On Efficient Meta-Level Features for Effective Text Classification.
CIKM '14: 2014 ACM Conference on Information and Knowledge Management Shanghai China November, 2014(2014)
摘要
This paper addresses the problem of automatically learning to classify texts by exploiting information derived from meta-level features (i.e., features derived from the original bag-of-words representation). We propose new meta-level features derived from the class distribution, the entropy and the within-class cohesion observed in the k nearest neighbors of a given test document x, as well as from the distribution of distances of x to these neighbors. The set of proposed features is capable of transforming the original feature space into a new one, potentially smaller and more informed. Experiments performed with several standard datasets demonstrate that the effectiveness of the proposed meta-level features is not only much superior than the traditional bag-of-word representation but also superior to other state-of-art meta-level features previously proposed in the literature. Moreover, the proposed meta-features can be computed about three times faster than the existing meta-level ones, making our proposal much more scalable. We also demonstrate that the combination of our meta features and the original set of features produce significant improvements when compared to each feature set used in isolation.
更多查看译文
AI 理解论文
溯源树
样例
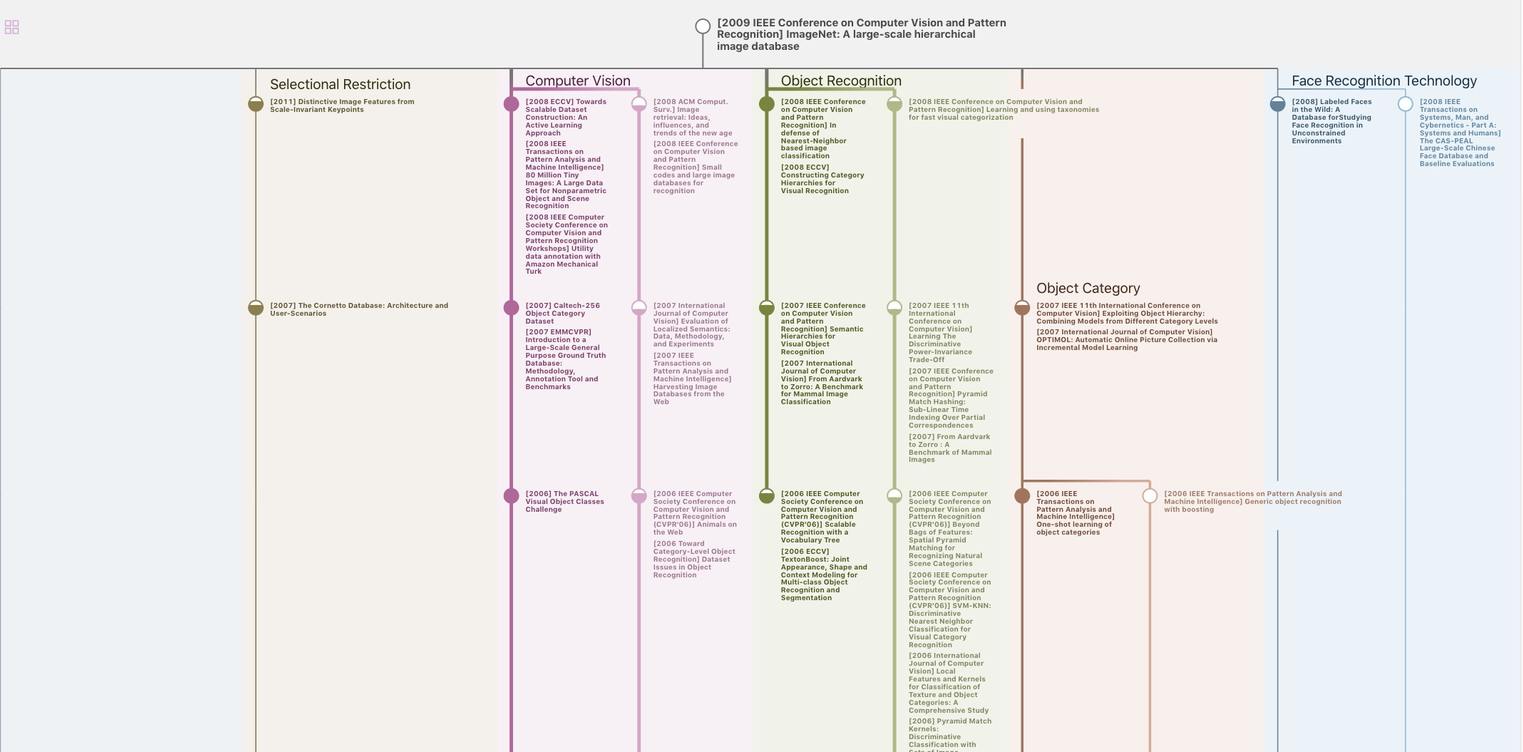
生成溯源树,研究论文发展脉络
Chat Paper
正在生成论文摘要