Compressive ELM: Improved Models through Exploiting Time-Accuracy Trade-Offs.
Communications in Computer and Information Science(2014)
摘要
In the training of neural networks, there often exists a trade-off between the time spent optimizing the model under investigation, and its final performance. Ideally, an optimization algorithm finds the model that has best test accuracy from the hypothesis space as fast as possible, and this model is efficient to evaluate at test time as well. However, in practice, there exists a trade-off between training time, testing time and testing accuracy, and the optimal trade-off depends on the user's requirements. This paper proposes the Compressive Extreme Learning Machine, which allows for a time-accuracy trade-off by training the model in a reduced space. Experiments indicate that this trade-off is efficient in the sense that on average more time can be saved than accuracy lost. Therefore, it provides a mechanism that can yield better models in less time.
更多查看译文
关键词
Extreme Learning Machine,ELM,random projection,compressive sensing,Johnson-Lindenstrauss,approximate matrix decompositions
AI 理解论文
溯源树
样例
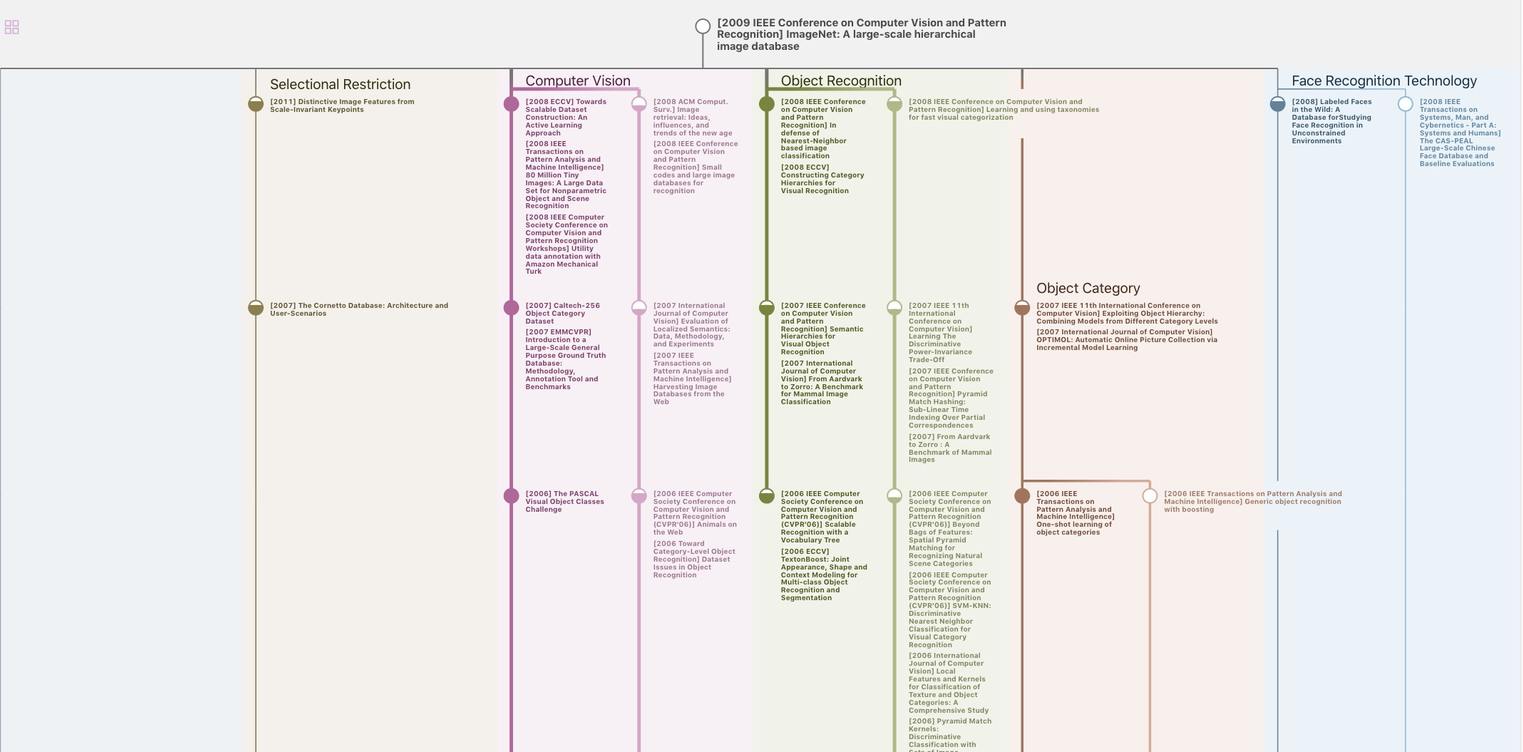
生成溯源树,研究论文发展脉络
Chat Paper
正在生成论文摘要