Beyond Standard Metrics - On the Selection and Combination of Distance Metrics for an Improved Classification of Hyperspectral Data.
ADVANCES IN SELF-ORGANIZING MAPS AND LEARNING VECTOR QUANTIZATION(2014)
摘要
Training and application of prototype based learning approaches such as Learning Vector Quantization, Radial Basis Function networks, and Supervised Neural Gas require the use of distance metrics to measure the similarities between feature vectors as well as class prototypes. While the Euclidean distance is used in many cases, the highly correlated features within the hyperspectral representation and the high dimensionality itself favor the use of more sophisticated distance metrics. In this paper we first investigate the role of different metrics for successful classification of hyperspectral data sets from real-world classification tasks. Second, it is shown that considerable performance gains can be achieved by a classification system that combines a number of prototype based models trained on differently parametrized divergence measures. Data sets are tested using a number of different combination strategies.
更多查看译文
关键词
Divergence,Metrics,Hyperspectral,SNG,GLVQ,RBF
AI 理解论文
溯源树
样例
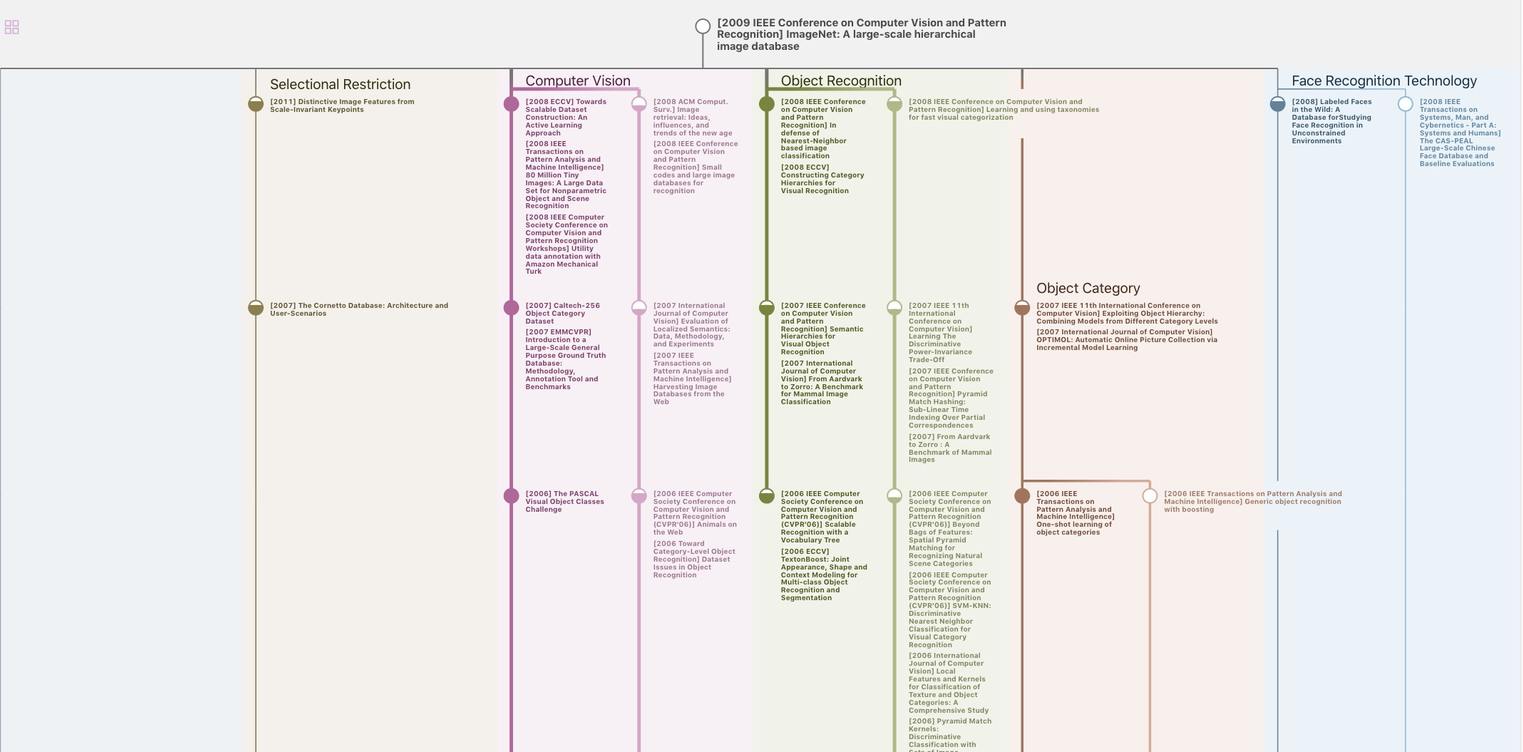
生成溯源树,研究论文发展脉络
Chat Paper
正在生成论文摘要