Improving Performance on Problems with Few Labelled Data by Reusing Stacked Auto-Encoders
ICMLA(2014)
摘要
Deep architectures have been used in transfer learning applications, with the aim of improving the performance of networks designed for a given problem by reusing knowledge from another problem. In this work we addressed the transfer of knowledge between deep networks used as classifiers of digit and shape images, considering cases where only the set of class labels, or only the data distribution, changed from source to target problem. Our main goal was to study how the performance of knowledge transfer between such problems would be affected by varying the number of layers being retrained and the amount of data used in that retraining. Generally, reusing networks trained for a different label set led to better results than reusing networks trained for a different data distribution. In particular, reusing for less classes a network trained for more classes was beneficial for virtually any amount of training data. In all cases, retraining only one layer to save time consistently led to poorer performance. The results obtained when retraining for upright digits a network trained for rotated digits raise the hypothesis that transfer learning could be used to better deal with image classification problems in which only a small amount of labelled data is available for training.
更多查看译文
关键词
shape,training data,knowledge engineering,vectors
AI 理解论文
溯源树
样例
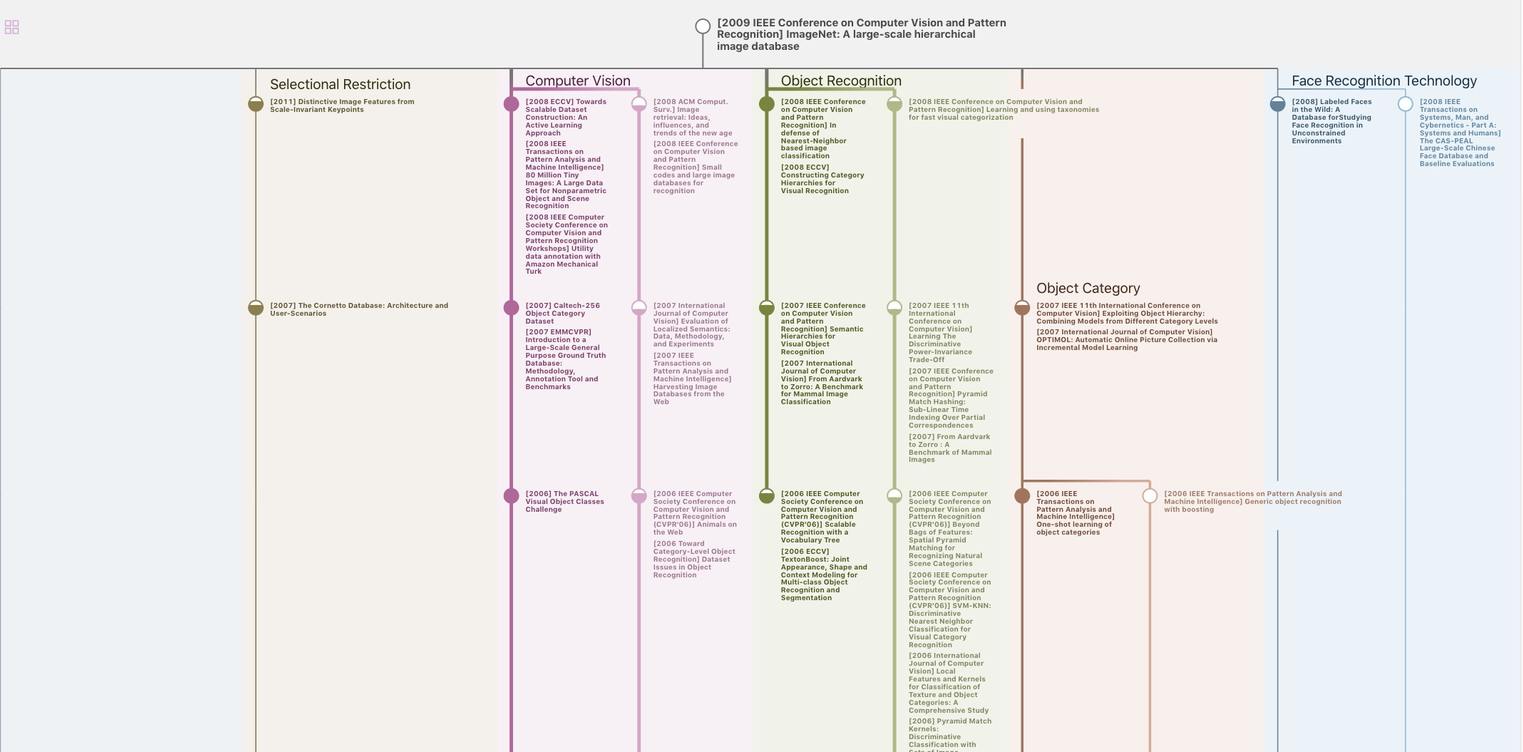
生成溯源树,研究论文发展脉络
Chat Paper
正在生成论文摘要