A Grid-Scan Maximum Likelihood Estimation With A Bias Function For Indoor Network Localization
2013 INTERNATIONAL CONFERENCE ON INDOOR POSITIONING AND INDOOR NAVIGATION (IPIN)(2013)
摘要
Indoor range-based localization always suffers from imprecise range measurements, especially non-Iine-of-sight (N-LOS) errors. Probabilistic approaches like maximum likelihood estimation (ML) are efficient under measurement errors; however, to get the optimal solution poses a non-convex problem stagnating to local optima instead of the global optimum. More important, the convergence of the objective function can be greatly misled by the inappropriately modeled NLOS errors. We propose a grid-scan approach to approximate the global convergence of ML estimate and a bias function against the positive indoor ranging error and the NLOS effect, namely GML(b). GML(b) combines the robustness of Bounding-box algorithm and the reality of an exponentially bias function to indoor ranging characteristics: the Bounding-box intersection ensures that the NLOS ranges cannot deviate the estimate far away; further, the bias function tunings the model to be specific for positive ranging errors. Monte Carlo simulations and real-world indoor experiments are implemented to investigate the efficiency of the strategies against the non-convex and NLOS problems. The Monte Carlo simulations demonstrate that GML(b) achieves comparable accuracy to ML in an ideal case and higher robustness than ML series algorithms in the NLOS case. The experiment reveals that GML(b) is superior in scenarios rich of positive ranging errors, in particular, GML(b) performs the best in worst case. And GML(b) works well with a small number of grids, causing a low computational requirement on the ability-limited network devices.
更多查看译文
关键词
Indoor network localization, the NLOS error, maximum likelihood, non-convex optimization, positive ranging errors
AI 理解论文
溯源树
样例
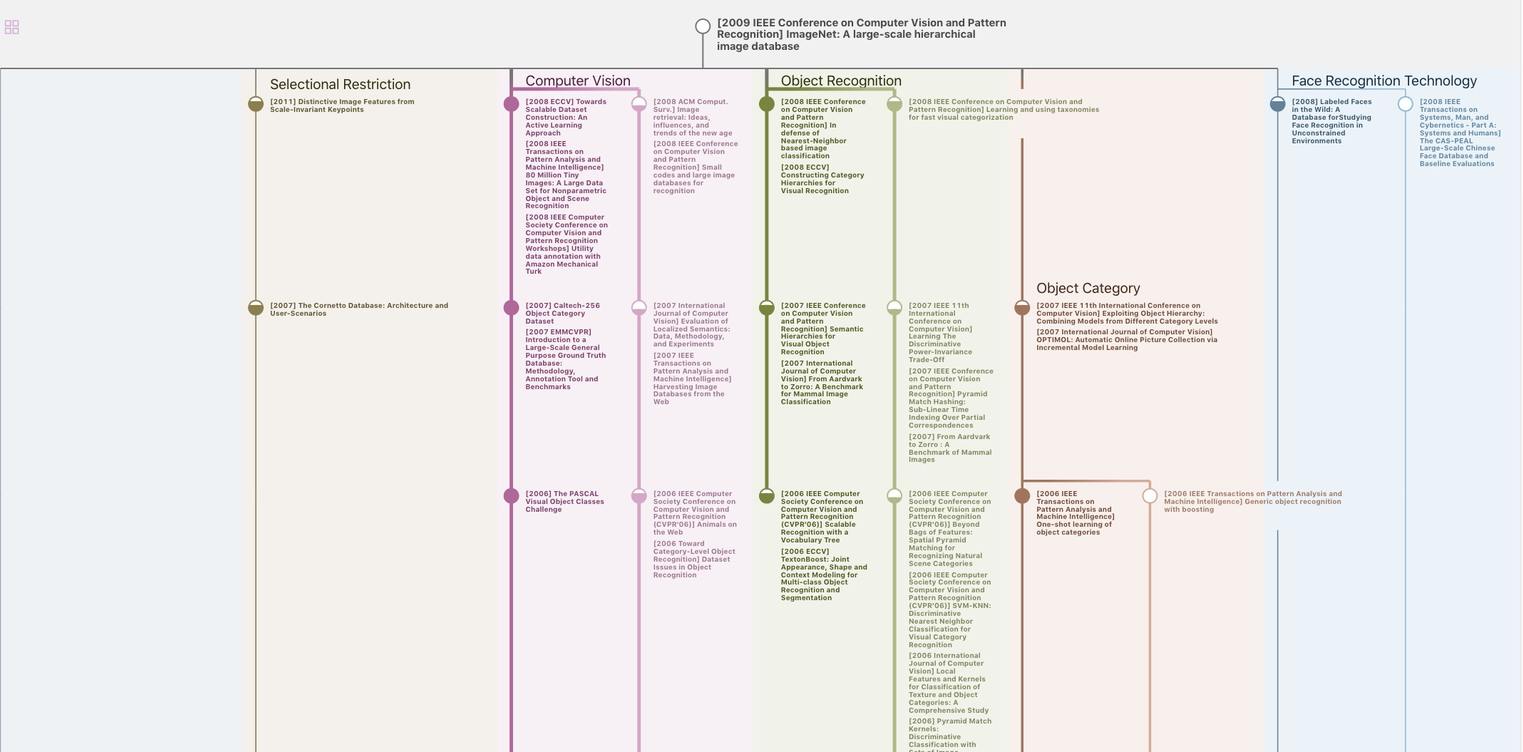
生成溯源树,研究论文发展脉络
Chat Paper
正在生成论文摘要