Fast Information-Theoretic Agglomerative Co-Clustering
DATABASES THEORY AND APPLICATIONS, ADC 2014(2014)
摘要
Jointly clustering the rows and the columns of large matrices, a.k.a. co-clustering, finds numerous applications in the real world such as collaborative filtering, market-basket and micro-array data analysis, graph clustering, etc. In this paper, we formulate an information-theoretic objective cost function to solve this problem, and develop a fast agglomerative algorithm to optimize this objective. Our algorithm rapidly finds highly similar clusters to be merged in an iterative fashion using Locality-Sensitive Hashing. Thanks to its bottom-up nature, it also enables the analysis of the cluster hierarchies. Finally, the number of row and column clusters are automatically determined without requiring the user to choose them. Our experiments on both real and synthetic datasets show that the proposed algorithm achieves high-quality clustering solutions and scales linearly with the input matrix size.
更多查看译文
AI 理解论文
溯源树
样例
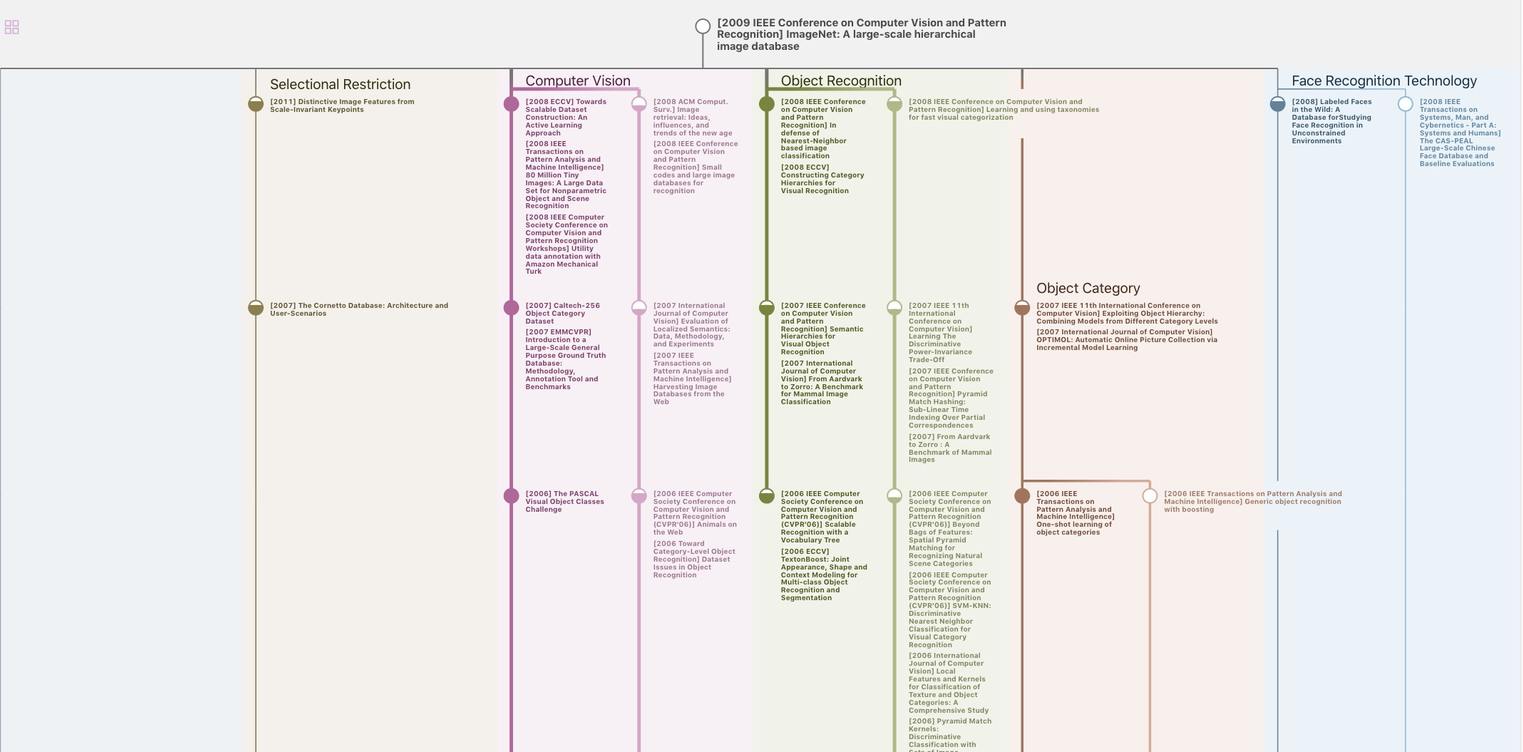
生成溯源树,研究论文发展脉络
Chat Paper
正在生成论文摘要