Supervised Hashing with Soft Constraints.
CIKM '14: 2014 ACM Conference on Information and Knowledge Management Shanghai China November, 2014(2014)
摘要
Due to the ability to preserve semantic similarity in Hamming space, supervised hashing has been extensively studied recently. Most existing approaches encourage two dissimilar samples to have maximum Hamming distance. This may lead to an unexpected consequence that two unnecessarily similar samples would have the same code if they are both dissimilar with another sample. Besides, in existing methods, all labeled pairs are treated with equal importance without considering the semantic gap, which is not conducive to thoroughly leverage the supervised information. We present a general framework for supervised hashing to address the above two limitations. We do not toughly require a dissimilar pair to have maximum Hamming distance. Instead, a soft constraint which can be viewed as a regularization to avoid over-fitting is utilized. Moreover, we impose different weights to different training pairs, and these weights can be automatically adjusted in the learning process. Experiments on two benchmarks show that the proposed method can easily outperform other state-of-the-art methods.
更多查看译文
AI 理解论文
溯源树
样例
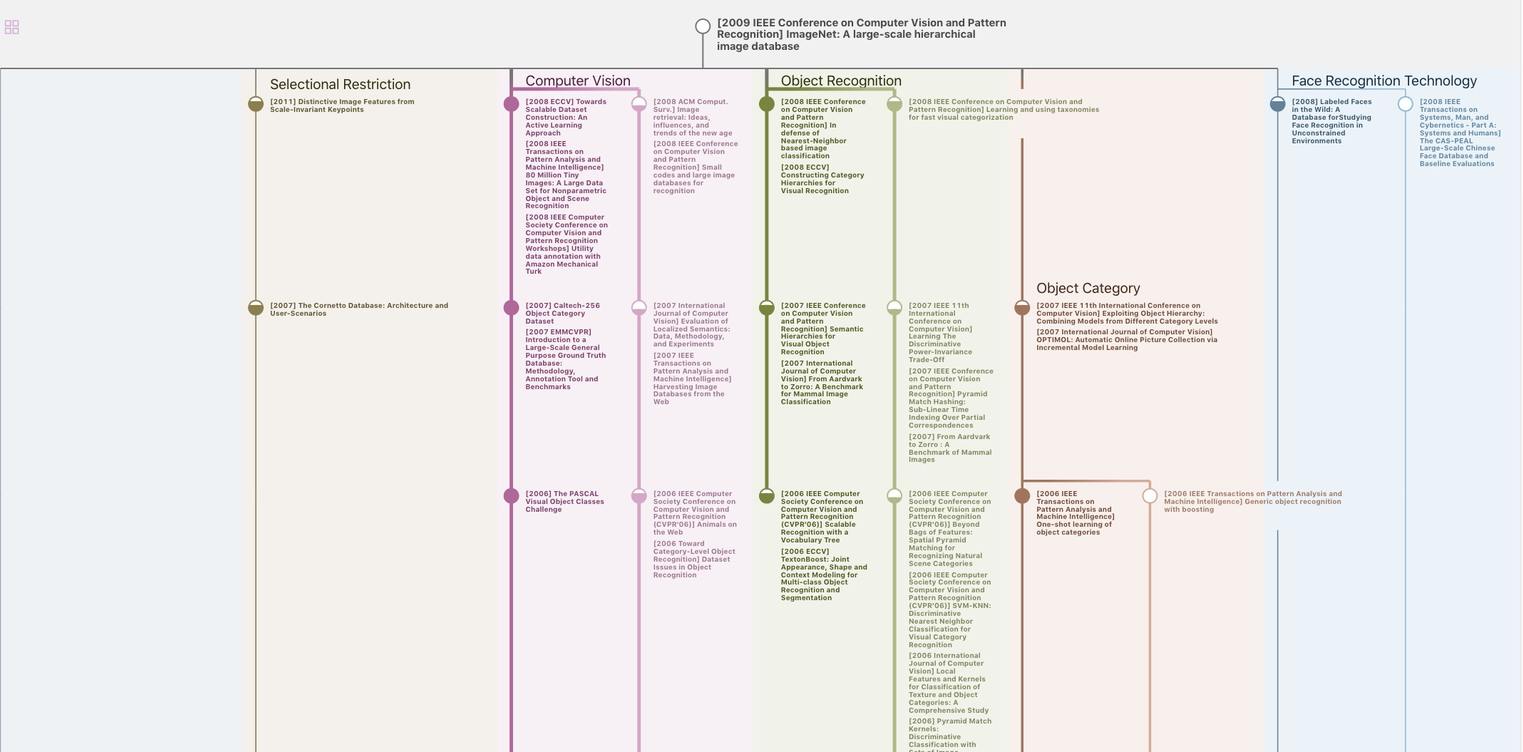
生成溯源树,研究论文发展脉络
Chat Paper
正在生成论文摘要