MapReduce for Large-Scale Monitor Data Analyses
TrustCom(2014)
摘要
The recent years witness the rapid development of the Internet of Things (IoT). Increasing numbers of conventional manufacturing enterprises face the challenge of using current data management systems to collect and analyze the massive volume of monitor data generated by sensors and equipments to improve the design, manufacture and maintenance of products. Hence, Map Reduce technique has gained a lot of attention for its applicability in large parallel monitor data analyses. In this paper, we apply a series of metrics implemented based on Map Reduce framework to depict a large collection of monitor data. With the help of these proposed metrics, domain experts can quickly analyze monitor data and feedback the analysis results to improve the design, manufacture and maintenance of products. Furthermore, we conduct a series of experiments on the real-world data sets and experimental results show that when facing a large amount of monitor data, these proposed Map Reduce implemented metrics outperform the conventional structured databases implemented metrics. Moreover, these Map Reduce implemented metrics have been successfully applied to a construction machinery manufacturer's condition monitoring system.
更多查看译文
关键词
database management systems,data management systems,product design,parallel processing,construction machinery manufacturer condition monitoring system,large-scale monitor data analysis,monitor data,mapreduce,iot,machinery production industries,mapreduce, monitor data, metric,data analysis,product manufacturing,internet of things,construction equipment,metric,condition monitoring,maintenance engineering,product maintenance,mechanical engineering computing,structured databases,manufacturing enterprises,parallel monitor data analysis,temperature measurement,distributed databases,time series analysis
AI 理解论文
溯源树
样例
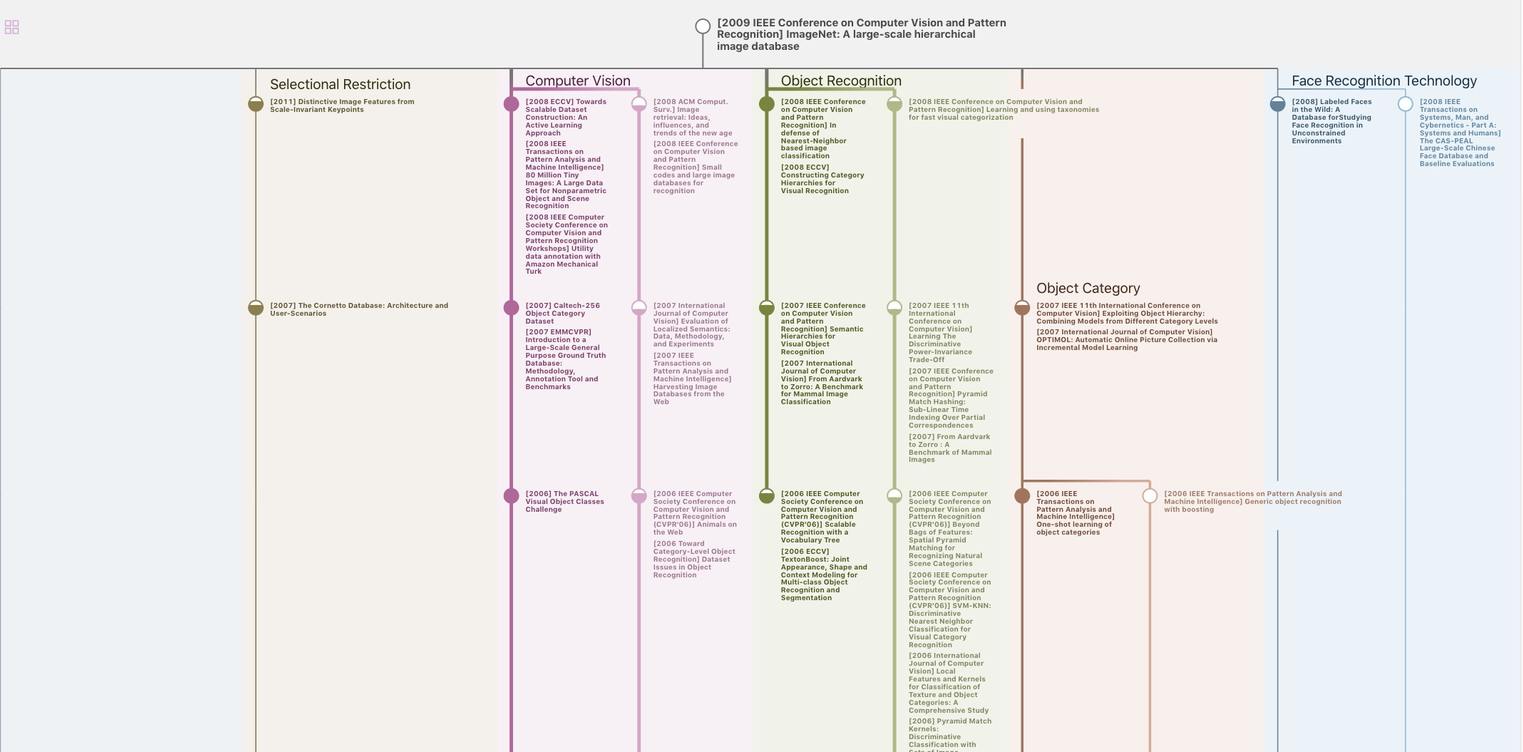
生成溯源树,研究论文发展脉络
Chat Paper
正在生成论文摘要