Unsupervised object individuation from RGB-D image sequences
IROS(2014)
摘要
In this paper, we propose a novel unified framework for unsupervised object individuation from RGB-D image sequences. The proposed framework integrates existing location-based and feature-based object segmentation methods to achieve both computational efficiency and robustness in unstructured and dynamic situations. Based on the infant's object indexing theory, the newly proposed ambiguity graph plays as a key component of the framework to detect falsely segmented objects and rectify them by using both location and feature information. In order to evaluate the proposed method, three table-top multiple object manipulation scenarios were performed: stacking, unstacking, and occluding tasks. The results showed that the proposed method is more robust than the location-only method and more efficient than the feature-only method.
更多查看译文
关键词
ambiguity graph,red-green-blue-depth image,unstacking manipulation,image segmentation,feature-based object segmentation,rgb-d image sequences,occluding manipulation,feature information,image sequences,stacking manipulation,graph theory,table-top multiple object manipulation,location information,falsely segmented object detection,unsupervised object individuation,object indexing theory,location-based object segmentation,image colour analysis,indexing,robustness,shape,gaussian distribution
AI 理解论文
溯源树
样例
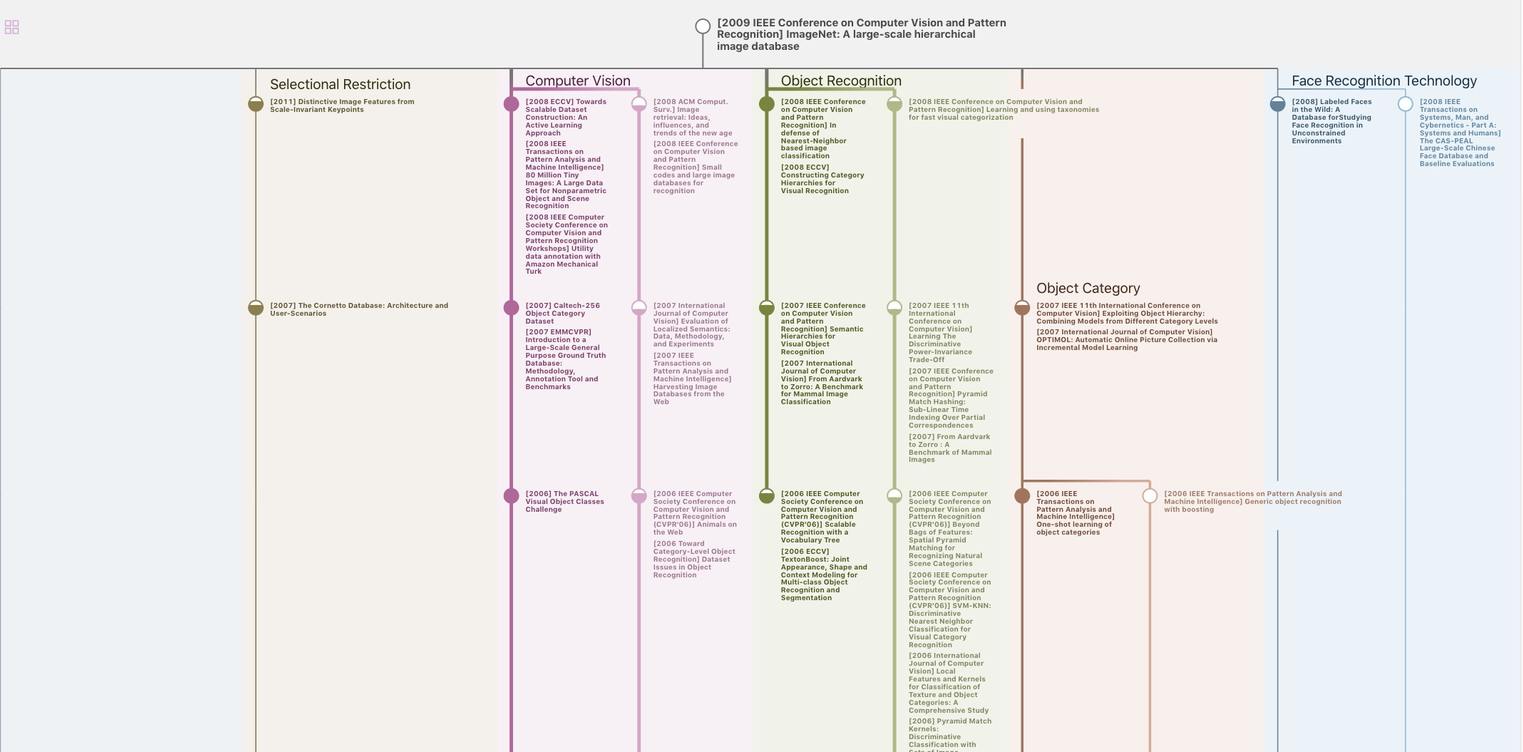
生成溯源树,研究论文发展脉络
Chat Paper
正在生成论文摘要