Optimal Estimation With Arbitrary Error Metrics In Compressed Sensing
2012 IEEE STATISTICAL SIGNAL PROCESSING WORKSHOP (SSP)(2012)
摘要
Noisy compressed sensing deals with the estimation of a system input from its noise-corrupted linear measurements. The performance of the estimation is usually quantified by some standard error metric such as squared error or support error. In this paper, we consider a noisy compressed sensing problem with any arbitrary error metric. We propose a simple, fast, and general algorithm that estimates the original signal by minimizing an arbitrary error metric defined by the user. We verify that, owing to the decoupling principle, our algorithm is optimal, and we describe a general method to compute the fundamental information-theoretic performance limit for any well-defined error metric. We provide an example where the metric is absolute error and give the theoretical performance limit for it. The experimental results show that our algorithm outperforms methods such as relaxed belief propagation, and reaches the suggested theoretical limit for our example error metric.
更多查看译文
关键词
Belief propagation,compressed sensing,error metric,estimation theory
AI 理解论文
溯源树
样例
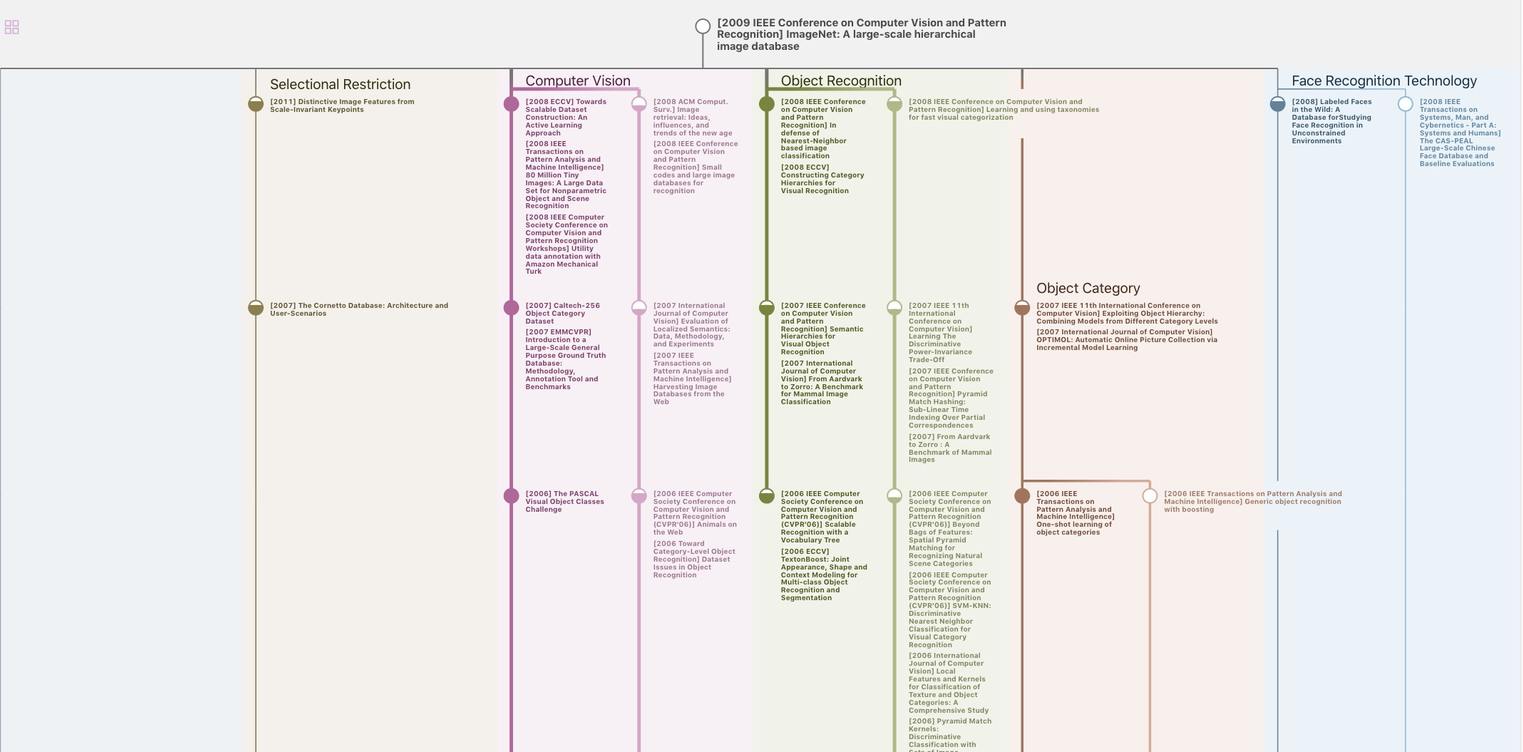
生成溯源树,研究论文发展脉络
Chat Paper
正在生成论文摘要