BayesWipe: A multimodal system for data cleaning and consistent query answering on structured bigdata
BigData Conference(2014)
摘要
Recent efforts in data cleaning of structured data have focused exclusively on problems like data deduplication, record matching, and data standardization; none of these focus on fixing incorrect attribute values in tuples. Correcting values in tuples is typically performed by a minimum cost repair of tuples that violate static constraints like CFDs (which have to be provided by domain experts, or learned from a clean sample of the database). In this paper, we provide a method for correcting individual attribute values in a structured database using a Bayesian generative model and a statistical error model learned from the noisy database directly. We thus avoid the necessity for a domain expert or clean master data. We also show how to efficiently perform consistent query answering using this model over a dirty database, in case write permissions to the database are unavailable. We evaluate our methods over both synthetic and real data.
更多查看译文
关键词
Big Data,learning (artificial intelligence),query processing,BayesWipe system,Bayesian generative model,attribute values correction,data cleaning,data deduplication,data standardization,database,learning,query answering,record matching,statistical error model,structured Big Data,data cleaning,databases,query rewriting,uncertainty,web databases
AI 理解论文
溯源树
样例
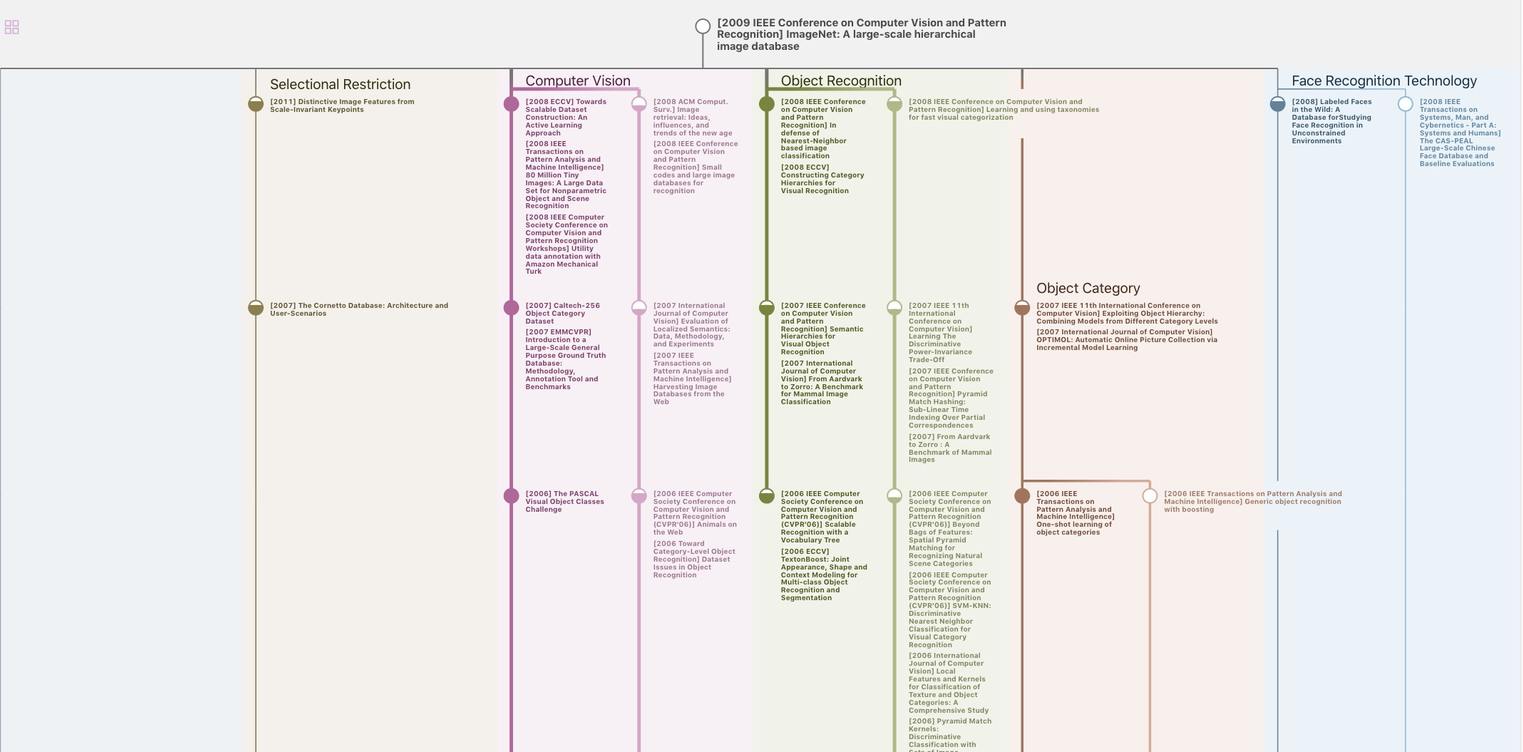
生成溯源树,研究论文发展脉络
Chat Paper
正在生成论文摘要