Prostate boundary segment extraction using cascaded shape regression and optimal surface detection.
EMBC(2014)
摘要
In this paper, we proposed a new method (CSR+OSD) for the extraction of irregular open prostate boundaries in noisy extracorporeal ultrasound image. First, cascaded shape regression (CSR) is used to locate the position of prostate boundary in the images. In CSR, a sequence of random fern predictors are trained in a boosted regression manner, using shape-indexed features to achieve invariance against position variations of prostate boundaries. Afterwards, we adopt optimal surface detection (OSD) to refine the prostate boundary segments across 3D sections globally and efficiently. The proposed method is tested on 162 ECUS images acquired from 8 patients with benign prostate hyperplasia. The method yields a Root Mean Square Distance of 2.11±1.72 mm and a Mean Absolute Distance of 1.61±1.26 mm, which are lower than those of JFilament, an open active contour algorithm and Chan-Vese region based level set model, respectively.
更多查看译文
关键词
optimal surface detection,random processes,noisy extracorporeal ultrasound image,prostate boundary segment extraction,regression analysis,root mean square distance,image segmentation,numerical analysis,biomedical ultrasonics,shape-indexed features,random fern predictor sequence,open active contour algorithm,feature extraction,edge detection,image sequences,chan-vese region based level set model,biological organs,benign prostate hyperplasia,ecus images acquisition,mean absolute distance,cascaded shape regression,medical image processing,mean square error methods
AI 理解论文
溯源树
样例
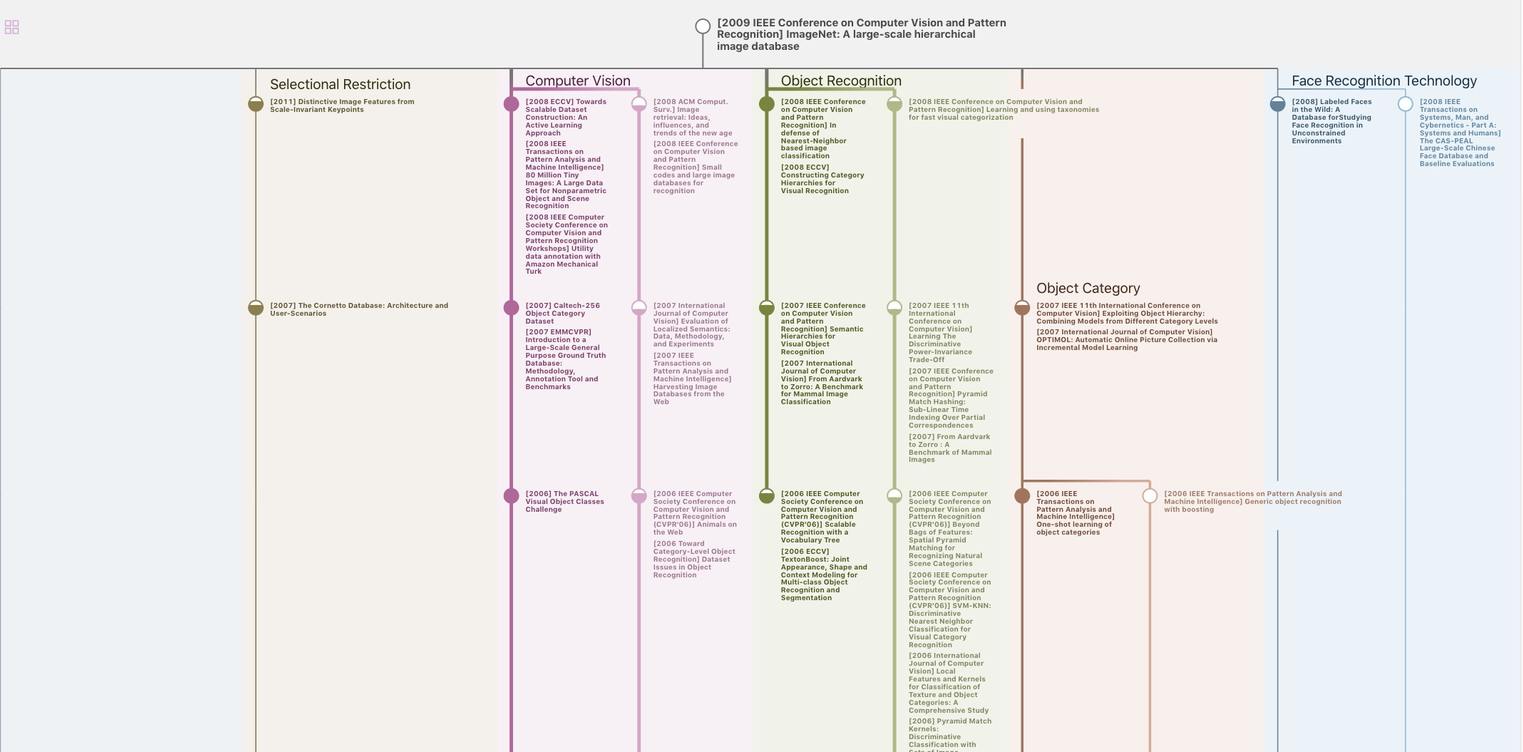
生成溯源树,研究论文发展脉络
Chat Paper
正在生成论文摘要