A machine learning approach for identifying anatomical locations of actionable findings in radiology reports.
AMIA ... Annual Symposium proceedings. AMIA Symposium(2012)
摘要
Recognizing the anatomical location of actionable findings in radiology reports is an important part of the communication of critical test results between caregivers. One of the difficulties of identifying anatomical locations of actionable findings stems from the fact that anatomical locations are not always stated in a simple, easy to identify manner. Natural language processing techniques are capable of recognizing the relevant anatomical location by processing a diverse set of lexical and syntactic contexts that correspond to the various ways that radiologists represent spatial relations. We report a precision of 86.2%, recall of 85.9%, and F(1)-measure of 86.0 for extracting the anatomical site of an actionable finding. Additionally, we report a precision of 73.8%, recall of 69.8%, and F(1)-measure of 71.8 for extracting an additional anatomical site that grounds underspecified locations. This demonstrates promising results for identifying locations, while error analysis reveals challenges under certain contexts. Future work will focus on incorporating new forms of medical language processing to improve performance and transitioning our method to new types of clinical data.
更多查看译文
AI 理解论文
溯源树
样例
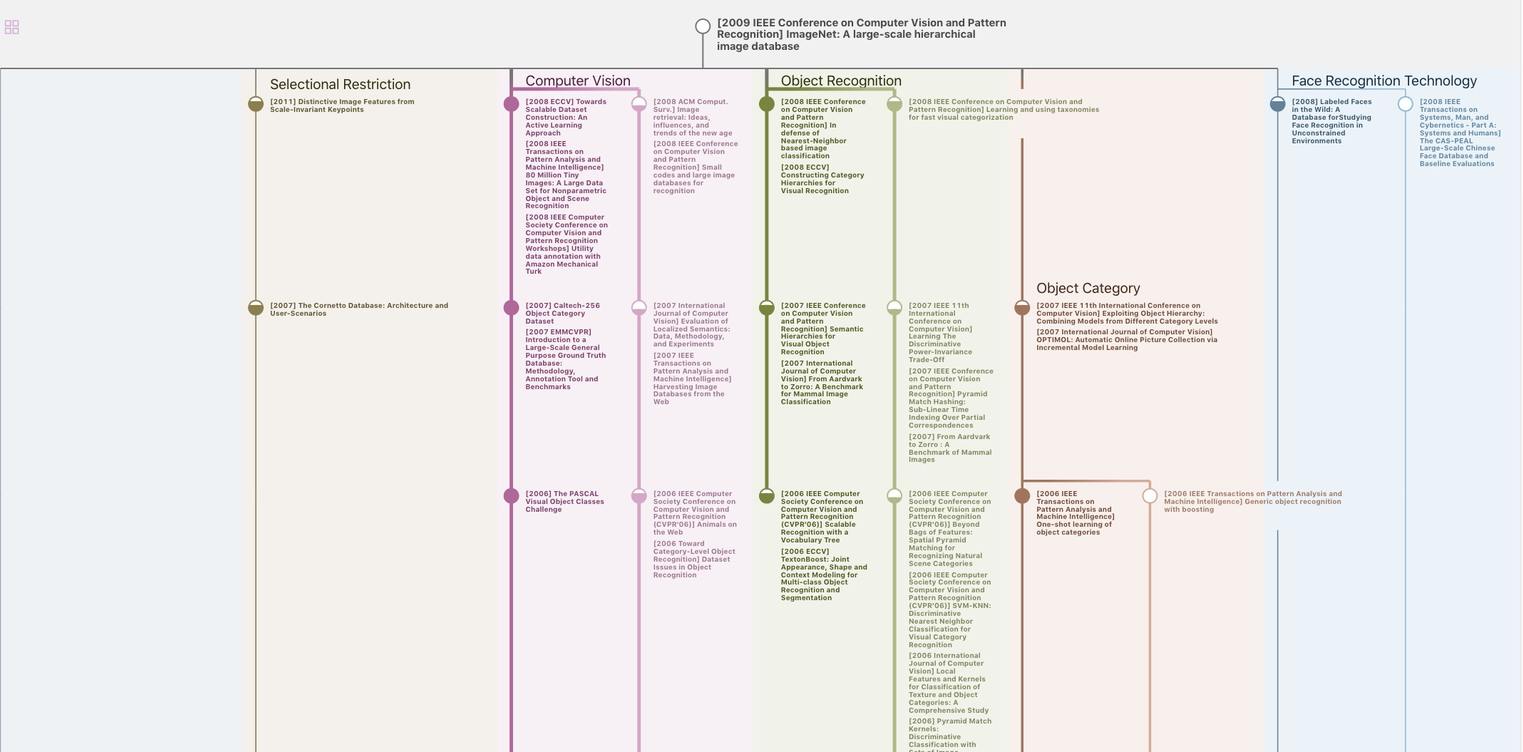
生成溯源树,研究论文发展脉络
Chat Paper
正在生成论文摘要