Sof: Soft-Cluster Matrix Factorization For Probabilistic Clustering
PROCEEDINGS OF THE TWENTY-NINTH AAAI CONFERENCE ON ARTIFICIAL INTELLIGENCE(2015)
摘要
We propose SoF (Soft-cluster matrix Factorization), a probabilistic clustering algorithm which softly assigns each data point into clusters. Unlike model-based clustering algorithms, SoF does not make assumptions about the data density distribution. Instead, we take an axiomatic approach to define 4 properties that the probability of co-clustered pairs of points should satisfy. Based on the properties, SoF utilizes a distance measure between pairs of points to induce the conditional co-cluster probabilities. The objective function in our framework establishes an important connection between probabilistic clustering and constrained symmetric Nonnegative Matrix Factorization (NMF), hence providing a theoretical interpretation for NMF-based clustering algorithms. To optimize the objective, we derive a sequential minimization algorithm using a penalty method. Experimental results on both synthetic and real-world datasets show that SoF significantly outperforms previous NMF-based algorithms and that it is able to detect non-convex patterns as well as cluster boundaries.
更多查看译文
关键词
nonnegative matrix factorization,optimization
AI 理解论文
溯源树
样例
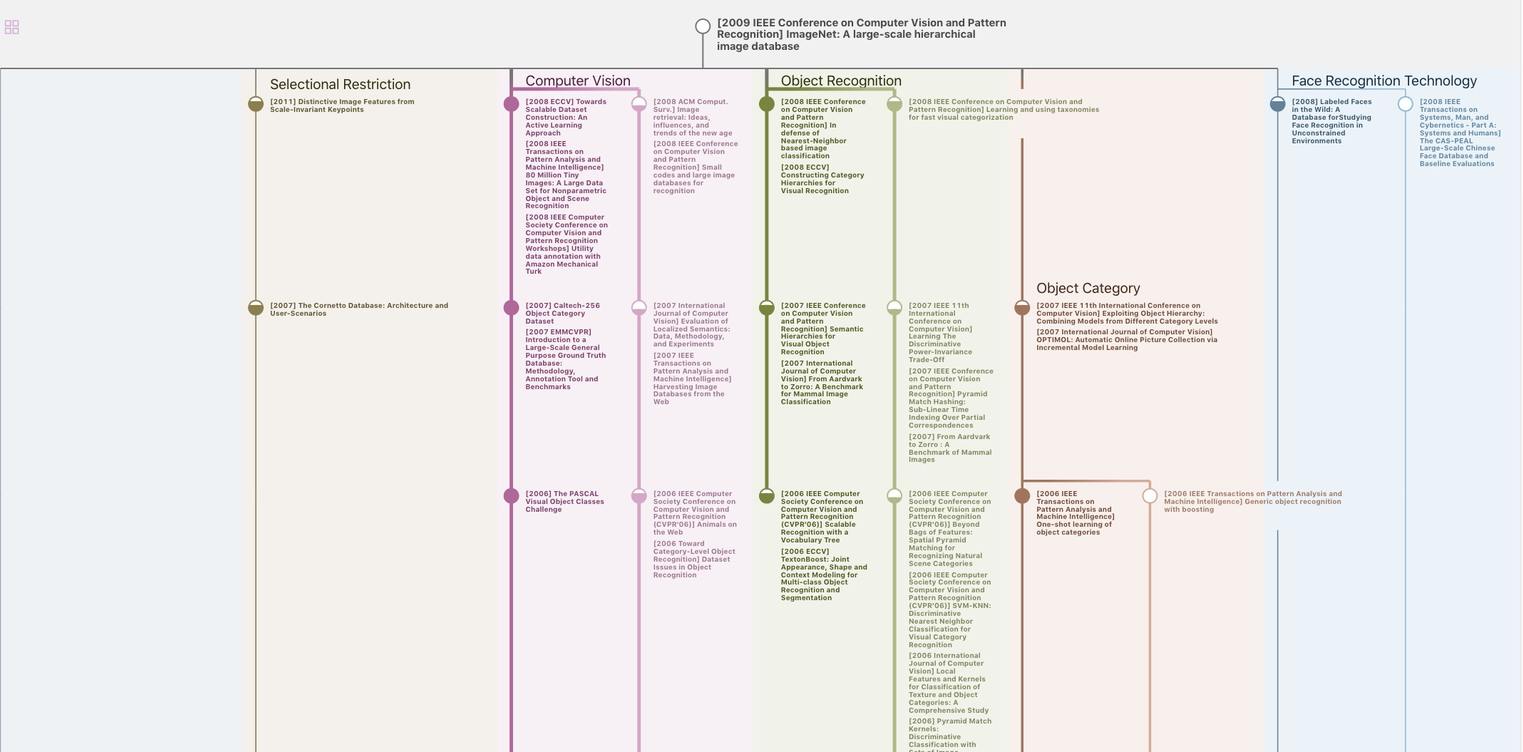
生成溯源树,研究论文发展脉络
Chat Paper
正在生成论文摘要