimation during periods of free motion, while relying on a less predictive approach for unknown user-driven signals. In addition, we propose a framework on evaluation and selection of process models for unpredictable signals. The implementation was compared with single-sensor and single-model filter designs. Results based on human subject data reveal that the proposed method improves the error covariance of the estimate by a factor of 2.2 for driven motions and 12.7 for free motions in comparison with single-sensor filter design.
更多查看译文
关键词
hall effect transducers,kalman filters,embedded systems,gesture recognition,image fusion,infrared detectors,motion estimation,hall effect sensors,clamped beam deflection,damped sinusoidal waveform,free motions,infrared sensors,infrared-based motion capture,motion segmentation,multiple-model linear kalman filter framework,process model,real-time embedded applications,reference measuring system,sensor fusion techniques,sensor technologies,single-model filter designs,single-sensor designs,strain gages,unpredictable signals,user-driven motion regimes,user-driven signals,kalman filter evaluation,sensor fusion,classification,user interfaces,hall effect,strain,measurement errors
AI 理解论文
溯源树
样例
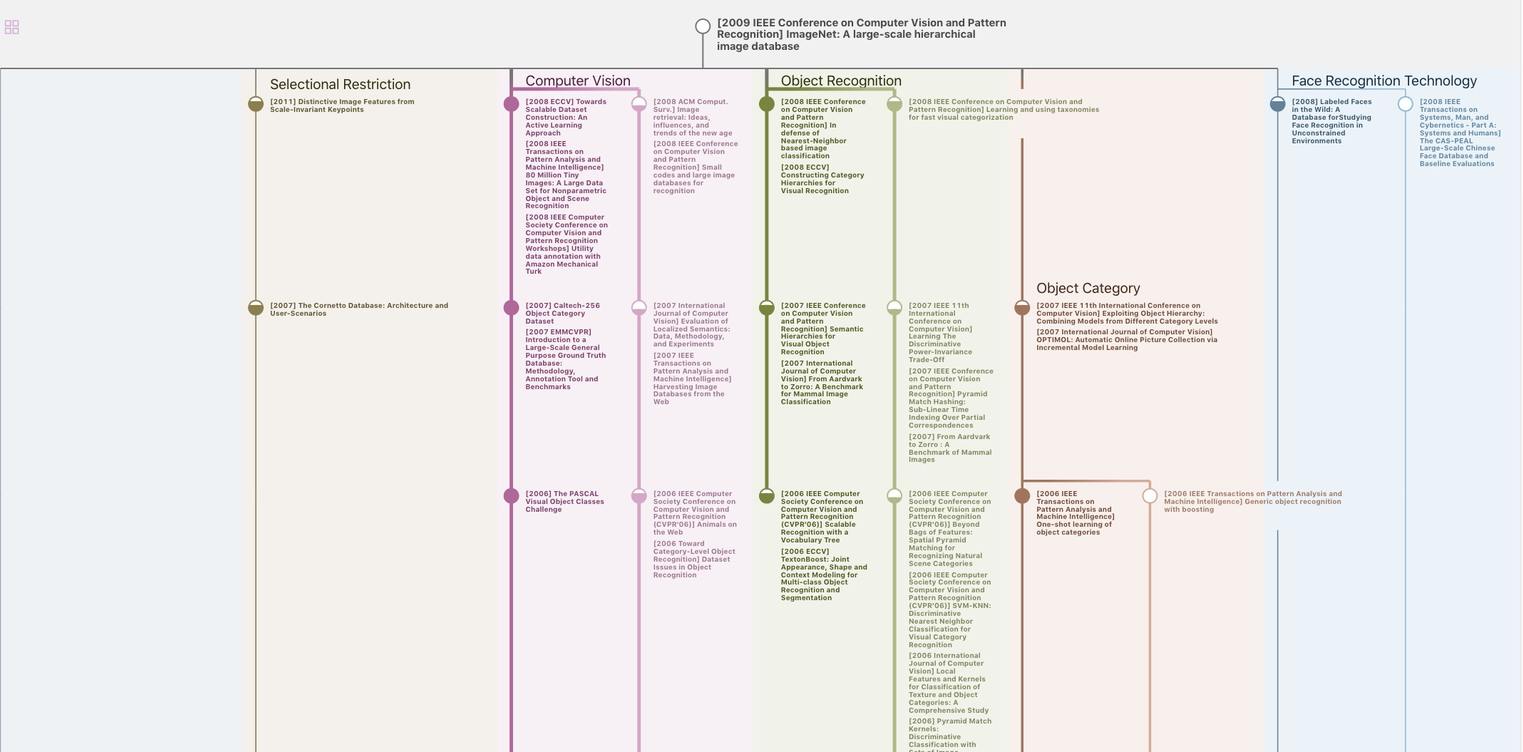
生成溯源树,研究论文发展脉络
Chat Paper
正在生成论文摘要