Target perceivability for multi-frame multi-target tracking
Fusion(2014)
摘要
This paper presents a novel weight assignment model for a rank constrained continuous formulation of multiframe multi-target data association problem. The new weight assignment model explicitly estimates the joint probability of the target perceivability, the target state and measurement, within a Bayesian framework with continuous update when new measurements are available. The knowledge on target perceivability state at every time step also provides a new pruning criterion for reducing the space of all possible track associations. Experiments on synthetic data under different operating conditions demonstrate the effectiveness of the proposed weight assignment model for multi-target tracking problem. It also presents good qualitative tracking performance on real-world video data.
更多查看译文
关键词
weight assignment model,video signal processing,track associations,image fusion,multiframe multitarget data association problem,statistical analysis,target tracking,joint probability estimation,multiframe multitarget tracking,target perceivability,real-world video data,pruning criterion,bayesian framework,computational modeling,hidden markov models,time measurement,data models
AI 理解论文
溯源树
样例
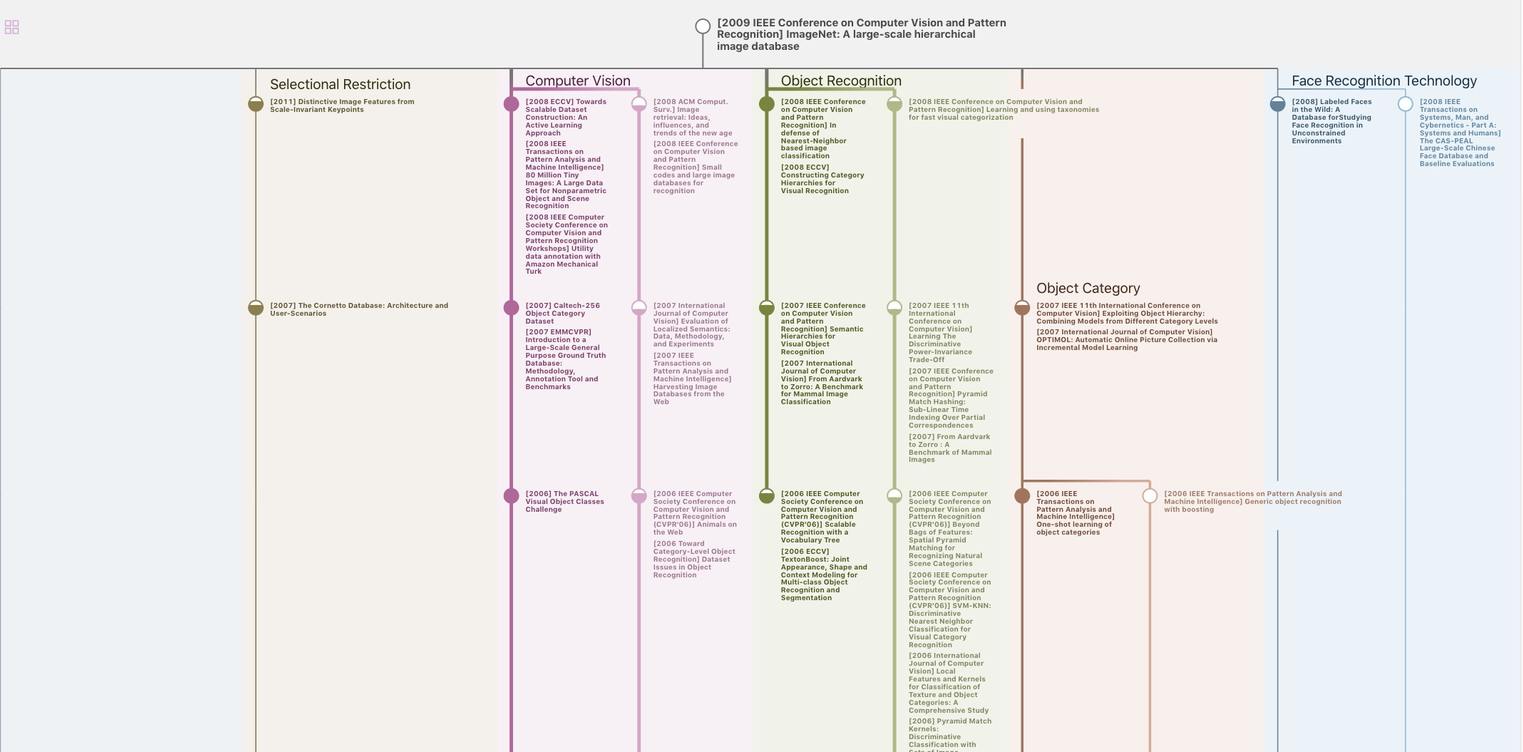
生成溯源树,研究论文发展脉络
Chat Paper
正在生成论文摘要