Testing the calibration of classification models from first principles.
AMIA(2012)
摘要
The accurate assessment of the calibration of classification models is severely limited by the fact that there is no easily available gold standard against which to compare a model's outputs. The usual procedures group expected and observed probabilities, and then perform a χ(2) goodness-of-fit test. We propose an entirely new approach to calibration testing that can be derived directly from the first principles of statistical hypothesis testing. The null hypothesis is that the model outputs are correct, i.e., that they are good estimates of the true unknown class membership probabilities. Our test calculates a p-value by checking how (im)probable the observed class labels are under the null hypothesis. We demonstrate by experiments that our proposed test performs comparable to, and sometimes even better than, the Hosmer-Lemeshow goodness-of-fit test, the de facto standard in calibration assessment.
更多查看译文
关键词
calibration,area under curve
AI 理解论文
溯源树
样例
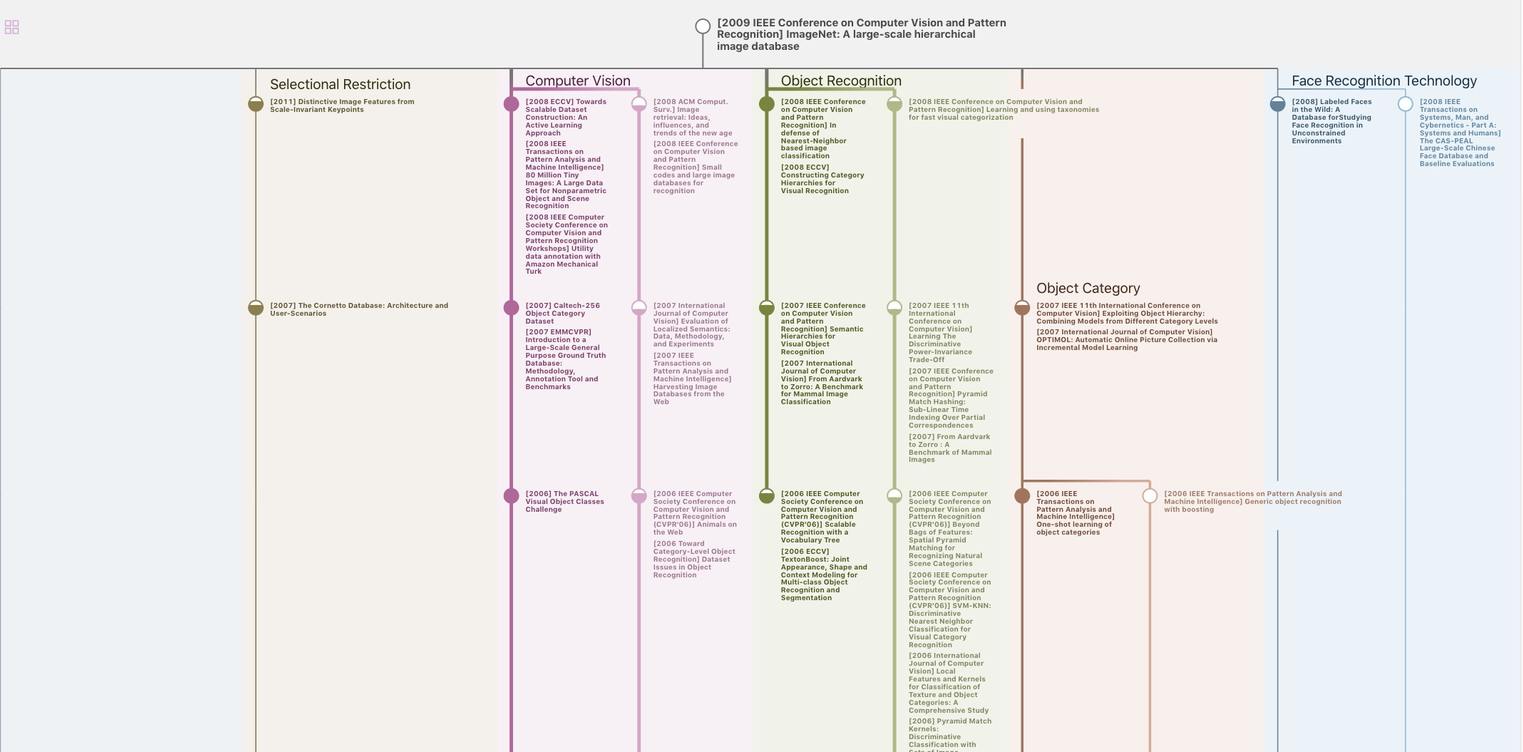
生成溯源树,研究论文发展脉络
Chat Paper
正在生成论文摘要