ACCD: Associative Classification over Concept-Drifting Data Streams.
Lecture Notes in Artificial Intelligence(2014)
摘要
Associative classification has shown good result over many classification techniques on static datasets. However, little work has been done on associative classification over data streams. Different from data in traditional static databases, data streams typically arrive continuously and unboundedly with occasionally changing data distribution known as concept drift. In this paper, we propose a new Associative Classification over Concept-Drifting data streams, ACCD. ACCD is able to accurately detect concept drift in data streams and reduce its effect by using an ensemble of classifiers. A mechanism for statistical accuracy bounds estimation is used for supporting concept-drift detection. With this mechanism, accuracy recovering time is decreased and a situation where ensemble of classifiers drops accuracy is avoided. Compared to AC-DS (first technique on associative classification algorithm over data streams), AUEH (Accuracy updated ensemble with Hoeffding tree) and VFDT(Very Fast Decision Trees) on 4 real-world data stream datasets, ACCD exhibits the best performance in terms of accuracy.
更多查看译文
关键词
concept drift,changing environments,data streams classification,associative classification,multiple class-sssociation rules
AI 理解论文
溯源树
样例
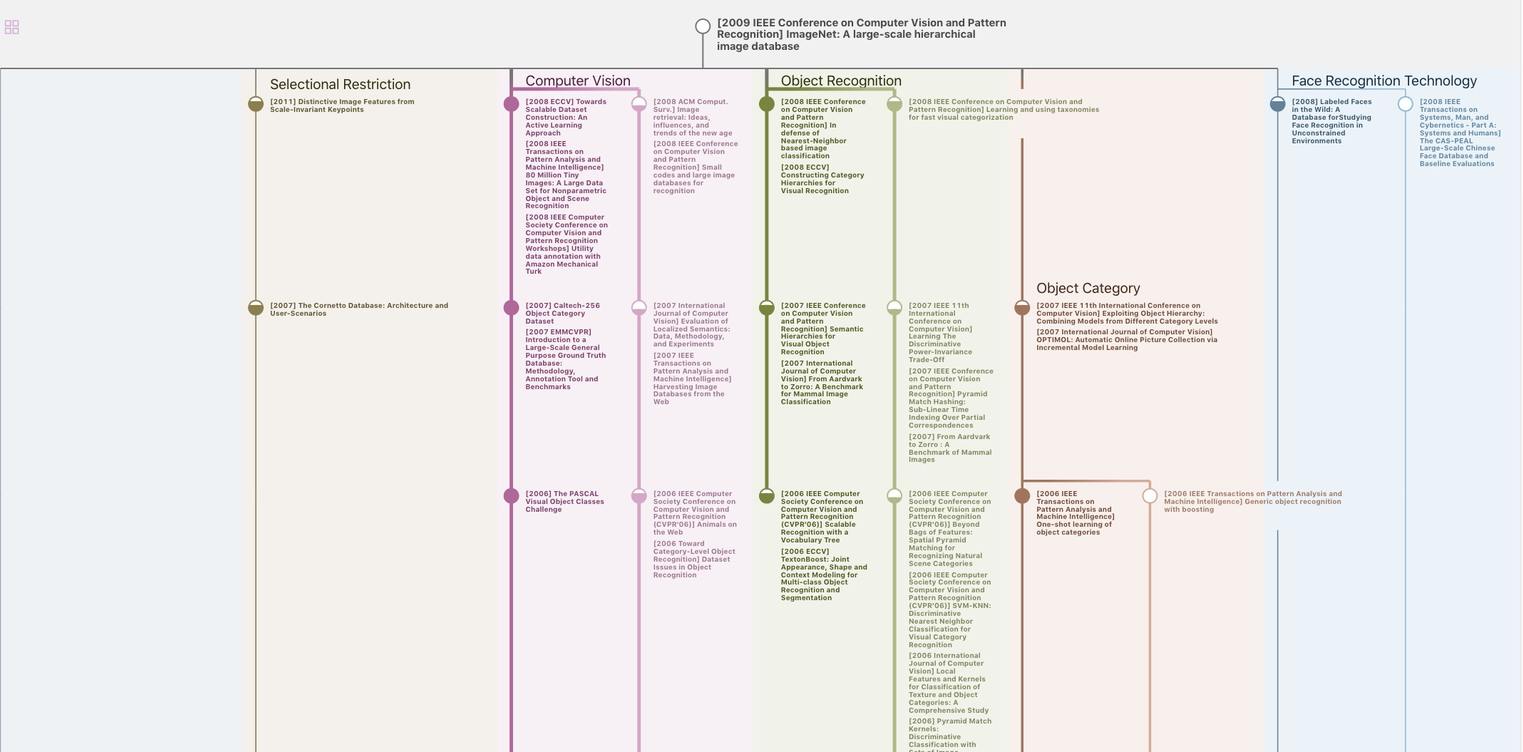
生成溯源树,研究论文发展脉络
Chat Paper
正在生成论文摘要