Backdoors to planning
Artificial Intelligence(2019)
摘要
Backdoors measure the distance to tractable fragments and have become an important tool to find fixed-parameter tractable (fpt) algorithms for hard problems in AI and beyond. Despite their success, backdoors have not been used for planning, a central problem in AI that has a high computational complexity. In this work, we introduce two notions of backdoors building upon the causal graph. We analyze the complexity of finding a small backdoor (detection) and using the backdoor to solve the problem (evaluation) in the light of planning with (un)bounded plan length/domain of the variables. For each setting we present either an fpt-result or rule out the existence thereof by showing parameterized intractability. For several interesting cases we achieve the most desirable outcome: detection and evaluation are fpt. In addition, we explore the power of polynomial preprocessing for all fpt-results, i.e., we investigate whether polynomial kernels exist. We show that for the detection problems, polynomial kernels exist whereas we rule out the existence of polynomial kernels for the evaluation problems.
更多查看译文
关键词
Planning,Backdoors,Causal graph,Fixed-parameter tractable algorithms,(Parameterized) complexity
AI 理解论文
溯源树
样例
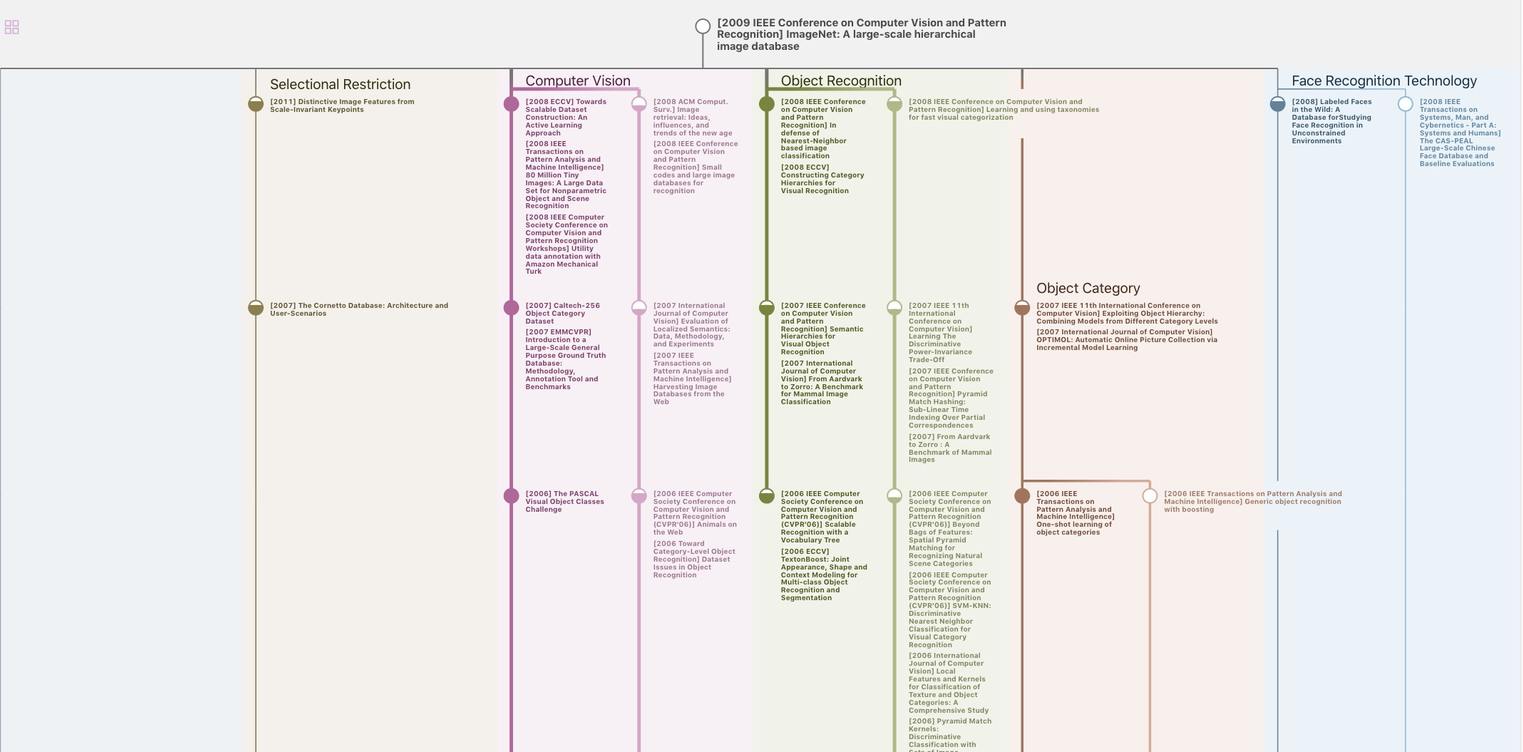
生成溯源树,研究论文发展脉络
Chat Paper
正在生成论文摘要