Robust Support Vector Machine
IJCNN(2014)
摘要
Support Vector Machine (SVM) is a well-known kernel-based method for binary classification problem. SVM aims at constructing the optimal middle hyperplane which induces the largest margin. It is proven that in a linearly separable case, this middle hyperplane offers the high accuracy on universal datasets. However, real world datasets often contain overlapping regions and therefore, the decision hyperplane should be adjusted according to the profiles of the datasets. In this paper, we propose Robust Support Vector Machine (RSVM), where the hyperplanes can be properly adjusted to accommodate the real world datasets. By setting the value of the adjustment factor properly, RSVM can handle well the datasets with any possible profiles. Our experiments on the benchmark datasets demonstrate the superiority of the RSVM for both binary and one-class classification problems.
更多查看译文
关键词
one-class classification problems,binary classification problem,pattern classification,decision hyperplane,robust support vector machine,kernel-based method,optimal middle hyperplane,one-class support vector machine,universal datasets,support vector machine,rsvm,support vector machines,gaussian distribution,robustness,kernel
AI 理解论文
溯源树
样例
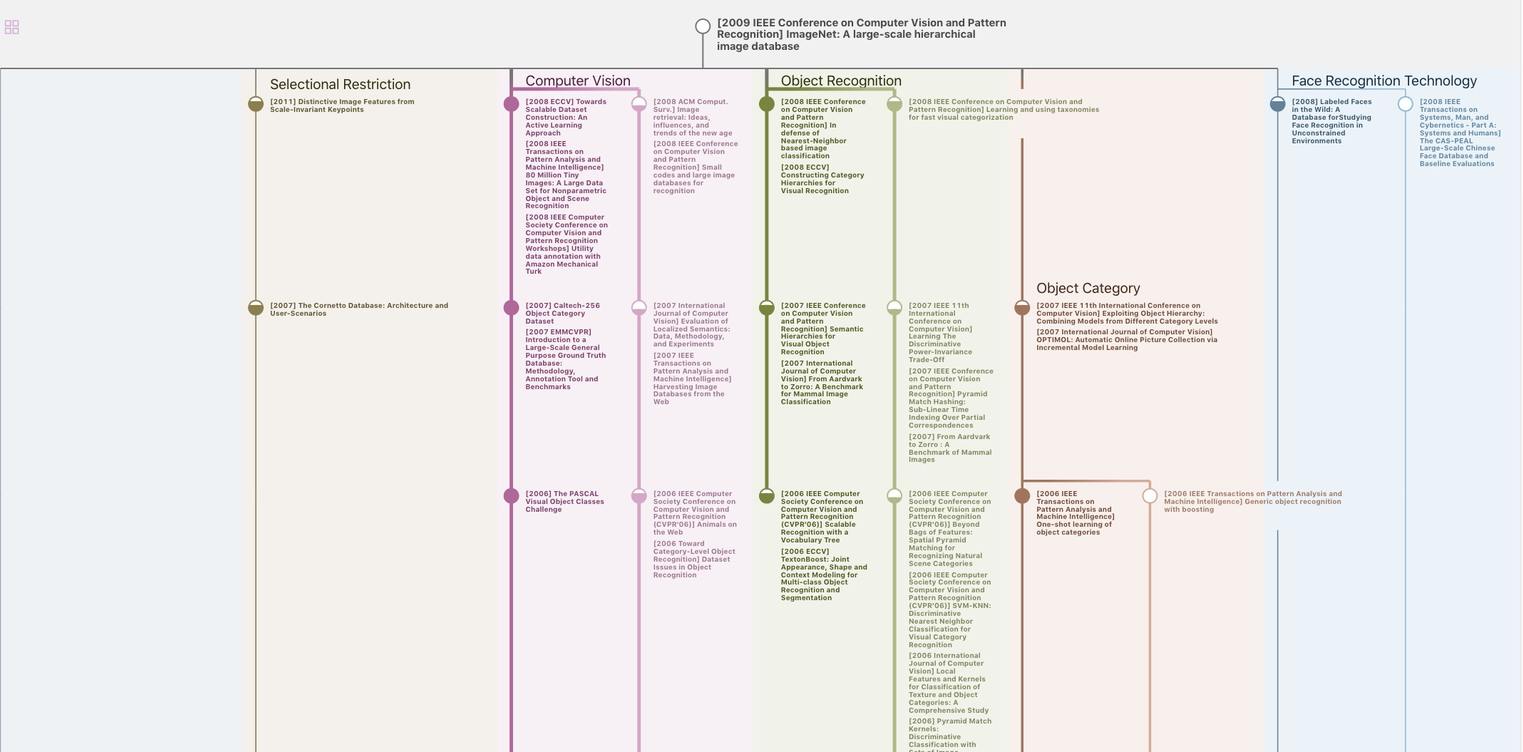
生成溯源树,研究论文发展脉络
Chat Paper
正在生成论文摘要