Novel Query Suggestions: Initial Work Report.
CIKM(2014)
摘要
ABSTRACTQuery auto-completion (QAC) is one of the most recognizable and widely used services of modern search engines. Its goal is to assist a user in the process of query formulation. Current QAC systems are mainly reactive. They respond to the present request using past knowledge. Specifically, they mostly rely on query logs analysis or corpus terms co-occurrences and rank suggestions according to their similarity with the partial user query, their past popularity, or their temporal dynamics features (e.g. trends, bursts, seasonality in query popularity). Consequently, a suggestion to be recommended by the QAC system must be preceded with a substantial users' interest and ipso facto must be an old information. However, a growing amount of people turns to search engines to find novel information, that is emergent or recently created (not redundant) one. Conventional QAC systems are thus unable to fulfill the increasingly real-time needs of the users. In this work-in-progress report, we introduce a new approach to QAC - the system filtering out potentially novel information and proactively delivering it to the users. It aims at providing the users with some novel insight. Thus, it caters for their open-ended or persistent and increasingly real-time information needs. The preliminary method proposed in this paper to evaluate this approach forms time specific suggestions based on a comparison of two corpora constantly being updated with new data from chosen sources. An unsupervised and language-independent algorithm relying on relative novelty of terms co-occurrences is used to generate suggestions. The initial experimental results demonstrate the effectiveness of the approach in recommending queries leading to novel information. Therefore, they prove that such a system can enhance the exploratory power of a search engine and support the proactive information search.
更多查看译文
AI 理解论文
溯源树
样例
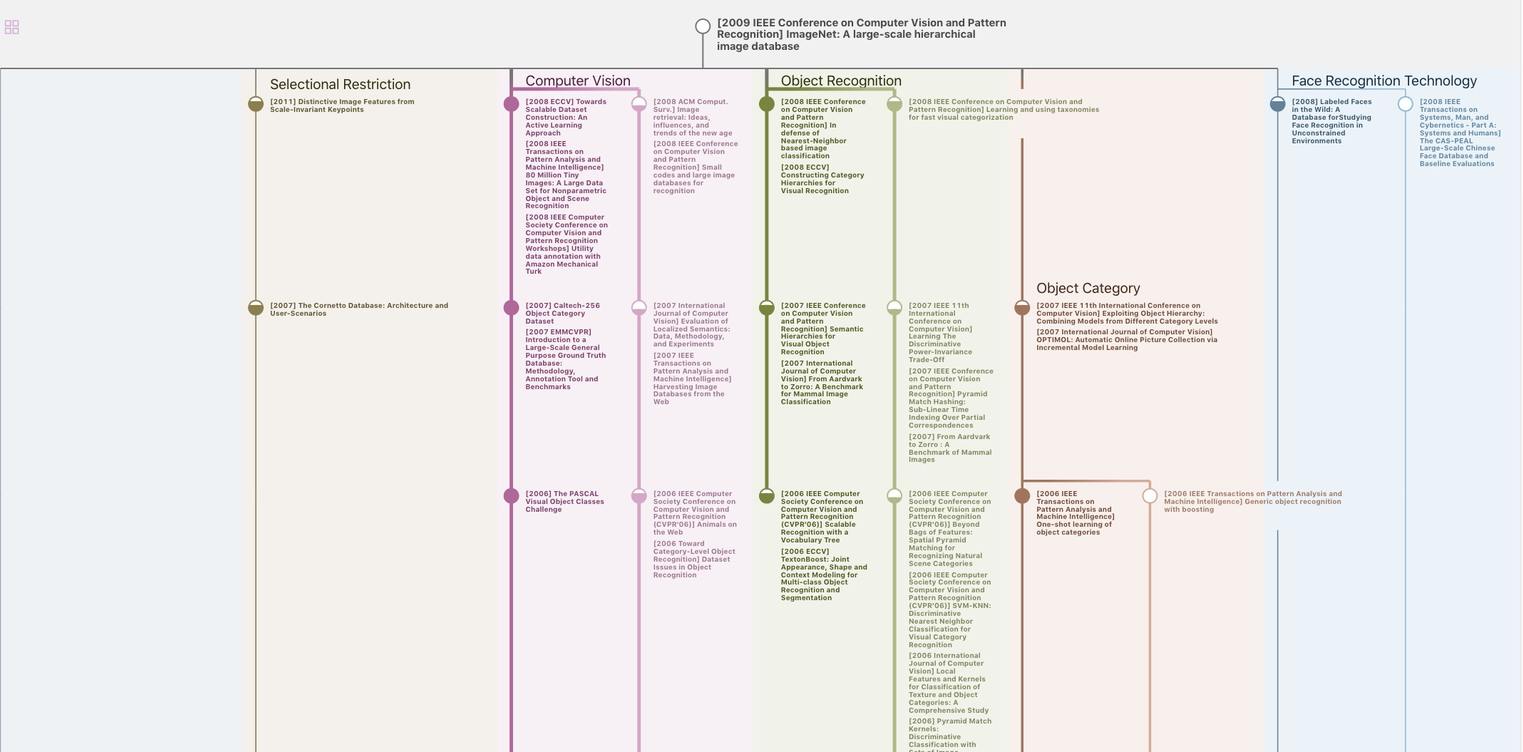
生成溯源树,研究论文发展脉络
Chat Paper
正在生成论文摘要