Complex stock trading strategy based on Particle Swarm Optimization
CIFEr(2012)
摘要
Trading rules have been utilized in the stock market to make profit for more than a century. However, only using a single trading rule may not be sufficient to predict the stock price trend accurately. Although some complex trading strategies combining various classes of trading rules have been proposed in the literature, they often pick only one rule for each class, which may lose valuable information from other rules in the same class. In this paper, a complex stock trading strategy, namely weight reward strategy (WRS), is proposed. WRS combines the two most popular classes of trading rules-moving average (MA) and trading range break-out (TRB). For both MA and TRB, WRS includes different combinations of the rule parameters to get a universe of 140 component trading rules in all. Each component rule is assigned a start weight and a reward/penalty mechanism based on profit is proposed to update these rules' weights over time. To determine the best parameter values of WRS, we employ an improved time variant Particle Swarm Optimization (PSO) algorithm with the objective of maximizing the annual net profit generated by WRS. The experiments show that our proposed WRS optimized by PSO outperforms the best moving average and trading range break-out rules.
更多查看译文
关键词
ma,stock price trend,weight reward strategy,single trading rule,pso,moving average,particle swarm optimisation,reward-penalty mechanism,stock markets,particle swarm optimization algorithm,wrs,trb,trading range break-out,pricing,complex stock trading strategy,optimization,radio frequency,testing,particle swarm optimization,vectors
AI 理解论文
溯源树
样例
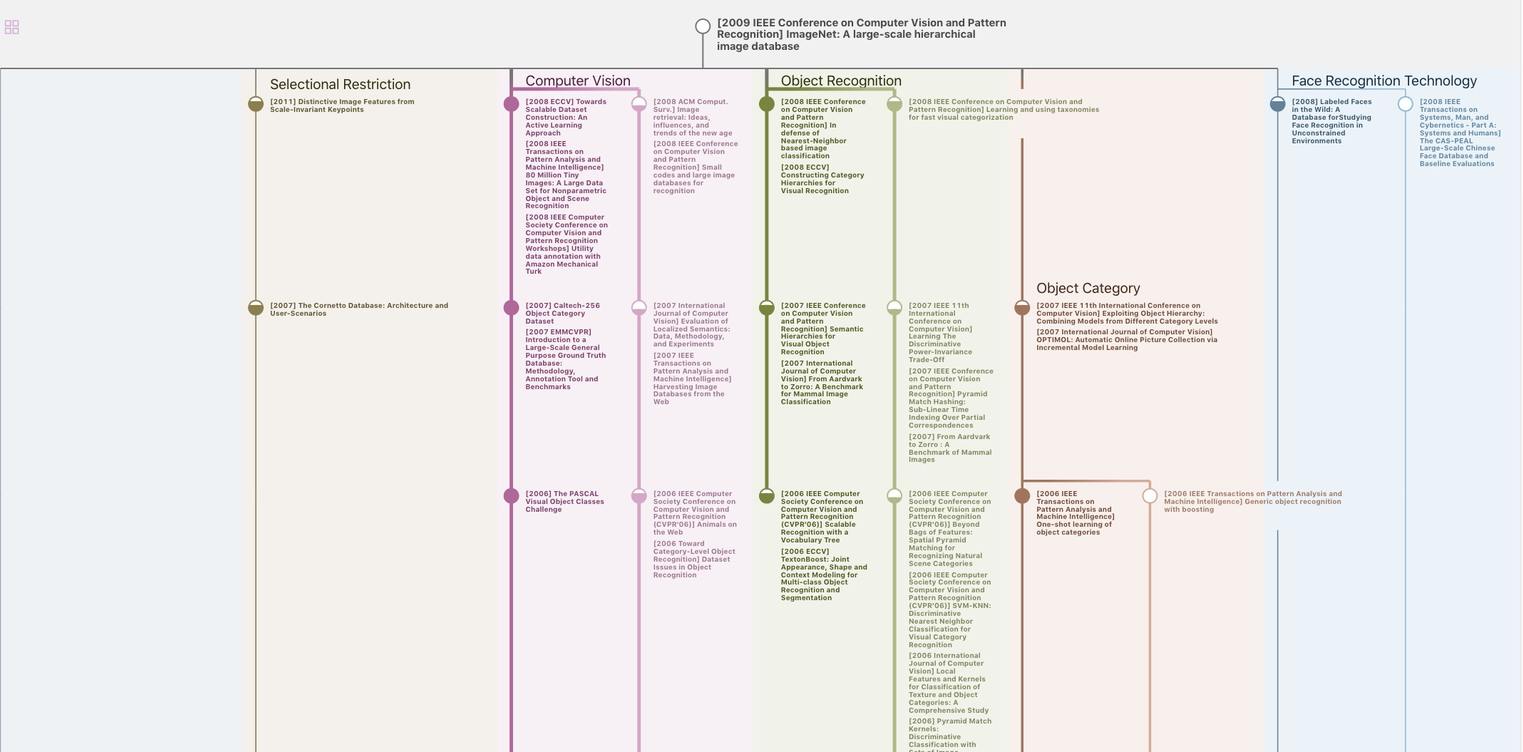
生成溯源树,研究论文发展脉络
Chat Paper
正在生成论文摘要