Integrated microarray analytics for the discovery of gene signatures for triple-negative breast cancer
Computational Intelligence(2014)
摘要
Triple-negative breast cancers (TNBC) are clinically heterogeneous, an aggressive form of breast cancer with poor diagnosis and highly therapeutic resistant. It is urgently needed for identifying novel biomarkers with increased sensitivity and specificity for early detection and personalised therapeutic intervention. Microarray profiling offered significant advances in molecular classification but sample scarcity and cohort heterogeneity remains challenging areas. Here, we investigated diagnostics signatures derived from human triple-negative tissue. We applied REMARK criteria for the selection of relevant studies and compared the signatures gene lists directly as well as assessed their classification performance in predicting diagnosis using leave-one-out cross-validation. The cross-validation results shows excellent classification accuracy ratios using all data sets. A subset signature (17-gene) extracted from the convergence of eligible signatures have also achieved excellent classification accuracy of 89.37% across all data sets. We also applied gene ontology functional enrichment analysis to extract potentially biological process, pathways and network involved in TNBC disease progression. Through functional analysis, we recognized that these independent signatures have displayed commonalities in functional pathways of cell signaling, which play important role in the development and progression of TNBC. We have also identified five unique TNBC pathways genes (SYNCRIP, NFIB, RGS4, UGCG, LOX and NNMT), which could be important for therapeutic interventions as indicated by their close association with known drivers of TNBC and previously published experimental studies.
更多查看译文
关键词
cancer,genetics,lab-on-a-chip,medical computing,patient diagnosis,REMARK criteria,TNBC,biomarkers,gene signatures,integrated microarray analytics,molecular classification,patient diagnosis,therapeutic resistant,triple-negative breast cancer,Bioinformatics,Cell signaling and Therapeutic,Diagnosis,Gene signature,Leave-out-one cross-validation,Triple-negative breast cancer,machine learning,microarray
AI 理解论文
溯源树
样例
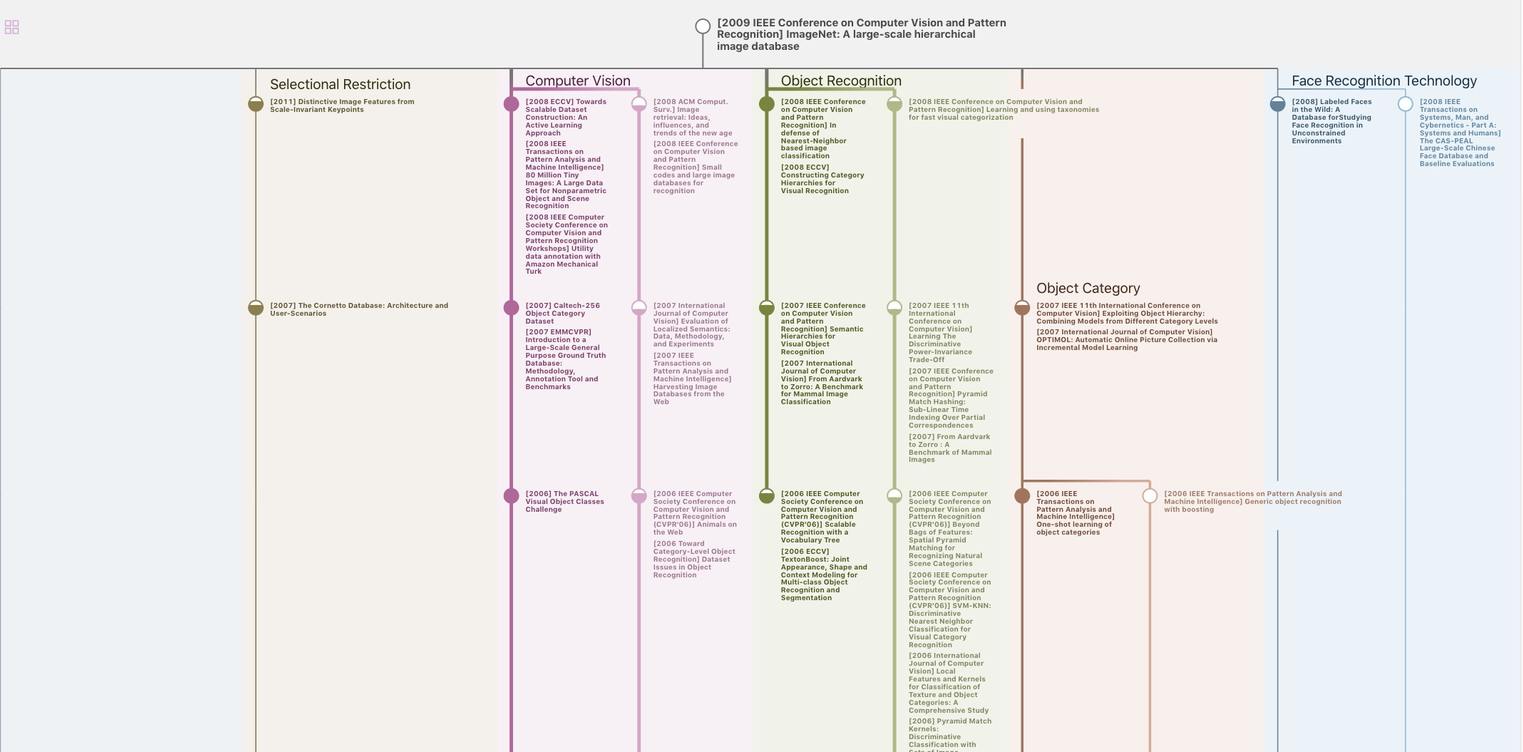
生成溯源树,研究论文发展脉络
Chat Paper
正在生成论文摘要