Psychological stress detection from cross-media microblog data using Deep Sparse Neural Network
ICME(2014)
摘要
Long-term stress may lead to many severe physical and mental problems. Traditional psychological stress detection usually relies on the active individual participation, which makes the detection labor-consuming, time-costing and hysteretic. With the rapid development of social networks, people become more and more willing to share moods via microblog platforms. In this paper, we propose an automatic stress detection method from cross-media microblog data. We construct a three-level framework to formulate the problem. We first obtain a set of low-level features from the tweets. Then we define and extract middle-level representations based on psychological and art theories: linguistic attributes from tweets' texts, visual attributes from tweets' images, and social attributes from tweets' comments, retweets and favorites. Finally, a Deep Sparse Neural Network is designed to learn the stress categories incorporating the cross-media attributes. Experiment results show that the proposed method is effective and efficient on detecting psychological stress from microblog data.
更多查看译文
关键词
tweet images,cross-media microblog data,social networks,cross-media,tweet text,retweets,psychology,physical problem,visual attributes,stress detection,psychological stress detection,deep learning,tweet comments,microblog,linguistic attributes,mental problem,automatic stress detection method,cross-media attributes,low-level features,social networking (online),social attributes,neural nets,deep sparse neural network,long-term stress,pragmatics,brightness,stress,feature extraction
AI 理解论文
溯源树
样例
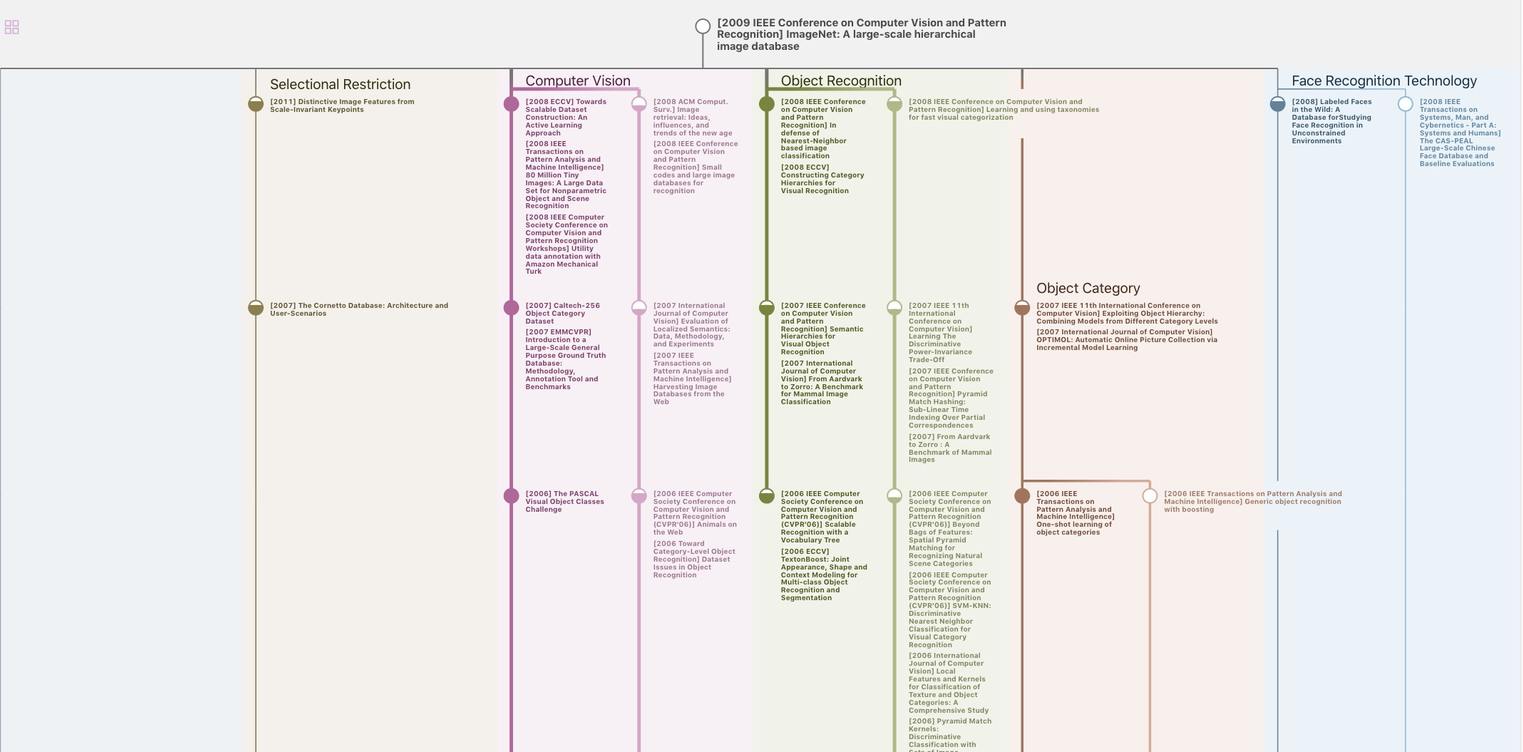
生成溯源树,研究论文发展脉络
Chat Paper
正在生成论文摘要