Dense motion segmentation for first-person activity recognition
ICARCV(2014)
摘要
In this paper, we propose a dense motion segmentation method for human daily activity recognition from a wearable device - "Smart Glasses". The glasses are embedded with a camera, which allows the system to automatically recognise the wearer's activities from a first-person perspective. This application can be broadly applied to patients, elderly, safety workers, e-health monitoring, or anyone requiring cognitive assistance or guidance on their activities of daily living (ADLs). We validate our system in challenging real-world scenarios, and compare two feature extraction approaches: averaged optical flow and a combined dense motion segmentation approach. We classify them using LogitBoost (on Decision Stumps) and Support Vector Machine (SVM). We also suggest the optimal settings of the classifiers through cross-validation over our ADLs database. The results show that the optical flow with average pooling has a good performance when classifying general locomotive activities. The results also indicate the benefits that dense motion segmentation features can have on reliably classify activities involving a moving object, such as hands. We achieve an overall accuracy of up to 69.76% on 12 ADLs using local classifiers, and with a Hidden Markov Model (HMM) process this accuracy improves to up to 89.59%.
更多查看译文
关键词
human daily activity recognition,first-person activity recognition,smart glasses,local classifiers,feature extraction approach,hidden markov model process,visual databases,camera,image segmentation,averaged optical flow,adl database,svm,cognitive assistance,logitboost,decision stumps,general locomotive activities classification,feature extraction,image classification,support vector machine,image sequences,cameras,object recognition,classifiers,disabled people,elderly people,hidden markov models,handicapped aids,wearable device,dense motion segmentation method,support vector machines,activities of daily living,image motion analysis,computer vision,optical imaging,accuracy
AI 理解论文
溯源树
样例
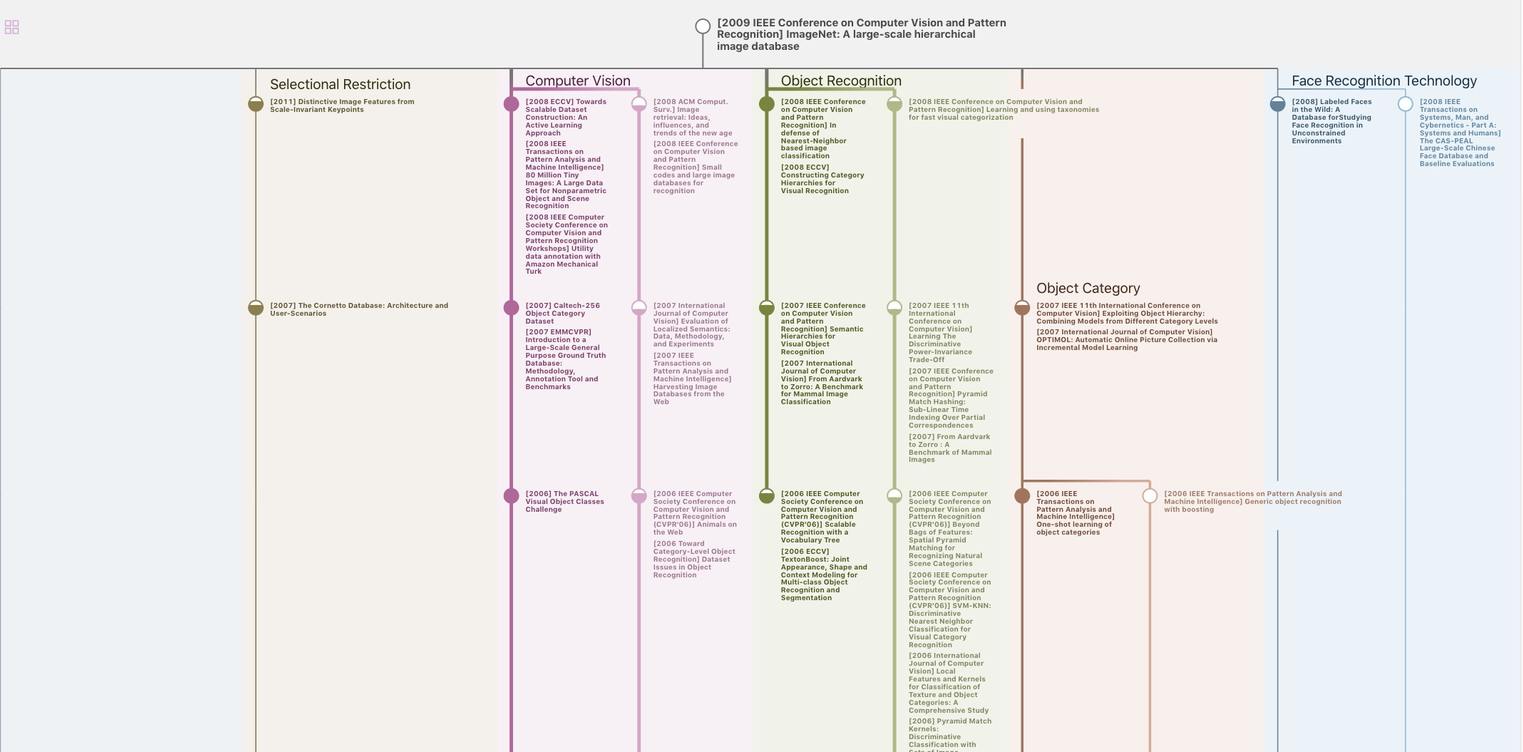
生成溯源树,研究论文发展脉络
Chat Paper
正在生成论文摘要