Interactively Guiding Semi-Supervised Clustering Via Attribute-Based Explanations
COMPUTER VISION - ECCV 2014, PT VI(2014)
摘要
Unsupervised image clustering is a challenging and often ill-posed problem. Existing image descriptors fail to capture the clustering criterion well, and more importantly, the criterion itself may depend on (unknown) user preferences. Semi-supervised approaches such as distance metric learning and constrained clustering thus leverage user-provided annotations indicating which pairs of images belong to the same cluster (must-link) and which ones do not (cannot-link). These approaches require many such constraints before achieving good clustering performance because each constraint only provides weak cues about the desired clustering. In this paper, we propose to use image attributes as a modality for the user to provide more informative cues. In particular, the clustering algorithm iteratively and actively queries a user with an image pair. Instead of the user simply providing a must-link/cannot-link constraint for the pair, the user also provides an attribute-based reasoning e. g. "these two images are similar because both are natural and have still water" or "these two people are dissimilar because one is way older than the other". Under the guidance of this explanation, and equipped with attribute predictors, many additional constraints are automatically generated. We demonstrate the effectiveness of our approach by incorporating the proposed attribute-based explanations in three standard semi-supervised clustering algorithms: Constrained K-Means, MPCK-Means, and Spectral Clustering, on three domains: scenes, shoes, and faces, using both binary and relative attributes.
更多查看译文
关键词
computer vision
AI 理解论文
溯源树
样例
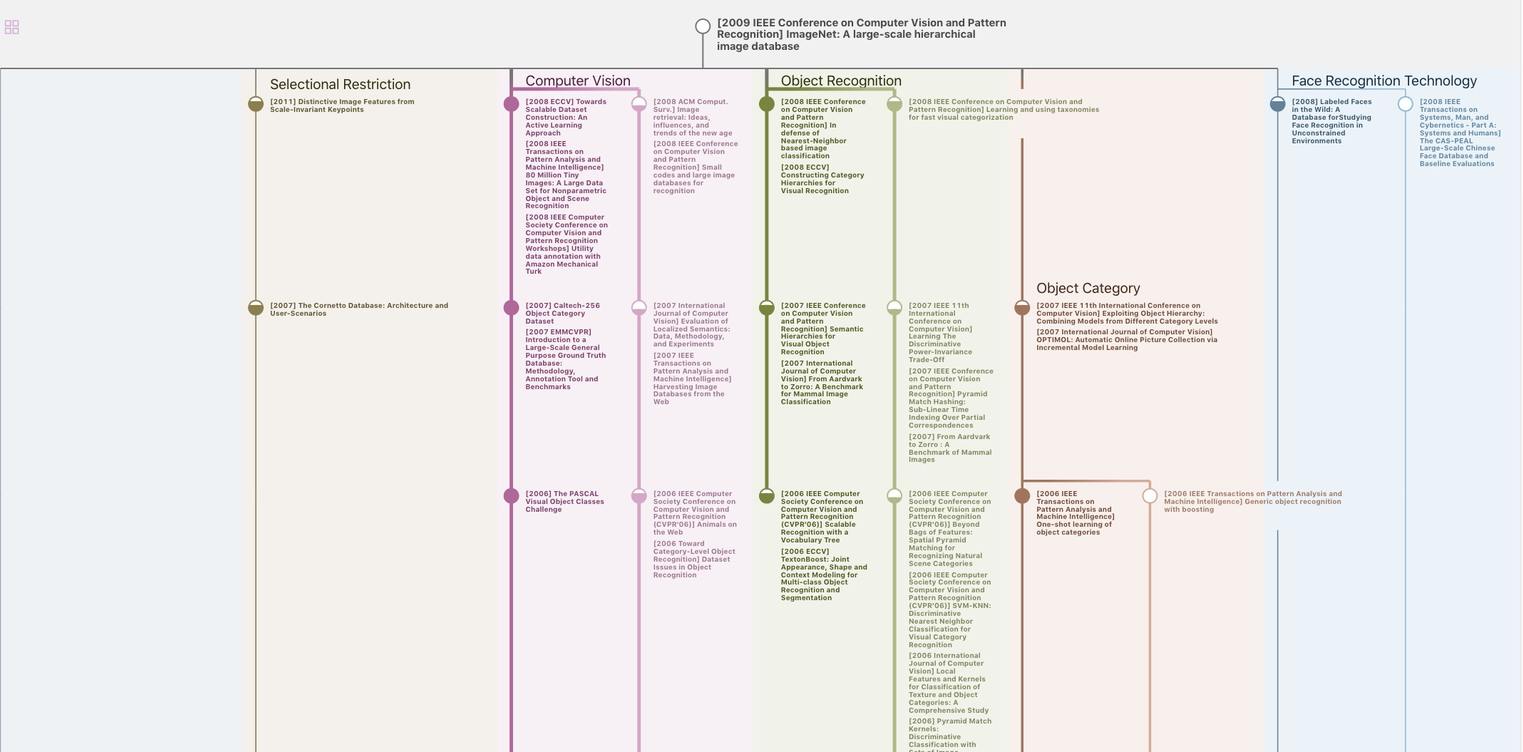
生成溯源树,研究论文发展脉络
Chat Paper
正在生成论文摘要