Uncorrelated Multi-View Discrimination Dictionary Learning For Recognition
AAAI(2014)
摘要
Dictionary learning (DL) has now become an important feature learning technique that owns state-of-the-art recognition performance. Due to sparse characteristic of data in real-world applications, DL uses a set of learned dictionary bases to represent the linear decomposition of a data point. Fisher discrimination DL (FDDL) is a representative supervised DL method, which constructs a structured dictionary whose atoms correspond to the class labels. Recent years have witnessed a growing interest in multi-view (more than two views) feature learning techniques. Although some multi-view (or multi-modal) DL methods have been presented, there still exists much room for improvement. How to enhance the total discriminability of dictionaries and reduce their redundancy is a crucial research topic. To boost the performance of multi-view DL technique, we propose an uncorrelated multi-view discrimination DL ((UMDL)-L-2) approach for recognition. By making dictionary atoms correspond to the class labels such that the obtained reconstruction error is discriminative, (UMDL)-L-2 aims to jointly learn multiple dictionaries with totally favorable discriminative power. Furthermore, we design the uncorrelated constraint for multi-view DL, so as to reduce the redundancy among dictionaries learned from different views. Experiments on several public datasets demonstrate the effectiveness of the proposed approach.
更多查看译文
关键词
recognition,discrimination,learning,multi-view
AI 理解论文
溯源树
样例
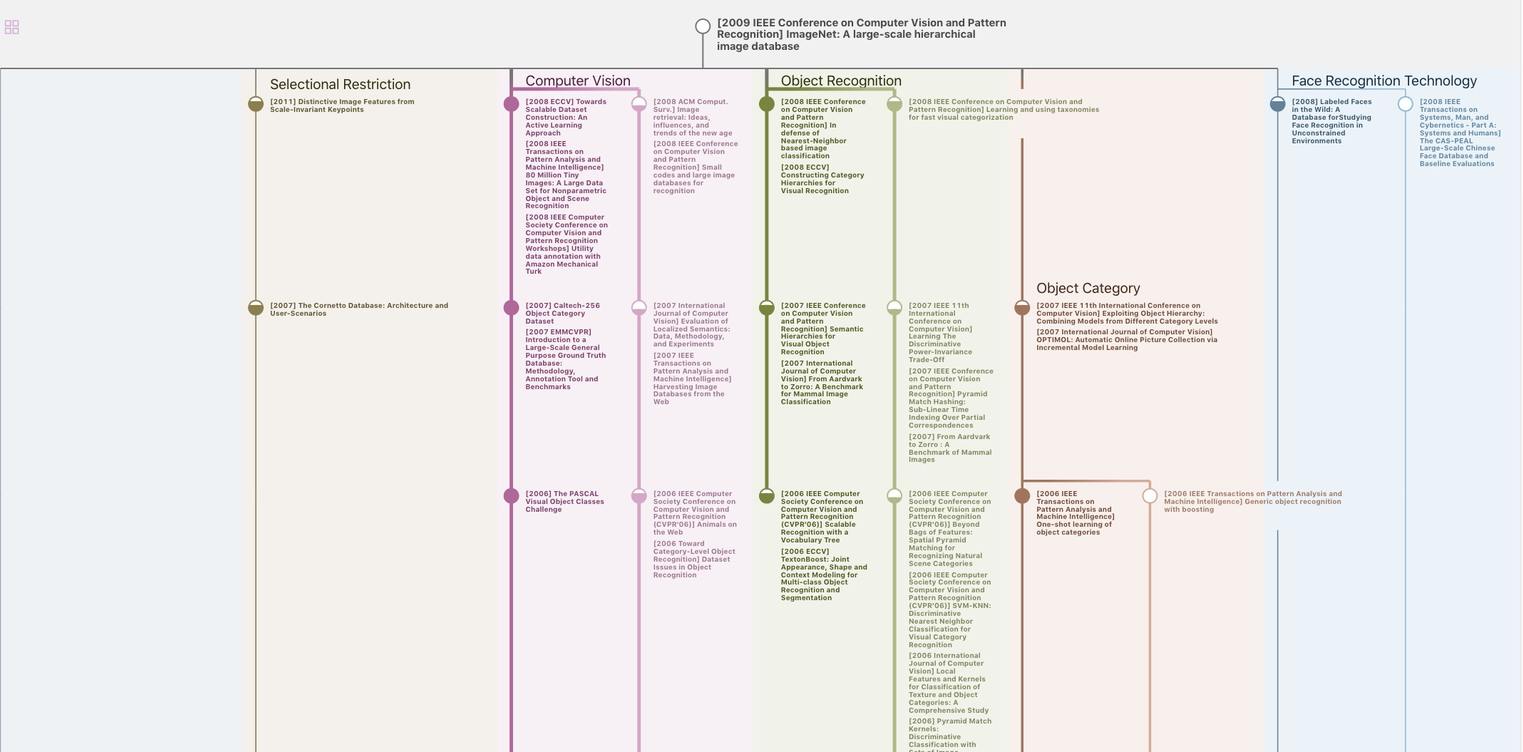
生成溯源树,研究论文发展脉络
Chat Paper
正在生成论文摘要