Gradient-based image up-scaling with local self similarity
GlobalSIP(2014)
摘要
For image up-scaling, blurs and ringing at the edges or contours of the high frequency components in the super resolution image is one of the major problems. The local-self-example image up-scaling method observe that small patches are similar to themselves upon small scaling factors so they use small patch search to reconstruct the high frequency components. Since the high frequency components contain mainly the contour and edge information, we propose a gradient based searching approach that utilizes the features of the high frequency components to extract similar patches. We present the 1-D and 2-D based gradient search for reconstructing the high frequency components in the super resolution image. The 2-D gradient based search takes eight-direction derivations into consideration so that most edge variations are well preserved. In addition, the found patches have high structure similarity between the original image and the up-scaling image. Compared with other methods, the reconstructed high frequency components of our proposed method maintain more edge and contour details. All our proposed approaches have better PSNR and SSIM values than Freedmanef al's[1] approach. From the simulation, the more directions in the gradient are considered, the results are more close to the original images.
更多查看译文
关键词
gradient-based image up-scaling,gradient based searching approach,2d based gradient search,high frequency component reconstruction,local-self similarity,super resolution,small scaling factors,patch extraction,image resolution,gradient-based search,fractals,edge variations,psnr values,image reconstruction,small patch search,superresolution image,feature extraction,gradient methods,contour information,edge information,image up-scaling,local-self-example image up-scaling method,local self similarity,ssim values,1d based gradient search,psnr,frequency domain analysis
AI 理解论文
溯源树
样例
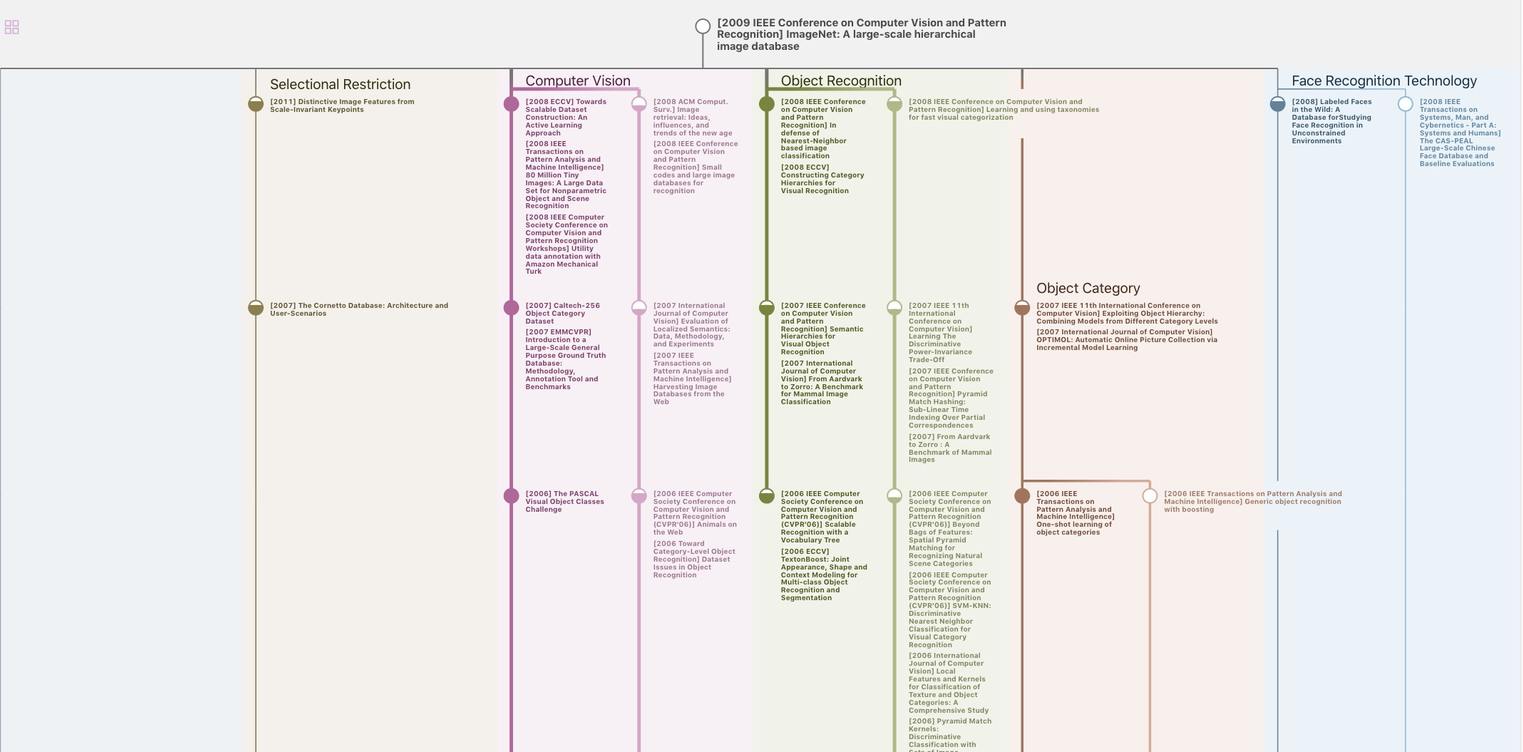
生成溯源树,研究论文发展脉络
Chat Paper
正在生成论文摘要