EEG signal with feature extraction using SVM and ICA classifiers
Information Communication and Embedded Systems(2014)
摘要
Identifying artifacts in EEG data produced by the neurons in brain is an important task in EEG signal processing research. These artifacts are corrected before further analyzing. In this work, fast fixed point algorithm for Independent Component Analysis (ICA) is used for removing artifacts in EEG signals and principal component analysis (PCA) tool is used for reducing high dimensional data and spatial redundancy can be removed. Support vector machine (SVM) tool is used for pattern recognition of EEG signals and the extracted parameters used to implement testing phase of the SVM on the hardware.
更多查看译文
关键词
electroencephalography,independent component analysis,medical signal processing,signal classification,support vector machines,eeg signal processing,ica classifier,svm classifier,fast fixed point algorithm,high dimensional data reduction,pattern recognition,principal component analysis,spatial redundancy,eeg signals,fast ica,pca,svm and hardware architecture,electrodes,vectors,feature extraction
AI 理解论文
溯源树
样例
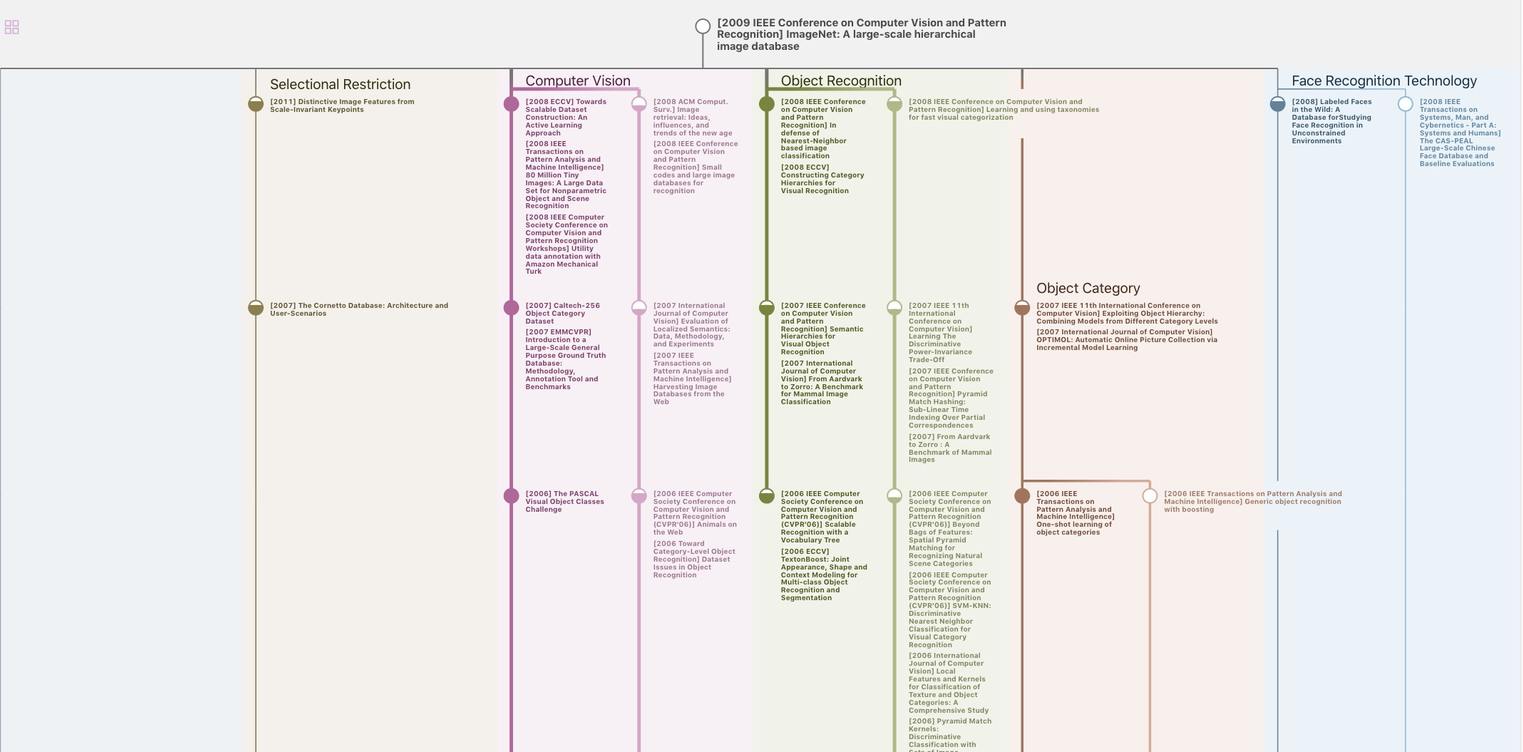
生成溯源树,研究论文发展脉络
Chat Paper
正在生成论文摘要