Stretching the Life of Twitter Classifiers with Time-Stamped Semantic Graphs
International Semantic Web Conference(2014)
摘要
Social media has become an effective channel for communicating both trends and public opinion on current events. However the automatic topic classification of social media content pose various challenges. Topic classification is a common technique used for automatically capturing themes that emerge from social media streams. However, such techniques are sensitive to the evolution of topics when new event-dependent vocabularies start to emerge (e.g., Crimea becoming relevant to War_Conflict during the Ukraine crisis in 2014). Therefore, traditional supervised classification methods which rely on labelled data could rapidly become outdated. In this paper we propose a novel transfer learning approach to address the classification task of new data when the only available labelled data belong to a previous epoch. This approach relies on the incorporation of knowledge from DBpedia graphs. Our findings show promising results in understanding how features age, and how semantic features can support the evolution of topic classifiers.
更多查看译文
关键词
feature relevance decay,dbpedia,social media,topic detection,concept drift
AI 理解论文
溯源树
样例
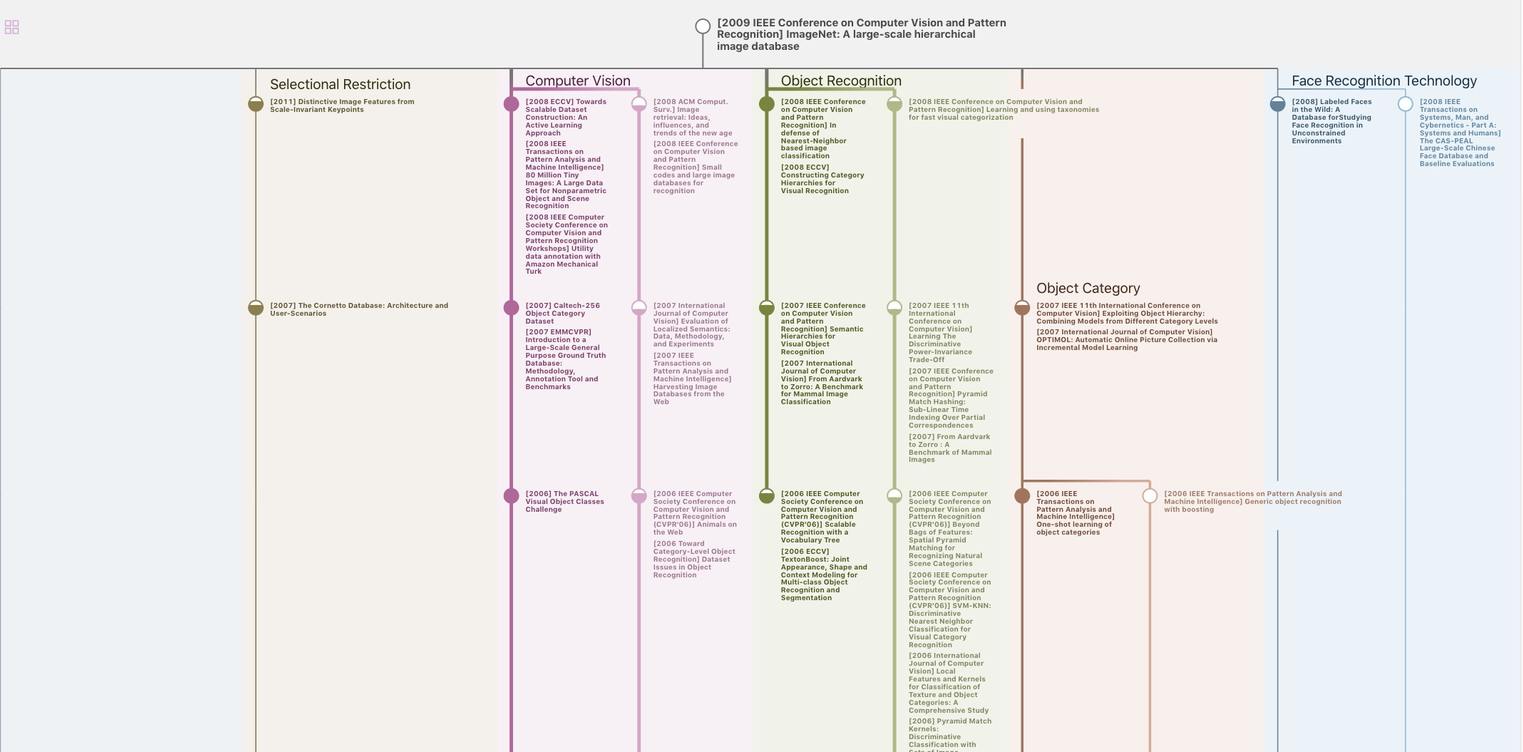
生成溯源树,研究论文发展脉络
Chat Paper
正在生成论文摘要