Recognizing Daily Activities In Realistic Environments Through Depth-Based User Tracking And Hidden Conditional Random Fields For Mci/Ad Support
COMPUTER VISION - ECCV 2014 WORKSHOPS, PT III(2014)
摘要
This paper presents a novel framework for the automatic recognition of Activities of Daily Living (ADLs), such as cooking, eating, dishwashing and watching TV, based on depth video processing and Hidden Conditional Random Fields (HCRFs). Depth video is provided by low-cost RGB-D sensors unobtrusively installed in the house. The user's location, posture, as well as point cloud -based features related to gestures are extracted; a standing/sitting posture detector, as well as novel features expressing head and hand gestures are introduced herein. To model the target activities, we employed discriminative HCRFs and compared them to HMMs. Through experimental evaluation, HCRFs outperformed HMMs in location trajectories-based ADL detection. By fusing trajectories data with posture and the proposed gesture features, ADL detection performance was found to further improve, leading to recognition rates at the level of 90.5% for five target activities in a naturalistic home environment.
更多查看译文
关键词
Adl recognition, User location trajectories, Posture, Gestures, Point-cloud features, Hidden conditional random fields
AI 理解论文
溯源树
样例
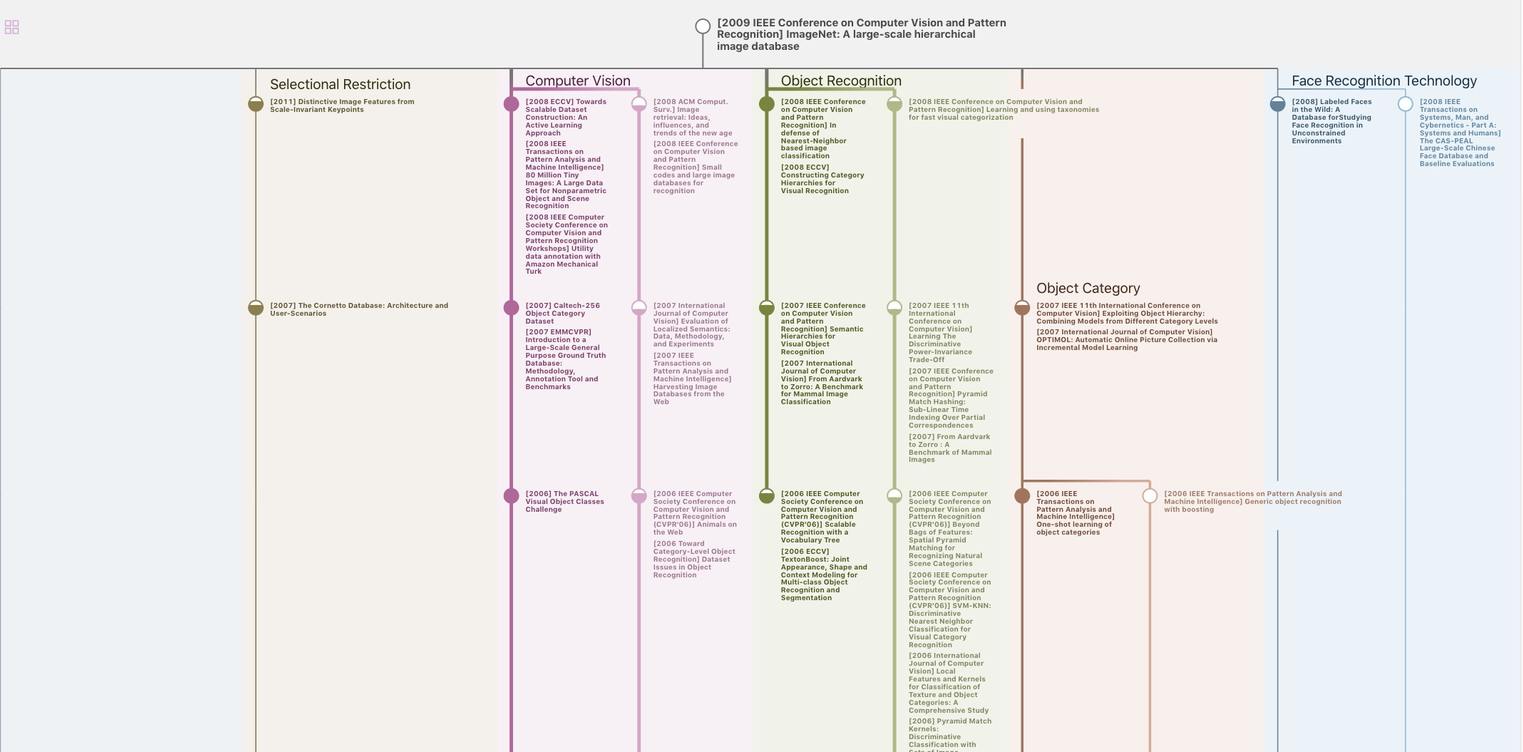
生成溯源树,研究论文发展脉络
Chat Paper
正在生成论文摘要