Locality Preserving Discriminative Dictionary Learning
Image Processing(2014)
摘要
In this paper, a novel discriminative dictionary learning approach is proposed that attempts to preserve the local structure of the data while encouraging discriminability. The reconstruction error and sparsity inducing l(1)-penalty of dictionary learning are minimized alongside a locality preserving and discriminative term. In this setting, each data point is represented by a sparse linear combination of dictionary atoms with the goal that its k-nearest same-label neighbors are preserved. Since the class of a new data point is unknown, its sparse representation is found once for each class. The class that produces the lowest error is associated with that point. Experimental results on five common classification datasets, show that this method outperforms state-of-the-art classifiers, especially when the training data is limited.
更多查看译文
关键词
Classification,supervised learning,discriminative dictionary learning,locality preserving
AI 理解论文
溯源树
样例
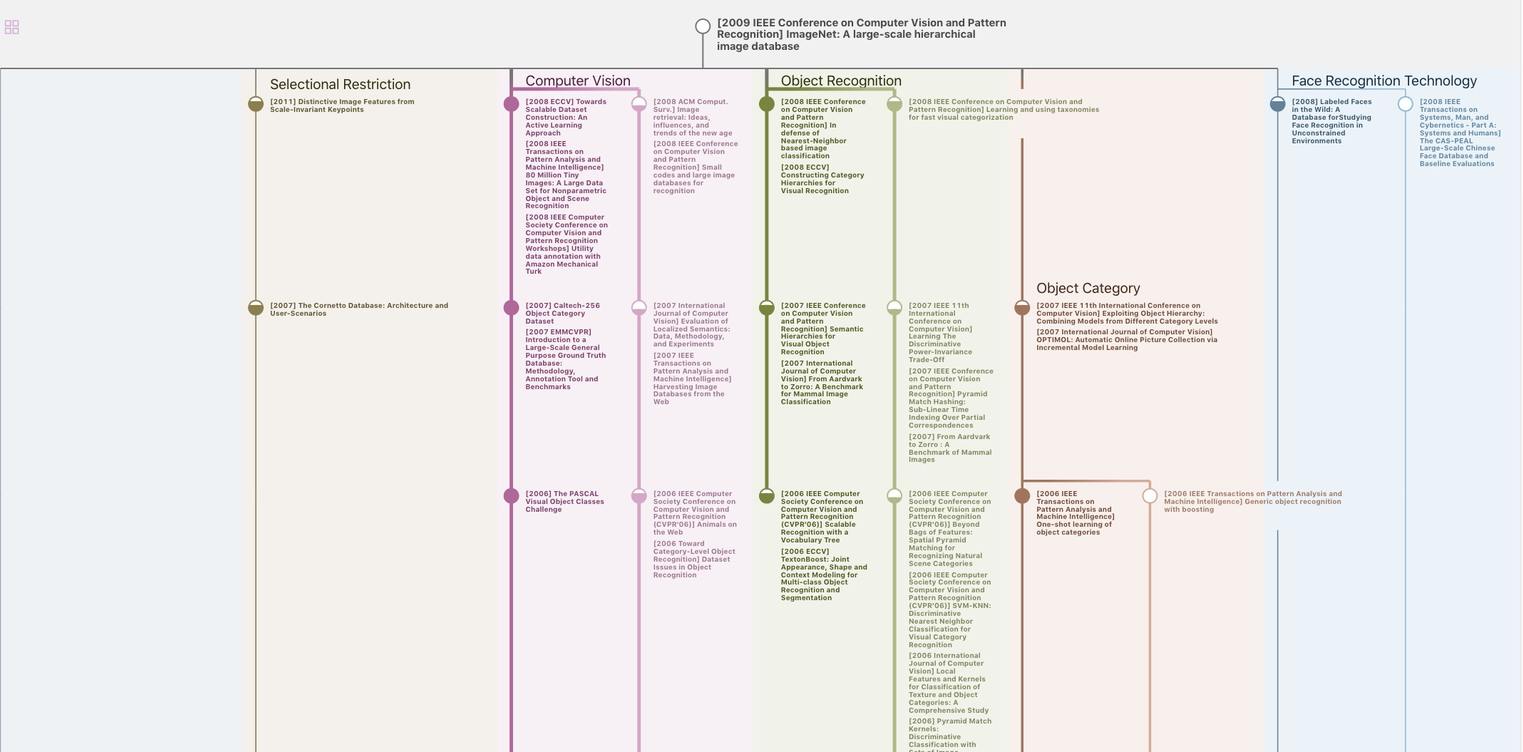
生成溯源树,研究论文发展脉络
Chat Paper
正在生成论文摘要