Robust Principal Component Analysis with Missing Data.
CIKM '14: 2014 ACM Conference on Information and Knowledge Management Shanghai China November, 2014(2014)
摘要
Recovering matrices from incomplete and corrupted observations is a fundamental problem with many applications in various areas of science and engineering. In theory, under certain conditions, this problem can be solved via a natural convex relaxation. However, all current provable algorithms suffer from superlinear per-iteration cost, which severely limits their applicability to large scale problems. In this paper, we propose a robust principal component analysis (RPCA) plus matrix completion framework to recover low-rank and sparse matrices from missing and grossly corrupted observations. Under the unified framework, we first present a convex robust matrix completion model to replace the linear projection operator constraint by a simple equality one. To further improve the efficiency of our convex model, we also develop a scalable structured factorization model, which can yield an orthogonal dictionary and a robust data representation simultaneously. Then, we develop two alternating direction augmented Lagrangian (ADAL) algorithms to efficiently solve the proposed problems. Finally, we discuss the convergence analysis of our algorithms. Experimental results verified both the efficiency and effectiveness of our methods compared with the state-of-the-art algorithms.
更多查看译文
AI 理解论文
溯源树
样例
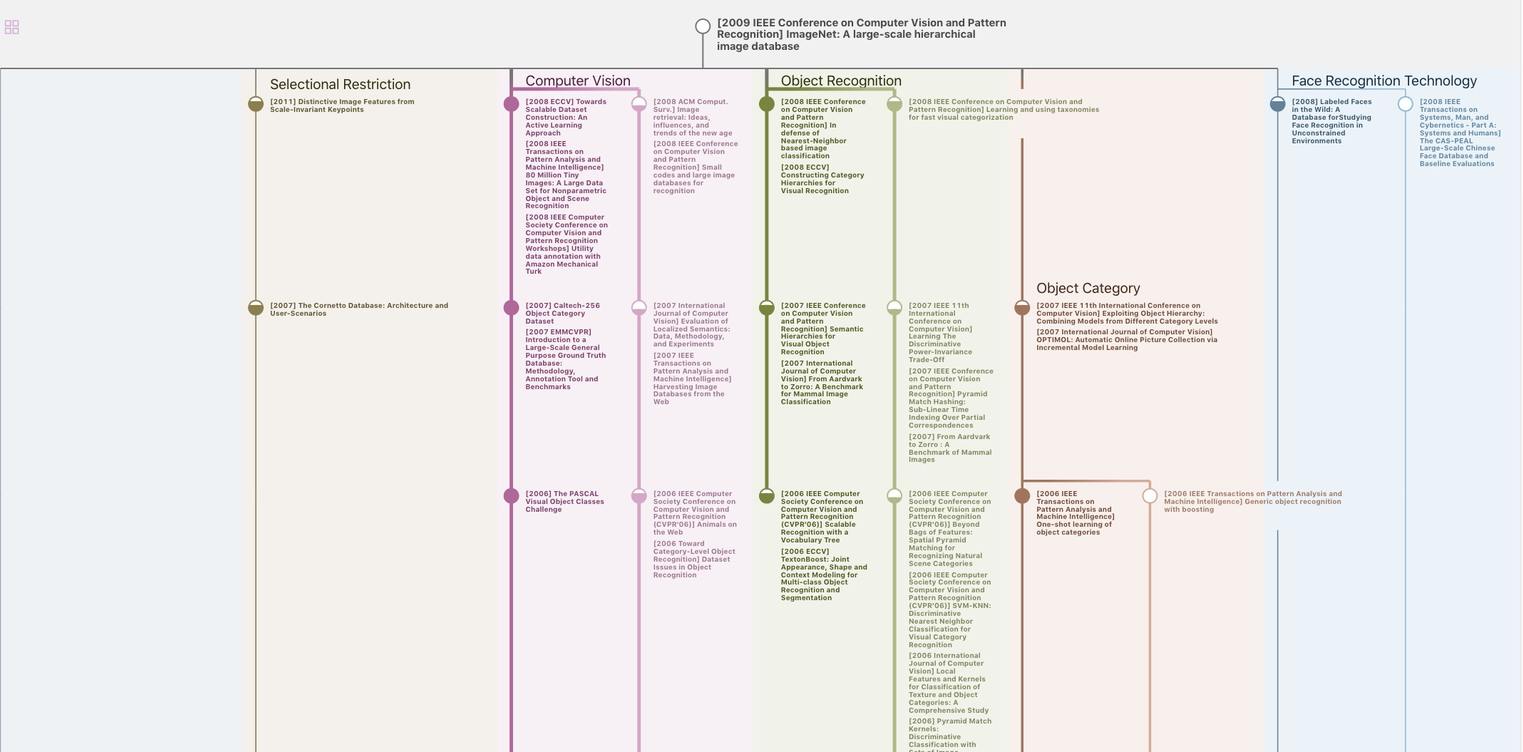
生成溯源树,研究论文发展脉络
Chat Paper
正在生成论文摘要