Stft-Like Time Frequency Representations For Nonstationary Signal - From Evenly Sampled Data To Arbitrary Nonuniformly Sampled Data
SPAC(2014)
摘要
Spectrograms provide an effective way for time-frequency representation (TFR). Among these, short-time Fourier transform (STFT) based spectrograms are extensively used for various applications. However, STFT spectrogram and its revised versions suffer from two main issues: (1) there is a trade-off between time resolution and frequency resolution, and (2) almost all existing TFR methods, including STFT spectrogram, are not suitable to deal with nonuniformly sampled data. In this paper, we address these two problems by presenting alternative approaches, namely short-time amplitude and phase estimation (ST-APES) and short-time sparse learning via iterative minimization (ST-SLIM), to improve the resolution of STFT based spectrogram, and extend the applicability of our approaches to signals with arbitrary sampling patterns. Apart from evenly sampled data, we will consider missing data as well as arbitrary nonuniformly sampled data, at the same time. We will demonstrate via simulation results the superiority of our proposed algorithms in terms of resolution, sidelobe suppression and applicability to signals with arbitrary sampling patterns.
更多查看译文
关键词
time-frequency representation,nonstationary data,nonparametric methods,missing data,nonuniformly sampled data,spectral estimation
AI 理解论文
溯源树
样例
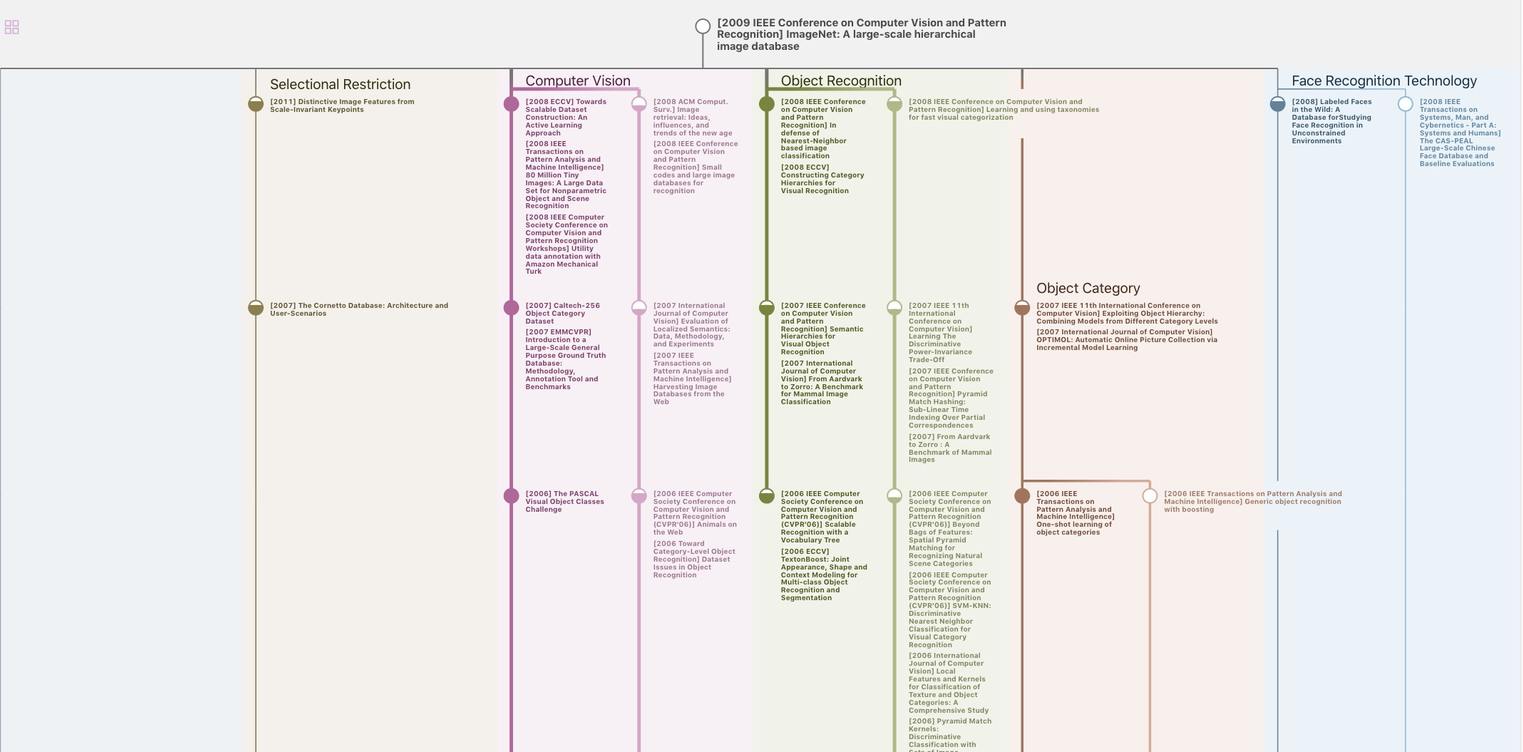
生成溯源树,研究论文发展脉络
Chat Paper
正在生成论文摘要