Bayesian spatiotemporal segmentation of combined PET-CT data using a bivariate poisson mixture model
Signal Processing Conference(2014)
摘要
This paper presents an unsupervised algorithm for the joint segmentation of 4-D PET-CT images. The proposed method is based on a bivariate-Poisson mixture model to represent the bimodal data. A Bayesian framework is developed to label the voxels as well as jointly estimate the parameters of the mixture model. A generalized four-dimensional Potts-Markov Random Field (MRF) has been incorporated into the method to represent the spatio-temporal coherence of the mixture components. The method is successfully applied to 4-D registered PET-CT data of a patient with lung cancer. Results show that the proposed model fits accurately the data and allows the segmentation of different tissues and the identification of tumors in temporal series.
更多查看译文
关键词
Bayes methods,Markov processes,cancer,computerised tomography,image representation,image segmentation,lung,medical image processing,mixture models,positron emission tomography,spatiotemporal phenomena,tumours,4D registered PET-CT data,Bayesian spatiotemporal segmentation,MRF,bimodal data representation,bivariate-Poisson mixture model,combined PET-CT data,generalized four-dimensional Potts-Markov random field,joint 4D PET-CT image segmentation,joint parameter estimation,lung cancer patient,spatio-temporal coherence representation,temporal series,tissue segmentation,tumor identification,unsupervised algorithm,voxel labelling,4-D segmentation,PET-CT,bivariate Poisson distribution,data fusion,multimodality
AI 理解论文
溯源树
样例
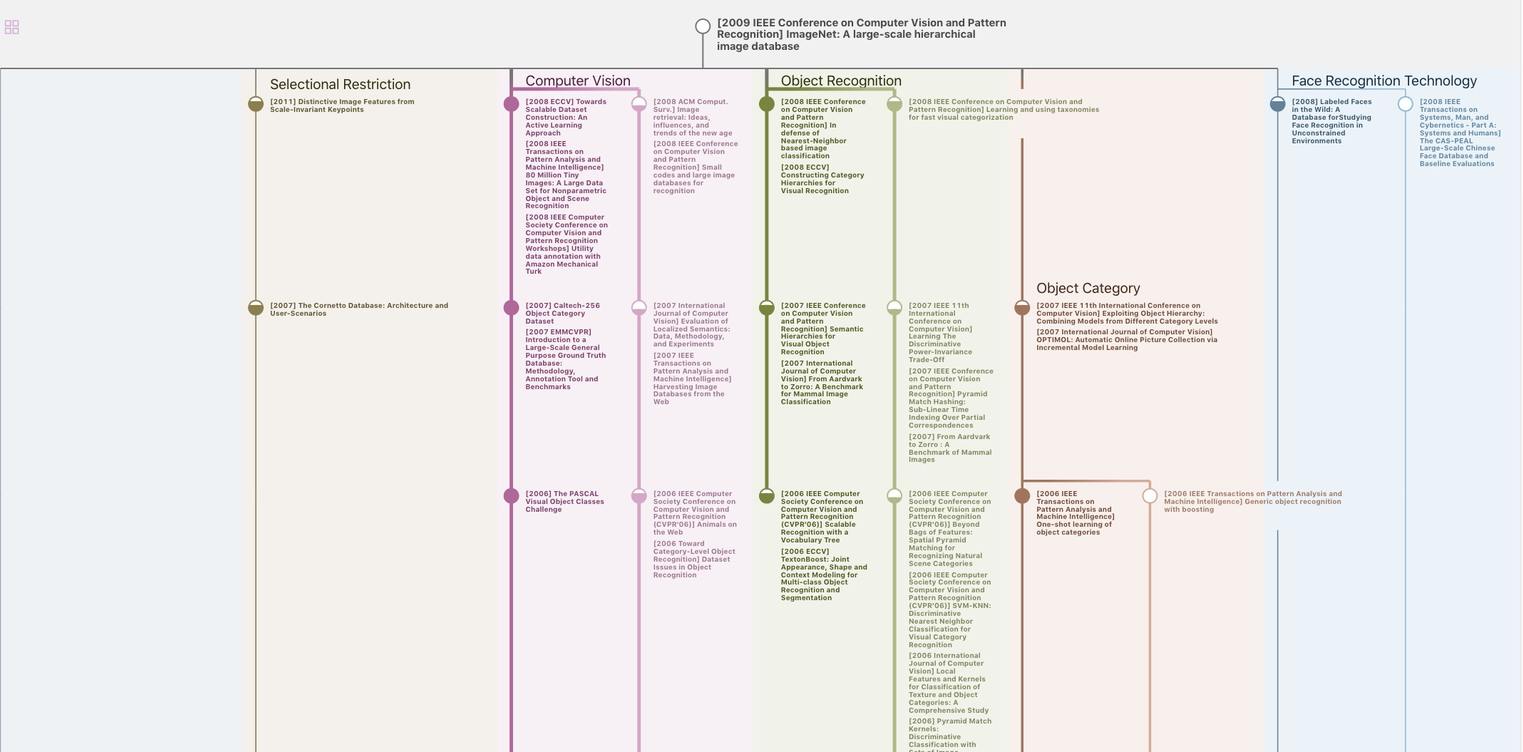
生成溯源树,研究论文发展脉络
Chat Paper
正在生成论文摘要