Unsupervised Induction Of Meaningful Semantic Classes Through Selectional Preferences
COMPUTATIONAL LINGUISTICS AND INTELLIGENT TEXT PROCESSING (CICLING 2015), PT I(2015)
摘要
This paper addresses the general task of semantic class learning by introducing a methodology to induce semantic classes for labeling instances of predicate arguments in an input text. The proposed methodology takes a Proposition Store as Background Knowledge Base to firstly identify a set of classes capable of representing the arguments of predicates in the store; where the classes corresponds to common nouns from the store to support interpretability. Then, it learns a selectional preference model for predicates based on tuples of classes to set up a generative model of propositions from which to perform the induction of classes. The proposed method is completely unsupervised and rely on a reference collection of unlabeled text documents used as the source of background knowledge to build the proposition store. We demonstrate our proposal on a collection of news stories. Specifically, we evaluate the learned model in the task of predicting tuples of argument instances for predicates from held-aside data.
更多查看译文
关键词
Semantic class learning, semantic class induction, generative model of selectional preferences
AI 理解论文
溯源树
样例
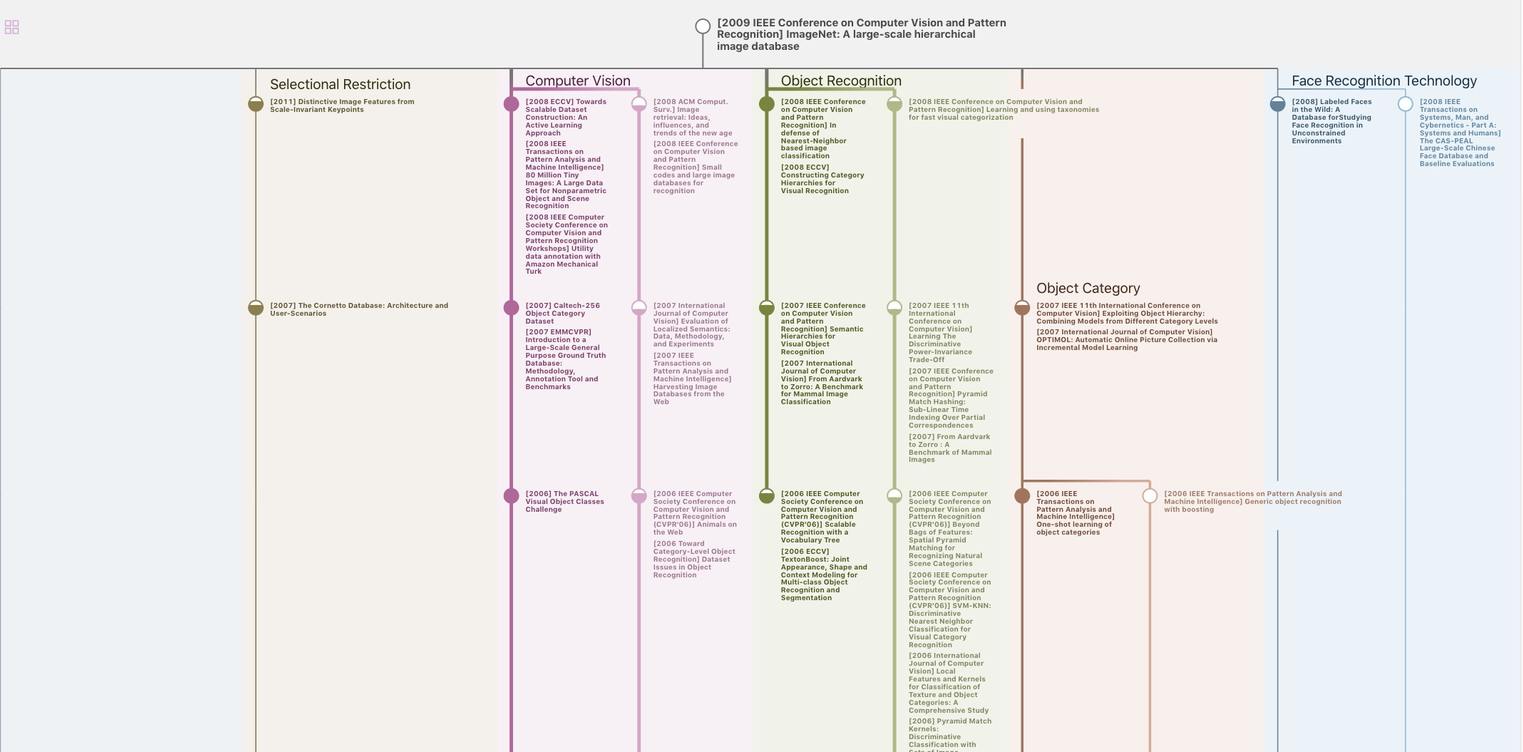
生成溯源树,研究论文发展脉络
Chat Paper
正在生成论文摘要