Online Boosting Algorithms For Anytime Transfer And Multitask Learning
AAAI'15: Proceedings of the Twenty-Ninth AAAI Conference on Artificial Intelligence(2015)
摘要
The related problems of transfer learning and multitask learning have attracted significant attention, generating a rich literature of models and algorithms. Yet most existing approaches are studied in an offline fashion, implicitly assuming that data from different domains are given as a batch. Such an assumption is not valid in many real-world applications where data samples arrive sequentially, and one wants a good learner even from few examples. The goal of our work is to provide sound extensions to existing transfer and multitask learning algorithms such that they can be used in an anytime setting. More specifically, we propose two novel online boosting algorithms, one for transfer learning and one for multitask learning, both designed to leverage the knowledge of instances in other domains. The experimental results show state-of-the-art empirical performance on standard benchmarks, and we present results of using our methods for effectively detecting new seizures in patients with epilepsy from very few previous samples.
更多查看译文
AI 理解论文
溯源树
样例
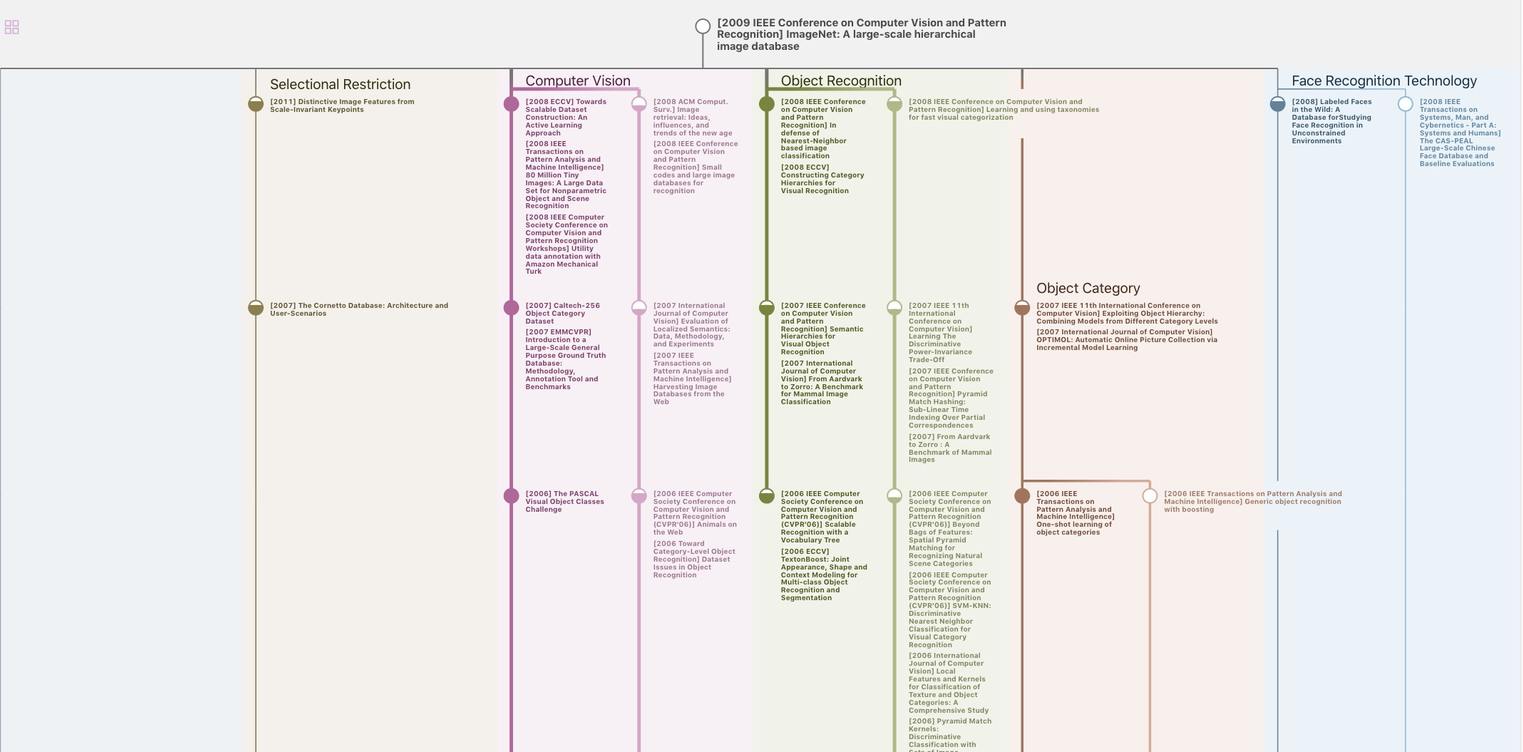
生成溯源树,研究论文发展脉络
Chat Paper
正在生成论文摘要